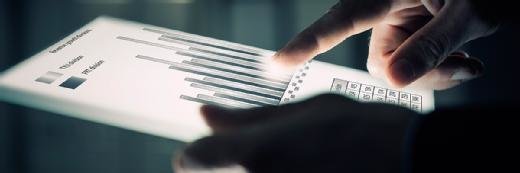
Sergey Nivens - Fotolia
Data science storytelling emerges as top analytics skill
Storytelling in data science is emerging as a critical skill to have in analytics. Without it, data-driven insights have a tendency to wither on the vine.
In the not-too-distant past, data scientists were evaluated mostly on their ability to discover, understand, curate and synthesize information. Coding skills became increasingly important, as data environments continued to grow and computing power became more accessible. Over time, nuanced needs emerged, including expertise in AI methods, judging the truthfulness of data and many other nuanced capabilities.
More recently, as many organizations have begun to realize the return from investments in advanced data capabilities, what is often lacking most is the ability to translate between what the data says and what the business needs. This ability to make sense out of a corpus of data and analytics and to convey that understanding in a way that is relevant to others not intimately involved with data and analytics is sometimes referred to as data science storytelling.
Making the pitch
Consider a typical situation: trying to make the case for an investment. Regardless of the formal process required, at the onset, there is usually a conversation -- sometimes called a pitch -- where one or more involved parties try to put forth the value proposition to commit funds and other resources. Many times, this pitch has been preceded by asking someone with access to data and analytics skills to create a supporting argument.
Armed with some understanding of what they are trying to demonstrate, data scientists may construct models with existing data to support the conclusion. They may have access to excellent tools to create visualizations, which contribute to a deliverable. The team completes the analysis and delivers its findings to the original stakeholders, as requested. All of this work is done in service to the pitch.
But even this simple scenario contains several classical data storytelling challenges.
At the outset, there was what is known as an a priori conclusion, an assumption about the conclusion that would be reached before any analysis. The data scientists were asked to reach a preestablished conclusion. The analytical exercise was done independently from the opportunity formulation, followed by involving those who were asked to create the supporting argument.
Information loss at this critical stage and the missed opportunity to ask a meaningful question can often result in starting out with a sort of cognitive bias -- bias that comes from the way in which one understands a problem or opportunity.
Armed with whatever understanding the team reached, the analysis then proceeded with existing data. There was no mention of whether the data in hand was sufficient, or even representative to the analytical effort. Using data simply because it is immediately available is sometimes called convenience sampling and can often lead to false or incomplete conclusions.
Finally, the data science team used visualizations -- let us assume wonderful ones, considering the state of tools currently available -- to hand off the analysis back to those who make the pitch. Any deep understanding of the analysis is lost or, at best, not available at the time of the pitch. Even assuming there was some debate regarding issues and concerns with the stakeholders, these complicating factors are likely missing, at least in part, in the final presentation. Simplification is key to efficient decision-making, but oversimplification can lead to a misinformed decision.
As a result, it's important to keep in mind three useful best practices regarding data science storytelling:
- Involve stakeholders in the creation of the analytical narrative. This helps mitigate information loss, ensures clear understanding of the conclusions and mitigates loss of potentially critical nuance in the final decision.
- Carefully consider the data and analytical method. This supports empirical rigor -- for example, whether the result is replicable -- and guards against sampling and other biases.
- Aim for simplification that leads to the right decision. Oversimplification to the point of omitting details that may have changed the decision can be a critical shortcoming. Visualization should be used to tell a story, but not to obscure the critical points in the argument -- for example, what assumptions have been made, why the data is the right data to reach the conclusion, etc.
Death by data
As we consider what skills are relevant in the future state of enterprise decision-making, we should carefully consider important trends like federation.
As data and analytics become more widely available in the enterprise, it is natural that more individuals are asked to use data science skills to support their work. Just as when presentation software became available and nongraphics professionals were suddenly required to understand fonts, graphic representation and other skills, many workers may not be ready for the shift. The analytical equivalent of "death by presentation" can become "death by data."
As federation of data and analytics continues in the enterprise, leaders should carefully consider what steps they are taking to make sure the workforce is armed with the right skills -- for example, problem formulation, understanding bias and basic preconditions -- as well as the right support from dedicated analytical resources.
Powerful decisions
Another critical trend is leading with a solution. As AI and other methods become more common, many times, we find ourselves in a conversation about a tool or method, looking for a solution to apply. It's quite common for conversations to start along the lines of, "How can we use AI to understand customer reviews?" Or, "How can we use visualization to demonstrate how our new product works better?"
We must be very careful when we lead with a tool or technique -- science teaches us to lead with a question. Consider how the approach might change if we asked, "Do we have access to data about Y that is sufficient to understand what is going on?" Or, "What method could we use to analyze that data?" Or, "How precise do we need to be in order to make a decision?" These sorts of questions will still lead to data and analytics, but they are much more likely to lead to effective choices in data sets, methods and the ability to tell a story with the conclusions that drive a powerful decision.