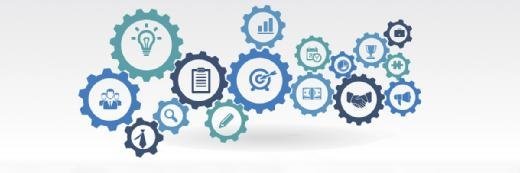
Fotolia
10 features to look for in visualization tools for big data
Big data is meaningless if it isn't understandable. Experts explain why users need data visualization tools that offer embeddability, actionability and more.
Visualization tools for big data show promise in unlocking the value of data collected by enterprises. Getting the best results requires building out an infrastructure for aggregating data from across the enterprise and simplifying the process of discovering and sharing insights.
Why? Because data is only as valuable as someone's ability to understand it. Humans are primarily visual creatures who understand relationships and trends in data swiftly and intuitively through images, charts and graphs.
"In the big data era, good visualization helps cut through the noise in your data to pick out significant values or patterns quickly and accurately," said Zachary Jarvinen, senior analytics product marketing manager at OpenText, an enterprise information management software company.
The following are a variety of features and capabilities that experts recommend organizations consider when adopting visualization tools for big data:
1. Embeddability: Big data is used to drive real business insights. Those insights then need to be embedded into operational business systems to properly guide users as to what has happened, why it happened, what will happen and the steps they can take to alter that outcome, said David Marko, managing director of on-demand analytics solutions and information management at Acumen Solutions Inc., an IT consultancy.
Visualizations can provide more value to business users when embedded via dashboards into the interfaces or tools that end users love and live in. This includes portals and applications already in use, because visual analytics don't require users to acquire a new skill set. Good APIs are important to help extend visualizations into other applications, said Saurabh Abhyankar, senior vice president of product management at MicroStrategy Inc.
2. Actionability: Tools for visualization must deliver practical value via useful predictions and prescriptions. "A visualization on its own delivers insight, but when coupled with transactions or write-back features makes it immediately actionable, and these instruments elevate the end user's role by making them more responsible," Abhyankar said. Features that support actionability include support for trend lines, one-click metrics and guided advanced analytics workflows.
3. Performance: If visualization tools for big data distract workers from the flow of their work, they're less likely to be used. A couple seconds' delay may not be significant for some use cases but may discourage users tasked with evaluating hundreds of decisions throughout the day. Features that help improve performance include prompts, data optimization settings and dynamic loading options.
Another performance-related feature to consider is the tool's ability to run computations on GPUs. GPUs were initially focused on better graphics. New math libraries from GPU-makers like Nvidia can also help accelerate complex data analysis. As data sets have grown, rendering large amounts of data with traditional architectures has become harder, said Nima Negahban, CTO and co-founder at Kinetica, a distributed database company. GPUs used with direct memory access can help crunch large volumes of data faster and more efficiently. This makes it easier to build high-definition visualizations on the server side that simply get served by the application via a web application.
4. Dynamic infrastructure: Serverless computing is an emerging paradigm for provisioning applications as code that is fully managed by cloud services. Developers can focus on code logic, while leaving the heavy lifting to a cloud provider that can access enterprise big data through preconfigured integrations. "Enterprise customers save time, headaches and money by not having to manage their own big data infrastructure for their BI workloads," said Nick Mihailovski, product manager for Data Studio at Google Cloud. Serverless tools also increase agility for big data ad hoc analytics projects that might normally strain BI infrastructure.
5. Interactive exploration: Data exploration features simplify the ability for employees analyzing big data sets to quickly spin up a space to visualize their data and validate their hypotheses. Ideally, this exploratory environment should be easy to use, easy to access and fit seamlessly into an analyst's BI workflow. Ad hoc analysis features require support for different types of visualizations and interactive analysis. This includes capabilities for filtering, slicing and dicing, and drilling up and down at speeds that make it possible for users to delve into huge volumes of data and get answers to their questions immediately, said Pratik Jain, technical analyst at Kyvos Insights, a BI software provider.
6. Collaboration: Real-time collaboration capabilities in visualization tools for big data allow employees to have more meaningful conversations about their discoveries. This includes the ability for employees to collaborate in real time on current data, rather than requiring them to send static files and screenshots to one another.
7. Streaming data support: Enterprises are now faced with wrangling massive volumes of complex, streaming data from a variety of different sources. Many visualization tools use legacy back ends based on structured batch data analysis. This makes it difficult to analyze extreme data in real time. Support for streaming data can allow more visualization use cases involving data from social media, internet of things devices and mobile applications.
8. AI integration: Visualization tools for big data are starting to experiment with machine learning, deep learning and natural language processing to make it easier to analyze, explore, predict and prescribe actions. Acumen's Marko said, "Some players are trying to create partnerships with a slew of companies to do this, but those are typically clunky integrations that slow down the process and confuse the users. The tools that can find ways to offer these under one skin are the ones that will separate themselves in the coming years."
9. Integrated metadata management: metadata management complements an enterprise visualization tool to improve both usability of data and the accuracy of the attendant analysis. Data lakes, by design, are geared toward reducing the time-to-value of data or, in other words, shortening and simplifying the process of data ingestion.
Nitin Bajaj, director of business intelligence and analytics at NTT Data Services, said, "While this paradigm reduces the effort involved in ingesting new data sets into the data lake, it also has the potential to exacerbate misuse of data by citizen users who may not completely understand the provenance of the data they wish to consume."
10. Self-service capabilities: Self-service capabilities in visualization tools for big data allow for rapid prototyping and development that accelerates hypothesis testing. Traditional BI and reporting tools are developer-oriented, with complicated functionality that slows the pace of analysis in the enterprise, Bajaj said.