PRO+ Premium Content/Business Information
Access your Pro+ Content below.
Big data throws bias in machine learning data sets
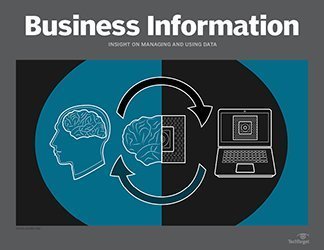
This article is part of the Business Information issue of February 2018, Vol. 6 No. 1
Say you're training an image recognition system to identify U.S. presidents. The historical data reveals a pattern of males, so the algorithm concludes that only men are presidents. It won't recognize a female in that role, even though it's a probable outcome in future elections. This latent bias is one of the many types of biases that challenge Data scientists today. If the machine learning data set they use in an AI project isn't neutral -- and it's safe to say almost no data is -- the outcomes can actually amplify discrimination and bias in machine learning data sets. Visual recognition technologies that label images require vast amounts of labeled data, which largely comes from the web. You can imagine the dangers in that -- and researchers at the University of Washington and University of Virginia confirmed one poignant example of gender bias in a recent report. They found that when a visual semantic role labeling system sees a spatula, it labels the utensil as a cooking tool, but it's also likely to refer to the person in ...
Features in this issue
-
Big data throws bias in machine learning data sets
AI holds massive potential for good, but it also amplifies negative outcomes if data scientists don't recognize data biases and correct them in machine learning data sets.
-
AI functionality limited today but could be a game-changer
Limited AI capabilities could soon give way to technology that is truly transformative for enterprises, surpassing the overhyped functionality that we see today.
Columns in this issue
-
AI technologies have come far, but the road is long
Machine learning and other artificial intelligence technologies are poised to offer businesses big benefits, but companies have to walk before they run with AI.
-
Cognitive computing in healthcare mends doctor-patient gaps
Cognitive computing and healthcare data aggregation may prove to be important bedside companions to doctors treating their patients in the era of medical specialization.