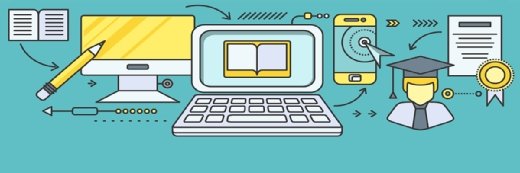
Certificates important to the data science learning path
While data science certificates have value in the professional world, candidates must make them a piece of a larger drive for technical mastery and continued education.
Certificates can help establish a benchmark of a data scientist's technical ability and skill, but their true impact on a resume remains unclear.
Organizations are looking for continued education and proof of technical ability for their open data science positions. Certificates can give candidates the skills to set themselves apart but -- for data scientists seeking an edge -- the data science learning path can never cease.
Certificates are a natural step on this journey but, above all, employers are looking for hard evidence of technical skill to match their open positions.
The value of a certificate
When it comes to data science, having a wide arrangement of skills and a drive to learn more are crucial. This is especially true for those seeking new positions.
"Continuing and pushing your skills in this field is the key to success," said Josh St. John, vice president of client delivery at Brooks Bell, an analytics and UX consultancy. "Data science, today, involves ever-evolving techniques and technologies that can make large impacts in what can be accomplished in business."
A common way for candidates to grow their skill set is through continuing traditional education and earning certificates. However, certificates are less powerful on the data science learning path than they used to be.
"Certificates have a muddled view of data science training," said Justin Richie, data science director at Nerdery. "Cloud certifications from AWS, GCP and Azure have more value than other certifications because they show an understanding of infrastructure."
The valuable certifications are the ones that can prove specific knowledge of a technical skill. These help organizations better understand what data scientists are familiar with, but even these certificates have limitations in their value.
Fortunately, most certificates that candidates can earn in the space are relevant to a specific technology, not an overall methodology. This is helpful because they can provide some evidence of technical familiarity, but they're tricky for those seeking a combination of skills.
Oftentimes certificates fall short of the value that on-the-job experience has in the hiring process because of their limited scope. Candidates would have to spend significant time collecting numerous certificates to truly stand out.
"Certificates are not as influential as solid technical ability and relevant project work," Richie said. "Most of today's data scientists come from all walks of life, so it's very hard to gauge skill set without more technical evaluations."
Getting the most out of certificates
To make the most use of certificates, it is important to understand that, while being certified doesn't necessarily make or break a candidate in the interview process, it can provide interviewers with insight to a candidate's knowledge level. Certifications can generally help match candidates to a position's responsibilities.
"In the interview process, certificates are most influential when they're relevant to specific skills required to fulfill the role responsibilities," St. John said.
But beyond that, having a long list of certifications does not make a candidate a preferred choice when another person displays a strong core in the base requirements.
"While certificates and certificate training programs are useful in growing data scientist skill sets, hands-on experience is always a much more effective teacher of these types of skills," St. John said. "When you combine the two, you get the well-rounded experience needed to grow in your role."
What matters in the interview process?
Balancing experience with certifications is crucial for candidates interviewing in data science.
"Relevant work is critical," Richie said. "The most important item that stands out to me in hiring decisions is a strong GitHub profile."
When it comes to the more large-scale hiring institutions such as Google and Facebook, proof of technical ability stands supreme over traditional weights like college degrees.
"Data preparation, analysis and presentation are baseline requirements for data scientists," St. John said. "This includes fundamental knowledge of statistical tools, databases, modeling and -- of course -- mathematical ability."
It is the demonstration of the abilities associated with certifications that is key for the candidate. Certificates are not enough if, during the interview, the skills can't be proved. And the best data scientists can prove these skills, as well as translate their knowledge to a nontechnical audience and therefore allow them to act on those recommendations accordingly.
It is only through investing in oneself and expanding technical capabilities that a data scientist can rise above other candidates.
"Continued education is essential," Richie said. "I envision continuing education and degrees as being the catalyst for maturity in the industry."