Business Information
- Editor's letterAugmented analytics reveals the hidden side of things
- Cover storyAugmented analytics, automated tools facilitate data analysis
- InfographicAutomated predictive analytics tools spawn wannabe scientists
- FeatureData science teams use business ties to boost data knowledge
- ColumnUCSD graduates to advanced analytics with SAP HANA platform
- ColumnWhat-if business planning simulation at its predictive best
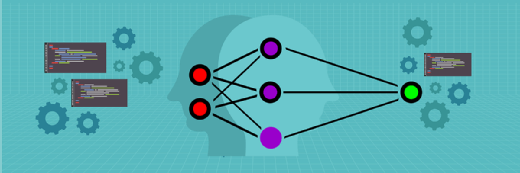
Augmented analytics, automated tools facilitate data analysis
From Tableau to Google, vendors increasingly incorporate augmented and automated analytics tools into their software to speed analytics work and open it up to more users.
Augmented analytics sounds futuristic -- and BI and advanced analytics vendors are increasingly moving to make it a here-and-now reality, along with automated machine learning, search-based querying and other technologies that have a common goal to simplify and streamline the analytics process for users.
In particular, augmented analytics and its cohorts are designed to make it easier for business users and the growing population of so-called citizen data scientists -- business analysts and other workers who lack formal data science training but are building predictive models themselves -- to drill down into data sets and gain valuable insights from BI and analytics applications.
These emerging technologies do arrive at the same point, but they come at it from different directions. Augmented analytics -- or augmented intelligence, as some vendors characterize it -- embeds machine learning algorithms and other advanced analytics functionality into BI tools to point users toward relevant data, help them prepare it for analysis and aid in creating data visualizations. On the other hand, automated machine learning platforms provide under-the-hood functionality to help analysts with limited expertise build and train machine learning models for more-advanced data mining and predictive analytics uses.
But while the simplification push encompasses a broad set of tools and approaches, it all boils down to enabling users -- especially less tech-savvy ones -- to uncover information faster than before. These technologies also pave the way for more people to get involved in analytics initiatives and can be "a big productivity booster" for organizations, TDWI analyst Fern Halper wrote in a September 2017 report on advanced analytics trends.
According to Gartner, augmented analytics includes a combination of natural language processing (NLP), augmented data preparation, automated analytics and visual-based data discovery. As a whole, those capabilities have yet to become a mainstream factor in software buying decisions, Gartner said in its 2018 "Magic Quadrant" report on BI and analytics platforms.
But the consulting and market research firm predicted that augmented analytics, by 2020, "will be a dominant driver of purchases" of BI tools, embedded analytics software, and data science and machine learning platforms. And by then, Gartner expects 50% of analytical queries to be automatically generated or created through searches, NLP applications or voice commands instead of conventional coding.
Longer term, Gartner sees technology-assisted analytics becoming even more prevalent. "Ten years from now, probably, you won't find a single business application that isn't enabled in some way by machine learning and AI, and analytics is no different," Gartner analyst Rita Sallam said in a recent webinar on augmented analytics.
Some users are already on board. In a TDWI survey conducted in June 2017, 27% of 222 respondents said their organizations were using analytics tools that suggest data visualizations to users. And in another survey done by TDWI in January 2018, 16% of 387 respondents said their organizations had deployed automated predictive modeling software, while an additional 40% said they expected to start using it in the next few years.
Giving machine learning the gas
Chevron Corp. is an early adopter of Google's Cloud AutoML technology, which is designed to help users with limited machine learning expertise build and train analytical models. Earlier this year, a seismic processing and imaging team at Chevron used the alpha version of an AutoML Vision image analysis tool to help analyze internal documents as part of the process of evaluating opportunities for oil drilling, according to Laura Bandura, a research geophysicist who works on the team.
Bandura, speaking during a keynote session at July's Google Cloud Next '18 conference in San Francisco, said Chevron has amassed millions of documents related to oil exploration in different areas -- far too many to search manually. As a result, finding relevant information about an area that's being assessed for possible drilling "can be a needle-in-a-haystack type of challenge," she explained.
To pinpoint the documents that might have useful geological info about locations in the Gulf of Mexico, the Chevron team did a search on the term geologic map to find documents with embedded map images. It next ran an analytical model that was built with AutoML Vision and trained to recognize more than 60 geologic labels against the map images, then used Google's pre-trained Cloud Natural Language API to classify the data found by the model and make the information searchable.
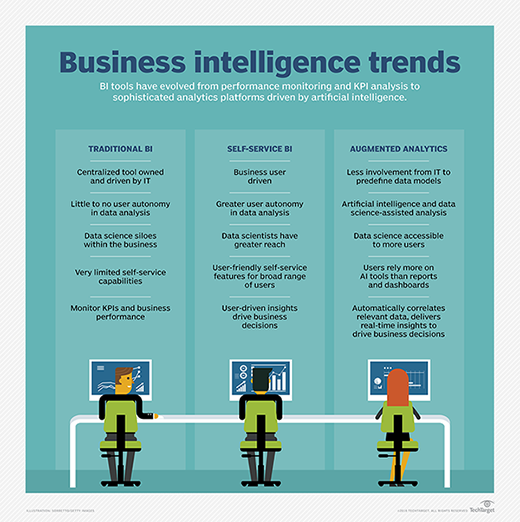
Thanks to the use of AutoML, Bandura said that she and other Chevron explorationists, as they're called in oil industry parlance, can now find pertinent documents about the geology of the gulf "in seconds instead of weeks." That enables more-timely decisions on possible drilling spots, without involving data scientists in the model development and training process, she added.
