8 benefits of using big data for businesses
Big data is a great resource for driving smart business decisions and changes. Here are eight ways that the use of big data is improving how business gets done.
When business leaders hear the term big data, they most naturally think of the massive volumes of data available today. This data is created by e-commerce and omnichannel marketing systems, IoT-connected devices or business applications that generate ever more detailed information about transactions and activities. And those are just a few examples.
The sheer scale of the data is daunting, maybe even overwhelming in some cases. But there are great business benefits to be gained by analyzing sets of big data. We'll explore some of these benefits below, but first let's get a clear idea of what we're talking about -- and there's more to it than the amount of data.
What is big data?
The term isn't entirely misleading -- the volume of data involved can indeed be staggering -- but don't mistake it for a complete definition. Big data platforms are certainly optimized for large data sets, but I've seen many data lakes built to store big data that were smaller than conventional data warehouses in the same organization. Nevertheless, it's generally true that big data tends to be pretty big.
So, what else is involved? One key aspect is the multiplicity of data types involved. A single big data system might contain XML documents, raw log files, text files, images, video, audio and traditional structured data. This is commonly called the variety of big data and being able to store and process some of these data types -- especially images, video and audio files, which can be very large -- does require a system that's capable of scaling quickly and easily.
Another twist to this is the velocity of the data. That refers to the speed at which it is generated or updated. For example, those log files from monitoring systems, mobile applications, websites and other sources often consist of a continuous stream of readings, perhaps thousands in an hour. You can have big data without such velocity, but a well-designed big data architecture should be able to handle it.
Many analysts and practitioners have expanded these V's of big data to include other characteristics, such as veracity and variability. In summary, though, big data typically is a resource with many types of data and the potential for great scale and rapid updates. It also encompasses new ways of storing, processing, managing and analyzing the data that drives business decisions. These new techniques are what enable the big data benefits that business executives and IT teams alike are seeking.
Now, let's look at eight ways in which big data can improve the way we do business.
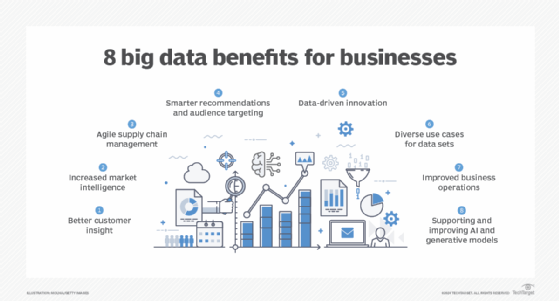
1. Better customer insight
When a modern business turns to data to understand its customers, whether individually or in categories, it has a wide range of sources to choose from. Big data sources that shed light on customers include the following:
- Traditional sources of customer data, such as purchases and support calls.
- External sources, such as financial transactions and credit reports.
- Social media activity.
- Data from internal and external surveys.
- Computer cookies.
Clickstream analysis of e-commerce activity is especially useful in an increasingly digital marketplace, shedding light on how customers navigate through a company's various webpages and menus to find products and services. Companies can see which items customers added to their carts but perhaps removed or later abandoned without purchasing; this provides important clues as to what customers might like to buy, even if they don't make a purchase.
Not only online stores, but brick-and-mortar locations can also glean useful understanding of their customers, often by analyzing video to learn how visitors navigate through a physical store compared with their navigation of a website.
2. Increased market intelligence
Just as big data can help us analyze the complex shopping behavior of customers in more detail, it can also deepen and broaden our understanding of market dynamics.
Social media is a common source of market intelligence for product categories ranging from breakfast cereal to vacation packages. For almost any commercial transaction you can imagine, there are people out there sharing their preferences, their experiences, their recommendations … and their selfies. Yes, even of their breakfast fare. These shared opinions are invaluable for marketers.
In addition to competitive analysis, big data can also help in product development -- by prioritizing different customer preferences, for example.
In fact, big data does not just assist with modern market intelligence; in almost any e-commerce or online market, almost all market intelligence is driven by diverse, ever-changing data.
