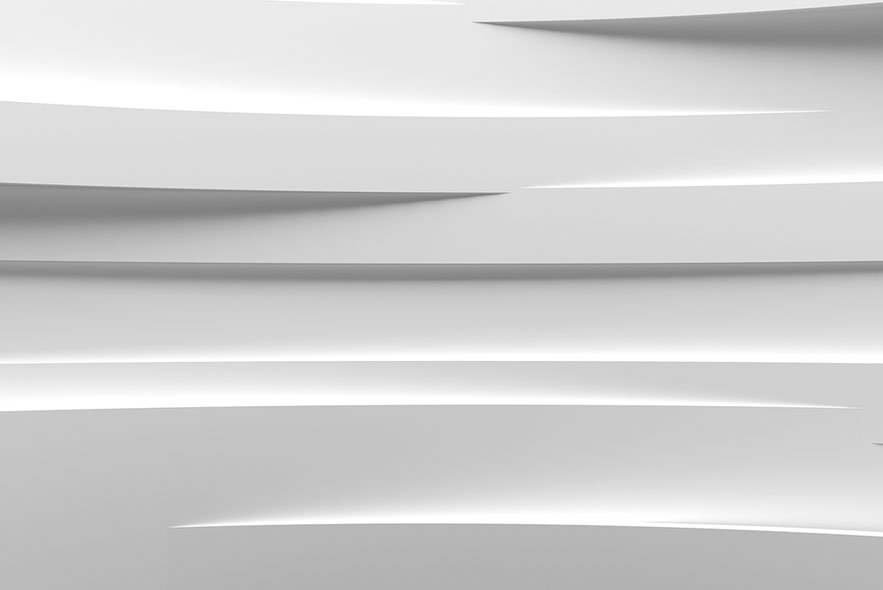
What is decision intelligence?
Decision intelligence is an emerging field that combines data science, artificial intelligence (AI) and decision theory to improve organizational decision-making.
It's important to note that the definition of decision intelligence is not always uniform. Analyst firm Gartner narrowly defines decision intelligence as the process of documenting and engineering how decisions are made. However, the broader market -- including vendors that participate in the space -- typically takes the larger view with the technology being a set of tools that combines business intelligence, AI and other data science functions to help drive decision-making.
The core concept of decision intelligence is that decisions are based on understanding how actions lead to outcomes. It provides a framework for analyzing this chain of cause and effect, using visual decision modeling to represent these relationships.
Decision intelligence platforms typically integrate and analyze data from various sources, including both structured data and unstructured data. AI and machine learning algorithms are used to help discover patterns, predict trends and generate actionable insights. These insights are then presented to decision-makers in accessible formats such as dashboards and visualization tools.
Decision intelligence aims to democratize data analysis, making insights accessible to both technical and nontechnical users across an organization. It's designed to help organizations navigate complex systems, deal with information overload and make more accurate, data-driven decisions.
Why is decision intelligence valuable to organizations?
Decision intelligence is valuable to organizations as they face growing complexity in their business environments.
With more complexity comes more challenges and the associated risk of making incorrect decisions. The promise of decision intelligence is that it lets companies benefit from data assets more effectively, turning raw information into actionable insights that lead to better outcomes. By providing a structured, data-driven approach to decision-making, decision intelligence helps organizations reduce risks, increase efficiency and identify new opportunities for growth.
Decision intelligence technology platforms let organizations manage, integrate and analyze data from virtually any source, with the ability to scale up to handle big data volumes. It augments human decision-making capabilities, allowing organizations to make faster and more accurate decisions.
How does decision intelligence work?
There are multiple steps in the decision intelligence process.
1. Data ingestion
Decision intelligence begins with the ingestion of data from multiple sources, that are both internal and external to the organization. This process can handle both structured and unstructured data, including text, audio, images and video. The system then integrates the different data sets into a unified data pool, aligning them into a uniform format that can be easily analyzed.
