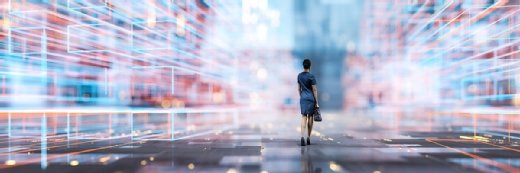
Getty Images
Citizen data scientist training for augmented analytics
Augmented analytics have made data analysis more accessible and led to the rise of citizen data scientists. But using these tools still require extensive training.
Even as companies invest heavily in digital transformations and become more data-driven, there's a dramatic shortage of data scientists.
According to QuantHub, there was a data scientist shortage of 250,000 people in 2020. In a report released earlier this month by tech career hub Dice, data scientist was one of the top five fastest-growing job roles this year, while LinkedIn's 2021 jobs report found hiring for data scientist jobs grew nearly 46% since 2019.
Augmented analytics bridges the gap
Augmented analytics tools offer the promise of addressing some of these shortfalls by making the technology more accessible to non-data scientists.
For example, analytics is increasingly being embedded into the applications that employees are already using, like Salesforce.
In addition, there has been a surge in the availability of low or no-code platforms such as H2O.ai, Knime, SparkBeyond, DataRobot, Rapidminer, Alteryx, SAS Viya and many more, said Amaresh Tripathy, global leader of analytics at Genpact.
"These platforms can automate the standard steps involved in traditional end-to-end data science projects," he said. "However, there are two major areas where 'humans-in-the-loop' are required."
Humans are needed to understand data within the context of a domain-specific application, he said, and to translate the insights into something that can be used to make business decisions faster.
"These areas are where citizen data scientists play a pivotal role," he said.
But without proper controls and training for these citizen data scientists in place, things can easily go wrong.
Take, for example, the question of correlation versus causation: The alarm clock goes off, and the sun comes up. Without an understanding of the underlying data set, and the domain expertise to know that the sun is going to come up whether the alarm is set or not, someone might conclude that the one causes the other. And therefore, if you change the time the clock is set for, you can make the sun come up earlier or later.
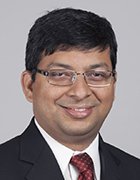
"Even expert data scientists make these mistakes all the time," Tripathy said. "But someone not as steeped in it is likely to make mistakes more often. If you don't understand the concept of causality, it could lead to things that are correlated in the wrong ways and result in bad business strategies."
There are other areas where analytics can go wrong. If a company has traditionally only hired white men for technical positions, for example, a resume screening algorithm might downgrade equally good resumes from women or minorities.
Another example is a scoring algorithm for loan applications might show a preference based on race based on historical trends. The easy fix, to remove race from the data set, might result in a proxy variable such as zip codes that has the same effect.
Either way, the company would get in trouble with regulators and end up with fines or public relations disasters on its hands. Depending on how they're using analytics, citizen data scientists may need training on core concepts, or on privacy, security or compliance issues.
Key data science skills for citizen data scientists
Before using the tools successfully, data scientists need to understand what data sets are relevant to the problem they're addressing, current trends and patterns relevant to that problem, and how to translate the insights they get from the data analytics platform into usable information.
That can require some additional training, Tripathy said.
Companies can deliver that training through sessions conducted by platform developers, in webinars, and with hands-on practical training. To get the maximum impact of that training, it should be based on data sets that are representative of the actual challenges those companies are facing, he added.
Genpact, a business transformation consulting firm spun off from GE in 2005, is doing exactly that.
To date, around 70,000 of its nearly 100,000 employees have gone through some degree of data literacy training, he said.
The in-house training program offers bite-sized customized learning paths in more than 70 different skills. In addition, employees are encouraged to enroll in a machine language incubator program where they get training in data science, augmented intelligence and visual storytelling platforms.
The program was created two years ago, and about 30,000 people from all backgrounds have completed the full program.
"There are people who are supply chain planners, claims processors, call center operators, risk management professionals, marketers," Tripathy said. "The program is designed for everyone. And the more diverse the backgrounds, the more interesting the ideas of how people will apply it."
One benefit of Genpact's data science training is lower attrition rates.
"We have higher engagement, we're building skill sets, so we have higher retention," he said.
There are also business benefits in being able to upskill existing employees when clients ask for new skill sets rather than trying to find people to hire.
Finally, employees with good analytics skills can better serve customers.
"You're sharing more interesting insights with clients, which increases the value of the service we provide," he said.
How long it takes to create a citizen data scientist
During the first year that Genpact had its program in place, the company focused on getting people through the basic concepts of data science.
The second year, it was all about solidifying the content and applying it.
"And now, it's about, 'are they connecting it to real projects and changing the work they are doing?'" Tripathy said.
But companies shouldn't focus on the length of any particular training programs, Tripathy said.
"You have to have a culture of learning," he said. "It's not a matter of time. Yes, some of the courses we have are micro-learning courses and in a week or 10 days you're going to get a lot of progress. But the real question is the immersion, and how you connect it to your day-to-day work."
Foolproof augmented analytics
Depending on the context, some augmented analytics tools might require no training at all.
For example, a tool that's embedded into an employee's workflow and that works within very narrow, predefined parameters might be so easy to use that employees can just start using it.
"The whole idea is to extend the democratization of self-service with the aid of computer assistance," said Doug Henschen, vice president and principal analyst at Constellation Research. "Only some of these features require training, and I wouldn't call it extensive training."
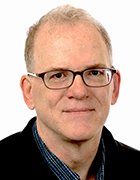
Intuitive add-ons to self-service business intelligence and analytics products can sometimes be mastered through experimentation, he said, or by reading documentation and help menus, or through guidance by analysts and power users.
"In many cases, vendors offer tutorial videos and online training courses," he added.
These might be appropriate for more sophisticated tools, like those that prepare data, or that are used for forecasts, he said.
Core skills for a citizen data scientist training program
Anand Rao, partner and global AI leader at PricewaterhouseCoopers, recommends that companies look at three levels of citizen data science training.
The first is digital upskilling. This is high-level training on different types of digital assets and how they relate to each other, he said, and includes data, analytics, automation and AI.
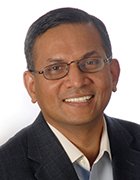
PricewaterhouseCoopers began this journey more than three years ago, Rao said, as a response to trends in the marketplace, client demands and employees wanting new skills so that they themselves could stay competitive.
The next level is business analytics, where a business or domain expert gets training on what kinds of business problems can be addressed with analytics and what the relevant data science solutions are.
Finally, citizen data scientists need data storytelling skills, he said. Depending on the educational background and prior experience, it takes three to six months to train a data scientist to be at the beginning level, he said, and six to 12 months to train one to be at an intermediate or advanced level.
"Citizen data scientists should be taught how to interpret the results of the different algorithms that they will use in the platform," Rao said. "In addition, they should also be taught how to tell a story using data, to highlight the insights and at the same time explain the evidence from the data."