Big data vendors should stop dissing data warehouse systems
Wayne Eckerson examines the analytics roles of data warehouses and big data systems and says he's tired of data warehouse bashing by big data vendors.
I've heard so many big data vendors bash data warehouses as a way to justify their new technologies that it's getting annoying. To them, data warehouse systems are monolithic, costly and inflexible, while their technologies are fast, flexible and affordable. "Buy our products," they shout in their shiny collateral, "and we'll save you from data warehousing hell."
As if technology were the problem. Or the solution.
I'll admit there are plenty of data warehousing failures out there. Designing a data warehouse is not easy, and implementing one is even harder. The critics are right -- data warehouses take a long time to build, cost a lot of money and are hard to change. But that doesn't mean we should ditch them.
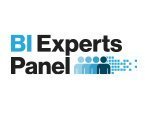
At its heart, a data warehouse is not a technology or tool. It is primarily a business process that unites an organization in electronic form (i.e., through data) so it can function as a single entity, not a conglomeration of loosely coupled fiefdoms. Without a data warehouse, business executives run blind, making critical decisions with inaccurate data or no data at all.
Although you need technology to implement data warehouses, technology can't harmonize business perceptions and deliver an enterprise view of an organization. Only business people can do that. In fact, getting business people to agree on the definitions of core business entities can be more challenging and time-consuming than creating the technical infrastructure. Instead of blaming technology or technologists for poorly designed or under-performing data warehouses, we should point the finger at executives who fail to provide sufficient leadership, vision and patience to create a common data vocabulary for doing business.
Data warehouse systems supply clean data
Big data vendors need to specify how they plan to deliver enterprise views and standard reports.
Technically, a data warehouse is a repository of clean, integrated and semantically unified data gleaned from major applications and systems in an organization. You can implement a data warehouse with a variety of technologies and tools, from relational databases to master data management hubs and Hadoop. Some technologies are better than others, and no technology is sufficient in and of itself. But that isn't the point. A data warehouse is really an abstraction, a logical representation of clean, vetted data that executives can use to make decisions.
Unfortunately, many in the big data community seem to advocate abandoning data warehouses altogether. Perhaps what they really mean is that they no longer want to use traditional relational databases and business intelligence tools to store and query business data. That's fine -- and welcome. New technologies bring benefits. But that doesn't eliminate the need for clean, integrated and certified data.
Big data vendors need to specify how they plan to deliver enterprise views and standard reports. Unfortunately, most ignore this annoying requirement or make it a small droplet in their big data lake.
The three pillars of an analytical ecosystem
Part of the problem is that the big data community inflates the role of a data warehouse before shooting it dead. The data warehouse is only one of several repositories in a mature analytical ecosystem, which also includes exploration/discovery and event-driven alerting environments (see Figure 1).
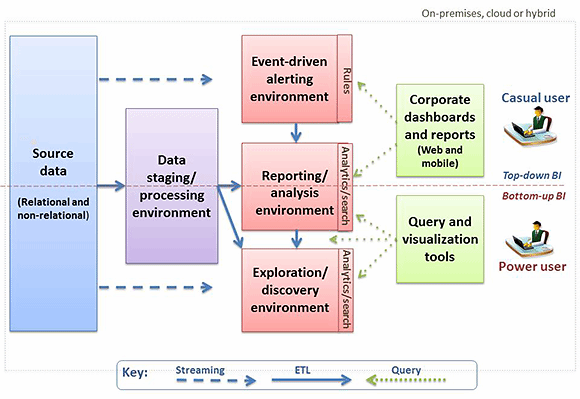
Simply put, the job of a data warehouse is to help business people monitor existing processes and activities and identify key trends and anomalies; it underpins a reporting and analysis environment that is designed to provide answers to predefined questions. Although a data warehouse supports some degree of analysis, it's not intended to answer new and unanticipated questions. That is the job of the exploration and discovery environment -- the hallmark of the big data movement today. It lets power users mash up new and existing data sets, run complex queries and apply machine learning algorithms to drive new insights. The alerting environment, meanwhile, handles event-driven data feeds from high-volume transactions or real-time processing systems and alerts users or downstream systems when data triggers predefined rules.
More expert insight from Wayne Eckerson
Learn why it's time to consider cloud-based BI systems
Find out why you need more than statisticians to develop effective analytical models
See what it takes to be a BI leader -- it's not just technology
Missing from Figure 1 is technology. As I mentioned above, you can implement data warehouse systems (and the other environments) using a variety of technologies and tools. Your choices depend partly on your organization's legacy systems, budget and tolerance for risk. But whatever you decide to use, make sure you understand how it all needs to fit together in a well-designed analytical ecosystem.
Finally, let's not allow big data advocates to denigrate the data warehouse. It plays a vital role in any analytical ecosystem. A data warehouse is the vehicle that delivers an enterprise view of data and drives standard reports and analyses. And who can live without that?
About the author:
Wayne Eckerson is principal consultant at Eckerson Group, which helps business leaders use data and technology to drive better insights and actions. His team provides information and advice on business intelligence, analytics, performance management, data governance, data warehousing and big data. Email him at [email protected].
Email us at [email protected], and follow us on Twitter: @BizAnalyticsTT.