2. Entity resolution
Once data is ingested, the system performs entity resolution. This step matches all relevant data points belonging to the same entity, whether it's a person, organization, product or account. The result is a multisource profile for each entity, providing a comprehensive view of all available information.
3. Data enrichment and AI processing
The system then enriches the data using AI and machine learning techniques. This process includes extracting insights from data, such as tagging objects and detecting image-inferred relations. These processes add valuable context and make unstructured data more analyzable.
4. Advanced analytics
With the enriched data in place, decision intelligence platforms will then apply advanced analytics. This includes using machine learning models for pattern recognition and anomaly detection, employing predictive analytics to forecast trends and outcomes.
5. Visual decision modeling
Decision intelligence makes use of visual decision modeling techniques to represent decision processes and map cause-and-effect relationships between actions and outcomes. This step creates visual representations using tools like link analysis, heat maps and timelines, making complex data relationships more understandable.
6. Automated insight generation
The system automatically surfaces patterns, trends and anomalies within the data. It generates risk scores, predicts similarities between entities and infers relationships. This automated insight generation helps uncover valuable information that might be missed by manual analysis.
7. Decision support and automation
Based on the insights generated, the system presents actionable recommendations to decision-makers. Depending on the level of implementation, this can range from decision support that provides information to inform human decisions, to decision augmentation that offers AI-generated recommendations for human review, to decision automation that makes and implements decisions based on predefined criteria.
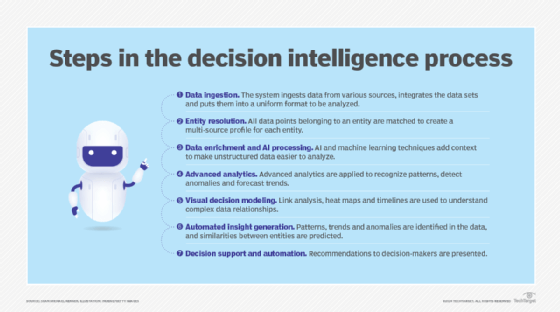
What are the key components of decision intelligence systems?
Key components of decision intelligence systems include the following:
- Data management and integration. Handles large volumes of diverse data types with the ability to integrate different data types plus APIs and connectors to various data sources and other systems.
- AI and machine learning. Uses a range of techniques, including predictive analytics, natural language processing and computer vision.
- Advanced data visualization. Offers interactive dashboards, network graphs and geospatial mapping for intuitive data representation.
- User-friendly interface. Provides self-service analytics capabilities accessible to both technical and nontechnical users.
- Security and compliance. Includes fine-grained access controls and audit trails to ensure data protection and regulatory compliance.
- Collaboration features. Tools for information sharing and teamwork across the organization.
Potential benefits of decision intelligence
Decision intelligence has numerous potential benefits that can help organizations. That includes the following:
- Enhanced decision accuracy and speed. Decision intelligence helps to improve human decision-making by using AI and advanced analytics to process vast amounts of data quickly. This leads to faster, more accurate decisions based on comprehensive data analysis rather than gut instinct.
- Comprehensive data integration and analysis. By integrating data from multiple disparate sources, decision intelligence provides a comprehensive view of all available information. This breaks down data silos and enables more thorough analysis.
- Bias reduction and objectivity. Decision intelligence helps minimize cognitive biases and analytical blind spots by providing data-driven insights. This leads to more objective decision-making and can help organizations avoid costly mistakes based on preconceived notions or incomplete information.
- Improved risk management. Decision intelligence can help to enhance risk assessment accuracy and enables more targeted approaches to risk mitigation.
- Proactive decision-making. Decision intelligence uses predictive analytics to model likely outcomes, enabling organizations to take a more proactive approach to decision-making. This allows for better preparedness and strategic planning in the face of future challenges and opportunities.
How can AI help with decision intelligence?
AI has become a foundational element of modern decision intelligence. Automated data analysis and insight generation are at the core of AI's contribution to decision intelligence. AI algorithms can rapidly process large amounts of structured and unstructured data, uncovering patterns and insights.