AutoML Vision became available as an alpha technology last January and was released in a beta version in July along with companion products for text analysis and language translation. Also in July, Google launched a beta version of BigQuery ML, which lets users build machine learning models inside the company's BigQuery cloud data warehouse with standard SQL commands instead of using more-advanced languages like R, Python and Scala.
A wider analytics embrace
Other top BI and analytics vendors are also embracing augmented analytics and other forms of automation. Tableau Software, for example, offers a recommendation engine that suggests relevant data sources to users of its self-service BI and data visualization software. It also built AI-based fuzzy clustering algorithms that help users group together related data sets into the Tableau Prep data preparation tool released in April.
In June, Tableau acquired AI startup Empirical Systems, developer of an analytics engine that automatically models data for analysis and identifies trends and outliers in the data. It plans to integrate the Empirical engine into its BI software in the hope of providing users with better insights into their data sets.
Tableau's chief product officer, Francois Ajenstat, said the company is also looking to use NLP technology so business users can intuitively ask questions about data to be analyzed without seeking help from a data scientist. Ajenstat wouldn't specify when that capability will be available, saying only that Tableau hopes to incorporate it "soon."
Qlik, one of Tableau's top self-service BI rivals, is likewise working to incorporate augmented analytics capabilities into its Qlik Sense software. Updates to Qlik Sense earlier this year included the addition of a feature that recommends chart designs based on the data fields selected by analysts and an Insight Advisor tool that can direct users to data that fits analytics applications they want to run.
Not to be outdone, Tibco Software is adding the ability to use NLP queries to navigate through data sets and get AI-driven visualization recommendations to its Spotfire self-service BI software. Those features, which join an existing AI function that automatically identifies relationships between data elements, are part of a new Spotfire X release announced last month and due out sometime in the fall.
Augmenting AI's cache
Other tools that offer augmented analytics capabilities include Salesforce Einstein and IBM Watson Analytics. In addition, IBM last month detailed an update to its traditional BI platform, Cognos Analytics, that includes natural language querying, automatic pattern detection and visualization recommendation features. Among other BI vendors adopting the concept, Sisense Inc. is using NLP technology to power a free chatbot application that lets users upload structured data, which the tool analyzes for basic pattern and anomaly detection and other insights (see "Say hello to your friendly little chatbot data analyst").
Augmented and automated analytics technologies offer a path for making AI more prevalent -- and more useful -- in user organizations, according to Guy Levy-Yurista, vice president of strategic growth and innovation at Sisense. "People all get excited about AI, but very few people know what it can do," he said. "Analytics should be embedded in your day-to-day life, and you should be consuming analytics without really thinking about it."
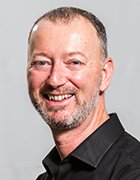
In addition to Google, automated machine learning platforms are available from vendors such as DataRobot and H2O.ai. Microsoft also added some automated functionality to its cloud-based Azure Machine Learning platform last month, enabling the software to identify algorithms that will run applications efficiently and optimize the performance of analytical models for users.
And there are plenty of startups working on augmented tools, like Ople.ai, which offers a platform that can be used to build AI models for accelerating data science applications, as well as the many companies that are part of the MIT Startup Exchange -- about 70 of which are working on AI projects. One such startup is Feature Labs; its software helps automate feature engineering by using automated techniques to turn raw data into machine learning algorithms.
Say hello to your friendly little chatbot data analyst
Chatbots are nothing new, but Sisense's Boto is a bit different. The free chatbot works across Facebook Messenger, Skype and Slack. Users access Boto simply by adding it to their contact list and using a drag-and-drop interface to upload data the bot can automatically analyze.
Insights generated by Boto are fairly basic compared to what a data scientist or other software could produce. Essentially, Boto is designed to demo Sisense's products and the general public to analytics, according to Levy-Yurista.
Natural language processing is becoming far more prevalent in our daily lives. From Apple's Siri to Amazon's Alexa to Microsoft's Cortana, more and more devices today are capable of understanding your words and responding to your questions.
NLP, specifically the chatbots that use it, is big right now and something "we should see a lot more of," Levy-Yurista said, adding that "investment in bots is a harbinger to a generation" of technology. He believes future bots "will serve as an agent operating on the behalf of the human" and act as "a true advocate on your behalf."
That might mean bots going out into the world or on the internet scheduling meetings for you, looking for and applying to jobs -- or perhaps even aiding in data analysis and analytics.
Software that automates advanced analytics processes "allows people with very little data science training to become data scientists," said Marcus Dahllöf, program director at the MIT Startup Exchange. Hence, the purpose of augmented analytics: Workers who aren't skilled data scientists can at least act like they are.
Senior Executive Editor Craig Stedman contributed to this story.