5 augmented analytics examples in the enterprise
Here are the top examples of augmented analytics uses that BI vendors support and enable, including data preparation, NLP-based querying and automated insights.
As business intelligence and analytics providers seek to boost the usability of their platforms, they're increasingly adding augmented analytics to their product and feature mix. As such, real-world augmented analytics examples have already started piling up in the enterprise.
Augmented analytics enhances the statistical number-crunching of continuously collected data points with advanced features that make it easier for both BI analysts and regular business user to tap into insights. These baked-in features include artificial intelligence, automation and natural language processing (NLP) for easier queries.
"Over the past decade, we have seen such a widespread explosion of the availability of data from so many different sources and channels that, regardless of size, brands need help organizing this data and making sense of it," said Ben Gaines, director of product management for Adobe Analytics Cloud, when explaining the role of augmented analytics in BI today.
That role is one that will grow in importance as the economy -- and the way business decisions are made -- is increasingly data-driven, according to Daniel Raskin, CMO of analytics firm Kinetica.
This article is part of
Ultimate guide to business intelligence in the enterprise
"In the extreme data economy, there will be winners and losers: those who collect data, and those who know how to use it," said Raskin. "It's not enough to stockpile data and analyze it on demand anymore. Now, businesses need to capture data, continuously assess it and instantly take action. If you can combine and analyze billions of live and historical data points continuously and automatically, you shape your decisions instantly."
This need is translating into tremendous growth for augmented analytics. According to Market Research Future, the global augmented analytics market is on track to grow 24% annually through 2023, when it is expected to become a $13 billion market.
Here, experts sound off on real-world augmented analytics examples, which, on a broad level, tend to fall into five major categories of analytical enhancement.
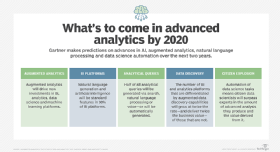
Data preparation
The first big use case for augmented analytics is in data preparation.
"Augmented and smart analytics reduces all the painstaking processes that data analysts need to do every time they receive new data sets to work with," said Krzysztof Surowiecki, managing partner at data analytics company Hexe Data.
Surowiecki explained that data analyst traditionally spend 80% of their time "cleaning" data through the extract, transform and load (ETL) process. Augmented analytics can be used to automate the process of ETL so that the people interacting with the data flip that ratio and spend more time thinking about the implications of the data, deriving insights from it and proposing recommendations to help the business, he said.
Improving 'queryability' of data
Another of the top real-life augmented analytics examples is around the use of AI to boost the "queryability" of data, Gaines said. That means making it easier for individuals across the enterprise to ask questions of -- and interact with -- the data.
"NLP really comes in handy here," said Gaines. "The ability to type or speak a question in one's own natural language and have a tool 'translate' that speech into a query that produces a meaningful result means that people who don't necessarily understand how to apply a filter or add a dimension to a report can now begin to understand their business on a data-informed level."
One example of this use case is unfolding at the insurance company Allianz Italy. The company built a platform called Allianz Virtual Advisor using augmented analytics technology from the startup iGenius. The platform allows insurance agents to use their smartphones to access data about income monitoring, performance of a particular product or client profiles using voice or typed natural language queries.
"The new virtual advisor is an extra colleague for our network of professional agents," said Agostino Ferrara, chief operating officer of Allianz, in an iGenius post. "[It's] an always-available coworker able to process vast amounts of data in a very short time and effectively support our Agents in their day-to-day operations, tapping into the extraordinary potential of conversational artificial intelligence."
Enabling citizen data scientists
This improved queryability not only allows data professionals to delve deeper into data, but also broadens the user base of analytics products. Augmented analytics are key for enabling citizen data scientists across the enterprise.
"Companies will no longer require candidates with experience in statistics and mathematics background [to do BI]," said Surowiecki. "They would be able to hire less-technical people who understand their business, can conduct insights and offer recommendations based on the delivered data. That would be a very interesting change that we can observe in the nearest future."
When well-employed, AI applications should lower the barrier of adoption to help non-technical business users feel data-oriented by offering them comfortable ways of looking at analysis created for them, Gaines says. This will help them derive insights and start digging deeper by asking the less apparent next question of data analysis.
"Static dashboards often aren't enough to answer deep questions," said Gaines. "Where AI and ML can contribute is in knowing what that next question should be, ensuring that brands are leveraging data in the right way and making that next question easier for everyone -- from the CEO to the front-line marketing manager."
"Suddenly, analytics capabilities are a lot more than just pretty bar graphs and pie charts -- they become a two-way conversation where the business can truly ask questions and get answers," said Gaines.
Tapping into 'tribal knowledge'
At many industrial firms, the aging workforce is starting to become a big concern, said Heena Purohit, senior product manager for IBM Watson IoT. She explained that in the oil and gas industry, a big chunk of their experienced workforce is expected to retire in the next five to 10 years. The fear is that the departure of these retiring gurus is going to put companies under severe risk of brain drain.
"Companies are now looking for innovative ways to retain their tribal knowledge and expertise, and augmented intelligence is helping them in this pursuit," she said.
In the industrial arena, IBM customers are using the NLP capability of an IBM Watson-powered feature called the Equipment Maintenance Assistant. It processes unstructured and structured data across a treasure trove of documents that includes manuals, standards, safety procedures, reports and historical work logs. Based on that ingested data, the assistant platform is then able to answer questions asked by maintenance and operations technicians.
One Australian oil and gas company had this augmented analytics feature absorb 30 years of engineering and drilling knowledge to help technicians tap into it to make fact-driven decisions about complex projects.
"Using this solution, technicians and operators reduced the time spent finding data by 75%, which translates to a $10 million savings in employee costs because of faster access to information and more intuitive analysis of engineering records for the organization," Purohit said.
Automation of insights
The last of the augmented analytics examples revolves around improving the automation of insights. The idea is to use repeatable machine learning tools to automate certain types of analyses that would take a data science team months to build on their own.
For example, Adobe built a machine learning tool called Segment IQ that offers button-click comparison of two groups of customers and compares them across hundreds of different dimensions. It's a common analysis outside of machine learning, but one which takes teams months or years to do. Having that tool available makes it possible to confirm or refute intuition-based hunches from leadership on the fly.
Gaines pointed to one B2B services company his firm was working with that had a major executive considering decreasing budget for paid search due to poor conversions from the investment.
"The marketing team created a segment of visitors who came to the site via paid search and compared them to all other visitors using Segment IQ," he said. "They discovered that although these visitors were not as likely to convert directly, they were three times more likely to upsell on a previously purchased service. The marketing team was then able to focus their budget on just the upsell-related keywords and saw a 56% increase in service upsells."