3. Agile supply chain management
Whether because of pandemic-driven shortages of toilet paper and other goods, the trade disruption of Brexit or a ship stuck in the Suez Canal, you should be aware by now that modern supply chains are surprisingly fragile.
It's surprising because, mostly, we don't notice our supply chains until there is a truly major disruption. Big data that enables predictive analytics, often in near real time, helps to keep our global network of demand, production and distribution working well for the most part.
This is possible because big data systems can integrate data on customer trends from e-commerce sites and retail applications with supplier data, real-time pricing and even shipping and weather information to provide a level of information not seen before.
It's not just large enterprises that benefit from these insights. Even modestly sized e-commerce businesses can use customer intelligence and real-time pricing to optimize business decisions such as stock levels and risk reduction, or temporary or seasonal staffing.
4. Smarter recommendations and audience targeting
In our lives as consumers, we are now so familiar with recommendation engines that we might not be aware of how much they have evolved since the advent of big data. At one time, the predictive analysis for recommendation engines was quite simple: association rules, which found those common items in market baskets. You can still expect to find this as a feature on e-commerce websites telling us that customers who bought widgets also bought fidgets.
Newer recommendation systems are much smarter than that, building on the sophisticated customer insights we have already discussed, with the result that they can be more sensitive to demographics and customer behavior. These systems aren't limited to e-commerce, either. A friendly waiter's recommendations might well be data-driven -- decisions prompted by a point-of-sale system that evaluates stock levels in the pantry, popular combos, high-profit items and even social media trends. When you share a picture of your meal, you are providing yet more input for the big data engines to digest.
Streaming content providers use even more sophisticated techniques. They might not even ask customers what they want to see next: Even before the current movie, program or song finishes, the next selection fades in, keeping viewers binge-watching by utilizing their own preferences combined with a great deal of big data analysis gleaned from other users and social media.
5. Data-driven innovation
Innovation is not just a matter of inspiration. There's a great deal of hard work in identifying subject areas that are promising for new efforts and experiments.
The various big data tools and technologies that are available can enhance R&D, often leading to the development of novel products and services. Sometimes the data -- cleansed, prepared and governed for sharing -- becomes a product in itself. The London Stock Exchange, for example, now makes more money from selling data and analysis than it does from securities trading.
Data by itself, even with the best big data tools, will not produce new insights. We still need the human element: the understanding and imagination of data scientists, BI analysts and other analytics professionals. However, the breadth and scope of big data, especially when stored in a single Hadoop cluster or cloud data lake, can lead teams to a new understanding of trends that would be difficult to glean in a less integrated environment.
6. Diverse use cases for data sets
Several times in my career, I've seen cases where data that was carefully prepared and modeled for one business purpose was completely unsuitable for another one.
For example, the marketing team at a credit card issuer wanted to understand how customers used the different cards they had in their wallets. The analysis was made more difficult by the numerous failed swipes and canceled transactions that were common at the time, often due to connection problems with the payment terminal or flaws in the magnetic stripe on the cards. So, the data was carefully cleaned up to remove the failed transactions.
The result was a data set that was great for the initial marketing application. But the fraud prevention team couldn't use it because they wanted to see those failed transactions that might have left clues about fraudulent card usage. Not only that, but the removed data was being archived onto tape storage and therefore was hard to access.
In the age of big data, we can store all of the raw data as is in a data lake and only apply data models to it when we need to use it for particular analytics applications. We can then design data pipelines specifically for each use case or just run ad hoc queries to populate the analytics processes. This enables great flexibility in the number and types of applications that can be run against the same data set.
7. Improved business operations
Business activity of all kinds can be improved by using big data. It helps optimize business processes to generate cost savings, boost productivity and increase customer satisfaction. Hiring and HR management can become more effective. Better fraud detection, risk management and cybersecurity planning help organizations reduce financial losses and avoid potential business threats.
