citizen data scientist
A citizen data scientist is an individual who does some data science work for an organization but doesn't hold the title of data scientist or have a formal background in advanced analytics, statistics or related disciplines. Citizen data scientists can include business analysts, data-savvy business users, business intelligence (BI) analysts and developers, data engineers and other workers.
In most cases, citizen data scientists don't directly replace professional data scientists. Instead, they augment the work of data scientists, enabling organizations to expand their data analysis initiatives and complete more analytics projects. The two groups may also collaborate on particular data science applications, with expert data scientists supporting and reviewing what the citizen ones do.
Role of a citizen data scientist
While data science work isn't the primary job function of citizen data scientists, they still play a vital role in advanced analytics programs. They typically get involved in data science activities as part of their regular jobs, doing analytics to help drive business decisions, strategies and operations. As part of that process, a citizen data scientist goes beyond basic BI tasks and engages in machine learning, predictive analytics and other higher-level analytics functions.
Essentially, a citizen data scientist qualifies as an analytics power user. Despite not having the same level of expertise and skills that full-fledged data scientists have, citizen data scientists run machine learning algorithms, do data mining, create analytical models, apply statistical analysis techniques and create data visualizations, dashboards and reports to communicate their findings to business executives and workers. They also bring specific knowledge of business issues and information needs to analytics applications.
Why are citizen data scientists important to businesses?
The citizen data scientist has become increasingly important in organizations because of the ongoing shortage of skilled data scientists. The latter typically possess a wide range of analytics and technical skills, and many also have an advanced degree in data science or disciplines like statistics, mathematics and computer science. As a result, data scientists continue to be in short supply, despite the growth of academic programs to educate and train them.
They're also expensive to hire: In the U.S., data scientists commanded average salaries of nearly $145,000 as of October 2022, according to job posting site Indeed. Instead, some data science roles can be filled by employees with other backgrounds who already have a sufficient level of skills or can be trained to do the required analytics work. By using citizen data scientists, organizations can save money, increase their analytics efficiency and make better use of their available data resources.
Like expert data scientists, the citizen variety can specifically help organizations do the following:
- predict how customers will respond to marketing campaigns and promotions;
- identify potential new revenue opportunities based on business trends;
- optimize supply chain management, customer service and other operations;
- analyze financial and reputational risks to avoid possible business problems;
- develop stronger business plans and strategies; and
- gain competitive advantages over business rivals.
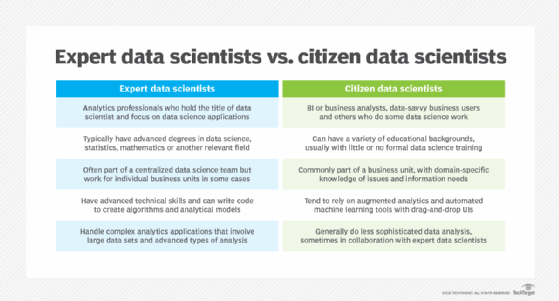
Citizen data scientist vs. expert data scientist
As a whole, citizen and expert data scientists do the same kind of work. Generally speaking, though, a citizen data scientist is likely to be tasked with less sophisticated and more straightforward analytics applications than an expert one in keeping with their different skill levels. Those differences manifest themselves in the following ways:
- An expert data scientist must be proficient in using Python, R, Julia and other programming languages, as well as various data science tools and technologies, to create custom algorithms and complex analytical models. They also must be accomplished in all aspects of the data science process, from data discovery, collection, cleansing and preparation to model selection, validation and deployment, and data visualization and storytelling.
- A citizen data scientist requires similar skills but is more apt to use artificial intelligence (AI) and augmented analytics tools that automate parts of the process for them. For example, automated machine learning (AutoML) software simplifies the development and deployment of machine learning models, with little or no coding necessary. Other tools can be used to help find and prepare relevant data sets and create data visualizations.
From an organizational standpoint, expert data scientists commonly are members of a larger data science team, along with lower-level data analysts, data engineers and other supporting workers. Those teams often are centralized but could be set up in an individual business unit, especially in larger organizations. While citizen data scientists might be assigned to a data science team in a business unit, many of them work independently to address specific business needs. In that case, though, they might have connections to expert data scientists for assistance and collaboration as needed.
Citizen data scientist vs. analytics translator
Analytics translators, also known as data translators, are similar to citizen data scientists in that they typically don't have specialized data analytics training or related technical skills. But the two roles are different. An analytics translator might help start a data analysis process that is carried out by an expert data scientist or a citizen data scientist and then aid in explaining the results to business stakeholders.
Translators are basically liaisons between data scientists and business decision-makers. They identify business needs for information, do initial research and help plan analytics projects to meet those needs. After the data science work is completed, they get involved in communicating the findings and recommending possible actions that business operations could take based on the analytics results. Analytics or data translator is still an emerging role but has become a part of some data science teams.
How to become a citizen data scientist
Various people in an organization can take on the role of a citizen data scientist, but it helps to have a background in the business operation or domain that the analytics work involves. It's also good to have some familiarity with data science and advanced analytics techniques, such as machine learning, data mining, predictive modeling and statistical analysis.
To obtain such skills, prospective citizen data scientists can attend bootcamps and online courses offered by Coursera, Udemy, Kaggle and other technology education platforms. Various universities, technology vendors and industry groups also have data science certification programs that could provide relevant skills and knowledge. Another option is to seek appropriate training within an organization.
In addition, useful information is available through newsletters and YouTube channels that focus on data science. A variety of open data sets are also available to explore and use in practice applications, and Kaggle and other sites run competitions designed to help improve data science skills; some competitions also pay cash prizes to the winners.
The following steps can also help in becoming a citizen data scientist:
- Volunteer for the role when the opportunity presents itself.
- Request access to needed data, along with required user permissions.
- Seek guidance on data governance, privacy and security best practices.
- Increase your overall data literacy to aid in the analytics process.
- Become proficient at data visualization and storytelling, if you aren't already.