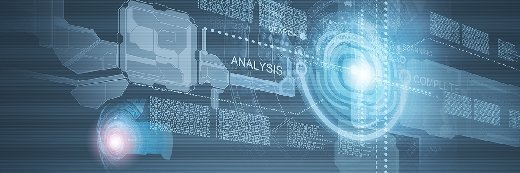
Sergey Nivens - Fotolia
The 5-step process for finding data science leader unicorns
Companies continue to struggle to effectively hire for their data science teams, but Gaurav Anand says that a hybrid solution that considers tech and business knowledge might work.
Are today's data science programs consistently telling decision-makers a useful story? Are those departments regularly solving the right problems? When those two questions are not routinely asked, data scientist teams often experience low morale resulting in high turnover. Besides the raw hurt of experiencing high personnel exodus, a company's leadership that has incurred huge investments of time and resources only to draw a very wrong conclusion or to take no action on vital matters can be devastating. Every decision, even small ones, can impact market share, which rests on creditability, innovation, response time, logistics, design sensitivity and more. Both the public and private sectors need data scientists to corral and query big data in inventive, provocative ways to achieve a competitive edge. But something has gone awry. Data science silos have sprung up across the datascape. What's the answer?
Organizations seeking the best resources for managing data science programs may need to rethink their tech leader hiring strategies. The industry is young, but a quick look at its short history and a review of the current challenges suggest a useful "unicorn" solution to overcoming the silo problem.
The history of data science and the data scientist position
Data scientists of today were the statisticians and mathematicians of the 1980s. The data scientist as an established role took off around the early 2010s with the rise of the internet economy. This role helps firms with recommender systems, fraud detection and churn prediction, for example. The nice-to-have data scientist became a must-have almost overnight in 2012, yet it still applies.
The data scientist's work depends on input from the less glamorous data engineer (who builds the database) and data analyst (also called a business analyst), who gathers insights from the data to make basic assumptions that the data scientist develops. Some data scientists build models for better human decision-making. Others create models for machines to achieve highly targeted outcomes. The third type of data scientist relates to research-based AI and machine learning.
Challenges with data science program leadership
Hiring is a big part of the problem. Most organizations look for a Ph.D. in a related field or with significant experience with machine learning models, and they often drop the management experience requirement to obtain technical talent. This strategy results in hiring a data scientist who does not have the three Cs -- culture, context and communication skill sets -- to manage effectively.
This flawed ethos is cultivated because business leaders want quick answers to stay one step ahead of their competitors. Through no fault of their own, the data scientists respond with solutions that solve no problems or didn't answer the right question. The cause stems from data science silos that often do not integrate with the overall business.
Several organizational charts such as these organizational/reporting permutations are worth experimentation (everyone must continue to iterate) to see which might fit the company's needs and cultures. However, all the organization charts on the earth will not provide better data science output if the data science leaders' profiles are not correct. The key to finding effective data science leaders is recruiting for both tech hard skills and business-savvy soft skills. This is best achieved through a hybrid approach to hiring.
The hybrid (unicorn) solution to hiring effective data science leaders
A data scientist is not the best person to manage a team of data scientists, which is part of the reason data science programs in many companies are not working as hoped. Percentages vary, yet as few as 10% of all data science programs make it into production. The hybrid/unicorn model involves a mix of technical prowess and business acumen, and a smattering of both senior and junior data scientists. The model includes:
- Hiring from within.
- Not requiring the technical depth, but rather a basic understanding of data science benefits.
- Placing someone who understands what algorithms have to offer.
- Recruiting a person who can visualize and communicate solutions to real business challenges.
- Ensuring effective mentorship for junior data scientists by including coach/mentor/leader senior data scientists on the team.
It is difficult to find one person who has both the hard technical skills of an experienced, senior data scientist and the soft transferrable skills of a seasoned business manager with excellent people skills. The hybrid unicorn team model offers the following advantages:
- Data science silos are gone.
- The myth of a pure data scientist as good data science manager is busted.
- Generalist managers better understands the people's goals and strengths on their teams and assign projects appropriately.
- The data science teams develop profound, new, useful business insights and capabilities.
- They will have fun.
Pros and cons of data science leaders: Hybrid/unicorn model uses best of both
Pros | Cons | |
Technical profile |
|
|
Business profile |
|
|
Pros, cons and expected outcomes of this approach
Corporations are still growing into the data science field and are struggling to optimize resources that will synthesize breathtaking insights from mountains of big data. The justifiable tendency is to over-index on hiring technical skills when looking for technical (data science) managers. In the beginning, it made sense. However, the turnover rate in these positions shows that firms are not making the best use of their data scientists. A reasonable yet gutsier course is to challenge the old and experiment with something new. As Peter Drucker once said, "If you want something new, you have to stop doing something old." Now is the time to break things up and re-imagine the data science manager's profile. The technical (data science) manager has benefits, as does the all-business profile. The hybrid unicorn solution is not a silver bullet, but the idea is to take the best of pros and reduce the cons seen in the accompanying table.
The potential that lies ahead
At the beginning of the big data era, data scientists were practically nonexistent. Today, universities are turning out data engineers, data analysts and data scientists aplenty. These very bright individuals can build algorithms to plug into workflows that move KPIs in the desired direction. The potential for using these resources is exhilarating. If they are properly managed, their work gets delivered to the right people to solve relevant problems, and the organization jubilantly uses the knowledge to edge out their competition in a timely fashion instead of two weeks too late.
Numbers are comfortable, and top decision-makers want to know easy answers to questions like "How much?" or "What's the percentage gain?" Data scientists are just the quantitative analyst to answer their questions. However, hiring "people" people -- who also have data science knowledge -- to manage the data science function will yield a better outcome in the heady and exciting data science discipline. As conservative companies suffer the known, roll-the-dice organizations push the envelope of the lucky unknown. Result: The delightfully nerdy data scientists working in an iterative, imaginative, fail-fast way will produce stimulating, needle-moving results.
About the Author:
Gaurav Anand is head of finance at Google Cloud. He has a proven track record in business strategy and analytics, and enterprise operations and team building. He has an MBA from the Stephen M. Ross School of Business, University of Michigan, and a Bachelor of Technology (B.Tech.), Chemical Engineering from the Indian Institute of Technology, Kharagpur. Anand can be reached at [email protected].