5-step predictive analytics process cycle
A viable predictive model that yields valuable outcomes requires a methodical team approach to goal-setting, data integrity and model development, deployment and validation.
There are many ways to conceptualize the predictive analytics process cycle. Execution will vary according to organization, domain and industry. In many cases, the overall process lifecycle may be embedded across one or more applications, services or algorithms.
In the most straightforward cases, the only connection to the predictive process is providing feedback to a service maintained by someone else. For example, predictive analytics is baked into fraud engines and spam filters. Marking an email as spam or a transaction as fraud provides feedback to a predictive process that someone else maintains.
At the other extreme, a more mature predictive analytics process includes three integrated cycles around data acquisition, data science and model deployment that feed into each other. Gartner's MLOps framework, for example, includes complementary processes around development, model release and model deployment that overlap and work together.
What are the applications of predictive analytics?
"Predictive analytics has broad use cases across healthcare, retail, sales and marketing, and a plethora of other sectors and industries," said Elif Tutuk, vice president of innovation and design at SaaS software provider Qlik. Predictive analysis can help forecast inventory levels, make customer recommendations, prioritize leads and improve healthcare.
"One of the primary ways in which these [predictive] models can be used practically is by analyzing customer shopping behavior," reported Bill Szybillo, business intelligence manager at ERP software provider VAI. "In an age of rapid demand and supply chain shortages, this is critical for businesses hoping to keep shelves stocked and customers in the aisles or customers on a website. However, it's not easy to just develop these forecasts at the snap of a finger, as this often requires ongoing data collection for months and often years in some instances."
Predictive analytics is "a universal technology," Tutuk added, "but the challenge comes from the tools and the data itself." That's why understanding the process cycle is helpful. If the wrong tools are used or if inaccurate or outdated data is included, the predictive outcomes will be negatively impacted.
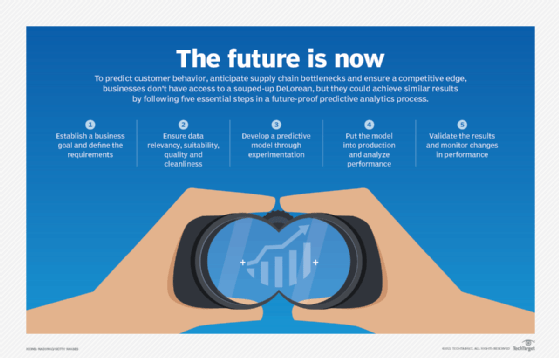
Businesses must practice due diligence when selecting data partners and ensuring their data is accurate and not siloed or otherwise limited in any way. Various data services make it easier to start with vetted external data that can shine a light on other factors leading to rapidly changing trends.
Many enterprises are combining internal data records with external sources to glean insights. "[A] major trend in predictive analytics," Szybillo said, "is the ability to help manufacturers determine future inventory levels not only through past usage, but also using external data sources with their internal analytics data, such as weather patterns, changes in demand, insights into the supply chain and more."
What are the steps in the predictive analytics process?
Five key phases in the predictive analytics process cycle require various types of expertise: Define the requirements, explore the data, develop the model, deploy the model and validate the results. Although each of these steps may be driven by one particular expertise, each step of the process should be considered a team effort. Statisticians, for instance, can help business users make informed decisions. Data scientists can help business analysts select better data sets. Data engineers can work with data scientists to create models that are easier to deploy.
Although various business applications, analytics toolkits and cloud services may automate many of these processes, understanding the entire process can help locate process bottlenecks and improve accuracy. Following is a detailed view of the predictive analytics process cycle and the experts influencing each step.
1. Define the requirements
Business user or subject matter expert
Predictive analytics typically begins with a business expert focused on solving a problem like reducing fraud, maintaining inventory, improving customer recommendations or increasing the value of a loan portfolio. Start the process by generating a list of questions and prioritizing each question according to importance, suggested Philip Cooper, vice president of AI and analytics products at Salesforce Tableau CRM. Focus could be on clarifying a specific goal using measurable outcomes. Business users may collaborate with a statistician to verify the result and establish metrics for measuring success. It's also important to place the goal in a broader context. A fraud engine, for example, may need to balance precision against timeliness or at least return results within a limited time period.