AI also enhances decision intelligence through entity resolution and relationship mapping across disparate data sources. This creates a more comprehensive view of the available information, breaking down data silos and enabling more thorough analysis. Additionally, AI can perform these tasks in real time, allowing for faster decision-making.
Machine learning is another critical component, allowing systems to learn from historical data and improve decision-making capabilities over time without explicit programming. This enables useful predictive analytics, where AI can forecast future trends and outcomes based on past data, helping organizations make proactive, data-driven decisions.
Decision intelligence vs. business intelligence and data science
Decision intelligence has some overlap with both traditional business intelligence approaches and more advanced data science ones. There are also many differences among the three disciplines that are outlined in the following table.
Decision intelligence | Business intelligence | Data science | |
Primary goal | Augment and improve decision-making processes across the organization. | Analyze and report on historical business data to inform strategy. | Extract insights and create predictive models from complex data sets. |
Approach | Integrates data science, social science, decision theory and managerial science with AI/machine learning technologies. | Focuses on data reporting, visualization and basic analytics. | Applies advanced statistical, computational and machine learning methods. |
Key outputs | Actionable insights, recommendations, predictive models and sometimes automated decisions. | Reports, dashboards and data visualizations. | Statistical models, algorithms, predictive models and analytical insights. |
Target users | Both technical and nontechnical users across the organization, including decision-makers at all levels. | Primarily business users, analysts and executives. | Mainly data scientists, analysts and technical specialists. |
Automation level | Can include automated insight generation and decision-making based on predefined criteria. | Generally, does not include automated decision-making. | Can automate analytical processes but typically not focused on automated decision-making. |
Decision intelligence use cases and examples
Decision intelligence has applications and use cases in various industries. The following list outlines potential uses in some major industries:
- Finance. Credit risk assessment, fraud detection and investment decision support.
- Healthcare. Medical diagnosis support, treatment recommendations and resource allocation.
- Retail. Inventory optimization, pricing strategies and product placement decisions.
- Manufacturing. Production process optimization, quality control and proactive maintenance.
- Energy. Grid management, demand forecasting and maintenance scheduling.
- Transportation. Flight and crew scheduling, fleet maintenance and route optimization.
Decision intelligence systems can also be used for various purposes by different departments in organizations across industries. For example, marketing can use decision intelligence to better understand customer behavior and optimize marketing campaigns. It can help human resources with workforce planning, hiring and employee retention strategies. Supply chain uses include demand forecasting and inventory management.
Best practices for decision intelligence deployments
Deploying decision intelligence systems can significantly enhance an organization's ability to make data-driven decisions. However, successful implementation requires careful planning and adherence to best practices. The following are key best practices to consider when deploying decision intelligence:
- Define clear goals. When starting, a solid best practice is to begin by identifying specific decision-making processes within the organization that could benefit from improvement. Set well-defined objectives for implementing decision intelligence, ensuring they align with overall business strategy. Carefully map out existing decision workflows and approval points to understand where decision intelligence can have the most significant impact.
- Start small. Decision intelligence can be complex and time consuming. Start with a low-risk, well-defined process as a pilot project. Adopt an iterative approach, continuously refining the system based on feedback and results.
- Ensure data quality. Decision intelligence relies on good data. Do a thorough audit of existing data sources to ensure the quality of inputs. Establish data governance practices to maintain data integrity over time.
- Implement feedback loops. While decision intelligence is likely to deliver good results, that might not always be the case. Establish mechanisms to gather both automated and human feedback on decision outcomes. Use this feedback to continuously improve decision models and refine the system's performance.
- Ensure transparency and trust. Being able to trust the decision intelligence process has a lot to do with transparency. Make decision-making processes and rationales easily explainable to build trust among users and stakeholders. Provide clear visibility into how AI and machine learning models arrive at their recommendations or decisions.