An AI-machine learning data challenge: Predicting the unpredictable
A difficult test for companies wanting to capitalize on huge amounts of data and emerging tech is getting it to fit what they can't see, says MIT's Iyad Rahwan.
AI and machine learning hold enormous promise, scholars at the MIT Sloan CIO Symposium stressed, with advances in healthcare and energy conservation, among others, attributed to the prodigious amounts of data the technologies rely on. But as behemoths like Google and others with the R&D dollars continue to push ahead, CIOs in many companies struggle to manage AI-machine learning data. What challenges should they expect to face?
The senior-executive-level audience gathered in Cambridge, Mass., in May put the question to MIT researchers in a panel discussion about the future of work. It was relayed by Erik Brynjolfsson, director of the MIT Initiative on the Digital Economy.
A big challenge, said panelist Iyad Rahwan, associate professor of media arts and sciences at MIT Media Lab, is making sure the AI-machine learning data is current and is directed toward a specific purpose, such as predicting the demand for a product. Sometimes companies "go wild" and use it for a variety of things.
"Then things change, and, sometimes when they change, the distribution of the things that are happening in the world shift because of some change in regulations or something else," Rahwan said. The result could at first be indirect, but later, "you could be losing out on further opportunities to optimize the business."
Brynjolfsson quizzed Rahwan about the AI-machine learning data challenge, some real-world ways it can play out and also about the biases humans may be building into algorithms and technology that make increasingly important decisions about people's lives. Their conversation, edited for clarity and brevity, follows.
Erik Brynjolfsson: Regarding the vast data that organizations have, what challenges exist leveraging that data in a world of AI and machine learning?
Iyad Rahwan: I think one of the challenges is to know that the data is up to date and actually reflects some underlying process. For example, if you're trying to predict the movement of a stock price, or you're trying to predict the demand for Uber in different locations, I think these are a bit more structured. But sometimes you build a predictive model from data, and then you go wild and you use it to optimize all sorts of business processes.
But then things change, and, sometimes when they change, the distribution of the things that are happening in the world shift because of some change in regulations or something else.
Brynjolfsson: Give me some specific examples.
Rahwan: You could, let's say, optimize something to do with transportation or with logistics, and then, all of a sudden, some change in regulation takes place. And this has an impact on your business that is very indirect. So, all of a sudden, maybe there are fewer migrants moving into the country or into the place, which means then that they would demand fewer removalists [movers].
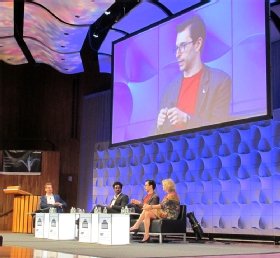
And there's kind of a trickle effect. If you train machine learning models on one set of data that is historical and then deploy it, and the world changes because of something you haven't really thought would impact your business, then you could be losing out on further opportunities to optimize the business.
So, in this case, a lot of the new kinds of techniques in AI have this online learning -- so, algorithms that basically continuously learn. It's not like you train them, and then once they're trained, you deploy them. You have to continuously learn from their experiences, from the real world nonstop, essentially.
Brynjolfsson: Are there concerns about them learning the wrong things? There's been discussion of the biases that we humans have when we make hiring decisions, parole decisions, recommendations, loan decisions -- and will those biases be learned by our machines?
Rahwan: Essentially, humans are biased already, and some of these biases are good, because we need to discriminate, for example, between something that is good quality and lower quality. That's a useful bias. But there are also bad types of bias -- a bias that is discriminatory or breaks some laws or norms in some way -- for instance, discriminating in hiring decisions against a minority group.
I think AI is now making these biases a bit more salient and a bit more identifiable, because now we have a better understanding of how the data causes the bias. And I think that's already creating pressure on companies to be a bit more thoughtful -- and if they're not, then that's a real public relations, reputational risk for companies.