Analytical modeling is both science and art
Models are the key to predicting outcomes to business decisions. And while math may make them tick, it also takes a certain eye -- and ear -- for what works.
Advanced analytics won't produce an ounce of business insight without models -- the statistical and machine learning algorithms that tease patterns and relationships from data and express them as mathematical equations. The algorithms tend to be immensely complex -- mathematicians and statisticians (think data scientists) are needed to create them and then tweak the models to better fit changing business needs and conditions.
But analytical modeling is not a wholly quantitative, left-brain endeavor. It's a science, certainly, but it's an art, too.
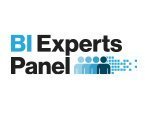
The art of modeling involves selecting the right data sets, algorithms and variables and the right techniques to format data for a particular business problem. But there's more to it than model-building mechanics. No model will do any good if the business doesn't understand its results. Communicating the results to executives so they understand what the model discovered and how it can benefit the business is critical but challenging -- it's the "last mile" in the whole analytical modeling process and often the most treacherous. Without that understanding, though, business managers might be loath to use the analytical findings to make critical business decisions.
An analytical model estimates or classifies data values by essentially drawing a line through data points. When applied to new data or records, a model can predict outcomes based on historical patterns. But not all models are transparent, and some are downright opaque. That's a problem for execs, who often don't trust models until they see something positive result from decisions based on modeling-generated insights -- for example, operating costs go down or revenues go up. For analytics to work, modelers need to build models that reflect business managers' perceptions of business realities -- and they need to make those connections clear.
For more on
analytical modeling
Not understanding products of analytical modeling is one of many advanced analysis missteps.
Canadian hospital says analytical modeling is but one aspect of BI.
They should also be realistic about the likely fruits of their scientific and artistic labors. Though some models make fresh observations about business data, most don't; they extract relationships or patterns that people already know about but might overlook or ignore otherwise.
For example, a crime model predicts that the number of criminal incidents will increase in a particular neighborhood on a particular summer night. A grizzled police sergeant might cavalierly dismiss the model's output, saying he was aware that would happen because an auto race takes place that day at the local speedway, which always spawns spillover crime in the adjacent neighborhood. "Tell me something I don't already know," he grumbles. But that doesn't mean the modeling work was for naught: In this case, the model reinforces the policeman’s implicit knowledge, bringing it to the forefront of his consciousness, so he can act on it.
Occasionally, models do uncover breakthrough insights. An example comes from the credit card industry, which uses analytical models to detect and prevent fraud. Several years ago, analysts using a combination of algorithms and data sets uncovered a new racket: Perpetrators were using automatic number generators to guess credit card numbers on e-commerce websites. The models found that nearly identical credit card numbers were spitting out a huge number of transactions and card declines, thus uncovering a new pattern. The companies quickly figured out what was going on and implemented safeguards, avoiding millions of dollars in fraudulent transactions.
Many people think that to excel at analytics their companies need only hire a bunch of statisticians who understand the nuances of sophisticated algorithms and give them high-powered tools to crunch data. But that only gets you so far. The art of analytical modeling is a skill that requires intimate knowledge of an organization's processes and data as well as the ability to communicate with business executives in business terms. Like fine furniture makers, analytics professionals who master these skills can build high-quality models with lasting value -- and reap huge rewards for their organizations in the process.
About the author:
Wayne Eckerson is principal consultant at Eckerson Group, which helps business leaders use data and technology to drive better insights and actions. His team provides information and advice on business intelligence, analytics, performance management, data governance, data warehousing and big data. Email him at [email protected].
Email us at [email protected], and follow us on Twitter: @BizAnalyticsTT.