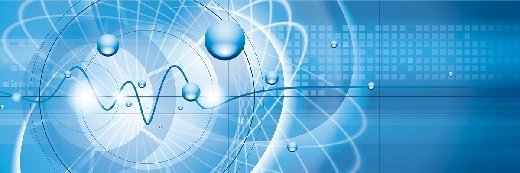
ag visuell - Fotolia
Overcoming the top 3 analytics adoption challenges
Analytics is a growing part of many businesses. Experts talk about some of the most pressing challenges enterprises may face when implementing new analytics strategies.
Analytics is key to making smart business decisions, but not every analytics adoption project succeeds. And the more significant the project, the greater the obstacles.
According to a survey of senior technology executives by Appen, a data vendor, that was released June 23, nearly 75% of executives surveyed considered AI critical to their success -- but nearly half said their company is behind on their AI journey.
Similarly, an IDC report estimates that nearly a third -- 28% -- of all AI and machine learning initiatives fail. According to IDC, one major reason that these projects fail is due to inadequate data. Another major challenge is the lack of stakeholder buy-in, experts said.
Data, data everywhere and not a drop to analyze
Steve Strohl, senior managing consultant for digital innovation services at Insight, was working with a large U.S. manufacturing firm on an analytics adoption project when he ran into a massive data problem.
"This firm must have had 50 to 60 different source systems, and each one was run like a different country," he said.
Some systems had excellent data quality, while the data quality was nonexistent in others.
"Being able to pull all that stuff together can be very challenging," Strohl said. "We had 150 different spellings for the same customer -- and they were treated as different customers in the systems. We had no way of connecting that customer to any other system in the organization."
The company wasted over a year and between a quarter to half a million dollars and eventually had to step back and do a data quality project first. What the company wound up doing was create a set of consistent business rules, Strohl said, to create a solid framework for their data, and then clean it up.
"The analytics is only going to be as good as the source data you're working from," he said.
Venkatesan Sukumaran, head of business analytics at Tata Consultancy Services, found a similar issue with a large Australian bank.
"The level of unstructured data in the enterprise had grown as the number of data sources relating to unstructured data was exploding," he said.
Some of the data was coming from outside partners as well. Addressing this increased complexity and the attendant costs required a change in mindset, said Sukumaran. Once the bank started to look at other ways to address the problem, they decided to move the data and analytics to the cloud, which affected the cost of storage, the speed and cost of processing and the time to market.
"All three dimensions achieved tangible outcomes," he said.
Another bank, based in Europe, was using analytics to determine which financial products or services to offer to which customers.
"They had many, many products, each one sitting on separate systems, not necessarily talking to each other," said Sukumaran. "So you're not optimizing at the customer level."
For example, a customer may be offered a product, but the customer did not meet the bank's risk requirements or it was priced inappropriately for that customer. The bank was able to solve the immediate data challenge with a machine learning project that created a single unified view of the customer.
But to address the bigger problem -- the lack of a coherent enterprise-wide data strategy -- the bank created a new position for a chief data officer.
"That helped bring in centralization of governance and standardization of processes," Sukumaran said. And it helped the bank create a stronger and more flexible IT environment that prepared it to deal with rapidly expanding data sets.
Are we all on the same track?
Getting senior leadership in place does a lot to help point business units in the same direction, but it only addresses part of the challenge of getting all the stakeholders on one page. One of the most critical groups of stakeholders are the end users.
At Sungard Availability Services, a disaster recovery company, one team spent three weeks out of every month developing reports for the company leadership. The company wanted to move the team to an analytics platform, specifically Qlik Sense BI.
"When we first introduced the tool, they were very skeptical and hesitant," said Shreeni Srinivasan, director of enterprise analytics and applications delivery at Sungard Availability services. "They insisted on procuring a server and database and hiring a small development team to automate the data extracts for the report."
It took several weeks to explain the features and capabilities of the analytics tool. The time it took to educate the users paid off, he said.
"Once the team bought into the tool, we were able to implement and automate the process of creating the 160-slide report," Srinivasan said. What used to take three weeks manually now only took a few hours.
"After realizing the value of the tool, this team is now the heaviest user of the tool and recommends it to other teams within our organization," he said.
A similar educational effort converted the company's HR team to analytics. Previously, the team spent several hours a month creating spreadsheets of employee data and distributing them to managers via secure email.
It took effort to convince the teams to try self-service analytics dashboards and to prove that they were secure and well-governed. But now, he said, managers can use the self-serve business intelligence tool to instantly get up-to-date information whenever they need it.
End user education is the biggest challenge in analytics adoption, said Daniel Elman, an analyst at Nucleus Research.
"Most business users aren't trained in advanced stats and math," he said. "This first step needs to be establishing user trust in the data and trust in the results."
Companies should also invest in creating a data culture, Elman said, where decisions are based on data and department leaders create data-based key performance indicators.
Lack of clear goals
Even with the right data in hand and all the stakeholders on board, analytics projects can still go awry without clear, attainable goals.
Kathleen Featheringham, director of AI strategy and training at Booz Allen Hamilton, said one problem could be that people don't understand the technology, especially when advanced analytics, machine learning and artificial intelligence come into play.
"They don't understand the current state and the capabilities," she said.
Featheringham said, there's a tendency for people to have unrealistic expectations for the accuracy of new technology.
"We think that machines should be 99% to 100% accurate," she said. "Take driverless cars. We want them to be perfect, but do you know of any humans who are 99% accurate when driving?"
The same is true of business analytics. If users expect the analytics to be 100% accurate, and they're only 70% accurate, they might see the project as a failure.
"But if the stuff you have today is only 20% accurate, then that's a big improvement," Featheringham said.
Similarly, she said, many platforms get better the more they are used. If users are expecting the platform to work right out of the box, they may be disappointed.
"Models need to be trained," she said. "Don't have the expectation that it will work the first time. It might be 50% right now, but it will go up to 80% at some point."
Srinivasan suggests that for analytics adoption projects that require new tools and processes, it helps if the chosen project offers clear and significant benefits to users. Vague expectations or the idea that analytics will magically resolve long-standing business problems are common reasons for analytics adoption project failures, he said.
"Surprisingly," he said, "Many analytics projects begin with no clear goal in mind."