One of the most interesting and rewarding applications for big data analytics is to improve physical operations. For example, the combination of big data and data science can inform predictive maintenance schedules to reduce costly repairs and downtime for critical equipment and systems.
You can start by analyzing the age, condition, location, warranty and service details. However, things such as the security and HVAC systems in facilities are notably affected by other business activities, such as staffing and production schedules, which might, in turn, be influenced by sales cycles and, therefore, by customer behavior. Well-integrated sets of big data pull all this together to help you maintain equipment at the optimal time.
8. Supporting and improving AI and generative models
Artificial intelligence, especially generative AI (GenAI) and large language models (LLMs), is transforming business operations and innovation. These advanced AI systems rely heavily on vast amounts of training data to learn patterns, understand context and generate humanlike text, images and other content. Within the context of your own business, big data enables the customization and improvement of GenAI tools.
One area of AI that holds great promise is retrieval-augmented generation (RAG). This approach combines the strengths of LLMs with the ability to query relevant information from extensive knowledge bases, such as your own corporate data. By harnessing big data technologies, organizations can build comprehensive knowledge repositories that RAG models can access in real time, generating more accurate, informative and contextually relevant responses.
For example, a customer service chatbot powered by RAG could draw upon a company's entire product information history, user manuals and customer interactions to provide highly personalized and effective support. Similarly, a content creation platform could use RAG to generate articles, reports or marketing copy incorporating the latest industry data and trends.
Big data empowers businesses to continuously update and refine their AI models based on new information and user feedback. For example, by collecting and analyzing data on how users interact with GenAI applications, companies can pinpoint areas for improvement, fine-tune their models and create more engaging and valuable user experiences over time.
How to get started with big data
With all these potential benefits, you might wish to start your big data journey sooner rather than later. But what are the first steps to take? I think the following three are critical.
Prepare the big data infrastructure. Your data needs to go somewhere for processing and analysis. It's a simple matter to provision a data lake on a cloud platform, especially if you're already working with a cloud vendor. In fact, it's often as simple as creating a storage account, giving the data lake a name and getting your connection string and credentials. Most cloud vendors provide easy-to-use tools to do this.
Of course, you can also build your own data lake, perhaps with a hybrid cloud architecture that includes cloud and on-premises systems.
Define data lake zones. In practice, most data lakes aren't merely mass stores of unorganized data. It's useful to organize them into different zones, each with different purposes and often with separate permissions for different groups of users.
Commonly, the first one is the landing zone, sometimes called the raw or ingestion zone; it's where new data is added to the data lake with minimal processing. Second is the production zone, where data that has been cleansed, conformed and processed is stored. This one is most similar to a data warehouse, but it's typically less constrained and structured.
There's usually also a working zone or sandbox, where developers and data scientists can store temporary files and data structures for their projects. Finally, depending on your business, it might be necessary to have a private or sensitive data zone with very restricted access to ensure that critical data sets are properly governed.
Catalog the data assets. Because of all the variety of data that can be stored in a big data system, it really is essential to provide a user-facing catalog of the available data resources. A cloud platform vendor might offer its own basic cataloging and search system. In many cases, though, creating a data catalog that's geared to the needs of data scientists, business users and developers might be preferable.
Big data's benefits are worth the effort
With this basic infrastructure in place, you're almost ready to open your big data system to users. But some training is required, as the big data environment might be quite different from familiar database and data warehouse systems. You'll also need to think through access rights, permissions and other security and data governance requirements. The big data journey really only starts here.
Nevertheless, the business advantages and benefits that you can achieve with big data are well worth the effort. Big data is the lifeblood of modern business and one of your greatest resources for driving smart, sustainable change in an organization and gaining a competitive advantage over business rivals.
Donald Farmer is the principal of TreeHive Strategy, who advises software vendors, enterprises and investors on data and advanced analytics strategy. He has worked on some of the leading data technologies in the market and in award-winning startups. He previously led design and innovation teams at Microsoft and Qlik.