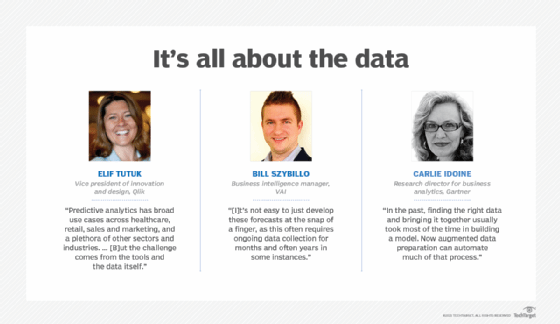
2. Explore the data
Statistician or data analyst
Identify the data that may be relevant to the goal's requirements. A data analyst should determine what data sets are available and how they might be used to improve the predictions and address other business objectives. The data's relevancy, suitability, quality and cleanliness must be considered. Knowing how and why the data is collected can help identify any problems in advance of feeding the information into the predictive analytics model.
3. Develop the model
Data scientist
Consider how different predictive models might yield the most valuable outcome, then work backward to determine the best way of organizing the raw data into the appropriate set of features for the model. Experiment with different features, algorithms and processes that strike the right balance between performance, accuracy and other requirements like explainability. The data scientist may use a data wrangling tool to transform raw data sets into new features for calculating a prediction. Grouping temperature levels into cold, warm and hot ranges, for example, may lead to a more efficient predictor of ice cream sales than raw numeric temperatures.
4. Deploy the model
Data engineer
The newly developed predictive model needs to be put into production so it can deliver results. A unique predictive analytics insight is only valuable if it makes a meaningful difference in the ongoing process. A new fraud engine, for example, may need to be integrated into the transaction approval process, or a new lead scoring algorithm may help support the sales team's workflow. Once the data scientist approves the model, the data engineer determines how to optimize the process of retrieving, cleaning and transforming the raw data required for the model at scale. The data scientist and engineer then need to connect the result with the appropriate application or workflow.
5. Validate the results
Business user and data scientist
If the data science was well executed, the predictive analytics model should meet the performance, accuracy and other requirements when it's deployed with live data. But performance can change over time. In some cases, changes in customer sentiment, business climate or other factors can affect model performance. In other cases, malicious actors may attempt to subvert model accuracy deliberately. Email spammers, for example, can develop better AI tools for simulating legitimate messages, while cybercriminals may use tactics to evade fraudulent detection. Regardless of the cause, it's helpful to monitor changes in model performance and set specific performance thresholds for updating models. A fraud detection model that degrades by more than 1%, for instance, may require updating, while the threshold for a product recommendation engine may be 5%.
Augmenting the predictive process
The predictive analytics process cycle has traditionally been complicated, time-consuming and arduous. Better tools, management processes and cloud services are helping to improve the process.
"Many steps in this process are now automated or augmented," said Carlie Idoine, research director for business analytics at Gartner. New tools can augment data preparation, model building and deployment. XOps characterizes the various capabilities involved in the predictive analytics process cycle, including DataOps, ModelOps, AIOps, MLOps and Platform Ops.
"In the past," Idoine explained, "finding the right data and bringing it together usually took most of the time in building a model. Now augmented data preparation can automate much of that process."
Augmented model building tools can help data scientists determine which features or combinations of variables lead to the best predictive outcomes. Augmented model deployment tools allow data scientists to push models onto an infrastructure created by data engineering teams.
Although these augmented and automated tools make it easier for business users to drive most of the process, Idoine cautioned against eliminating the need for experts to oversee the process. "Automation," she said, "does not take experts out of the loop but makes the process more efficient, and this allows different types of users to use the tools to take advantage of predictive analytics."