Trustworthy AI explained with 12 principles and a framework
To be considered trustworthy, AI systems should meet these 12 principles and employ a four-step framework to ensure the use of AI is ethical, lawful and robust.
Many executives don't realize the potential risks that can accompany working with artificial intelligence. But with AI technologies being empowered to code themselves through new generative AI capabilities and simultaneously having less human oversight, we all must slow down and take steps to bring about trustworthy AI.
What is trustworthy AI?
Characteristics of trustworthy AI systems include the following:
- Reliable performance that consistently delivers accurate and dependable results.
- Robust and accurate performance to handle various situations and data inputs without failing.
- Sound data practices that use high-quality, well-balanced, impartial data with proper permissions for use, security and privacy.
- Prioritized human values that emphasize transparency, safety and fairness in their algorithms.
- Clear explanations for their processes and decisions.
- Feedback loops from diverse users to improve and validate the AI system's performance.
- Continuous monitoring to ensure it functions correctly.
- Contingency plans to address potential issues.
Examples of failed AI
Consider the harm untrustworthy AI can cause:
- Tesla had to recall its Full Self-Driving software, faced damaging media coverage and underwent regulatory investigations after a phantom braking issue endangered customer and public safety by causing cars to erroneously and suddenly stop in traffic.
- Pinterest lost its carefully crafted reputation as one of the last safe places on the internet after a major news investigation revealed it failed to stop pedophiles from using its algorithm to share inappropriate pictures of young girls.
- The National Eating Disorders Association took down its Tessa chatbot -- intended to replace its human call center staff -- over concerns it was providing potentially dangerous advice.
- iTutorGroup, which offers English language classes to students in China, settled an age discrimination hiring lawsuit after its tutor application software allegedly rejected female applicants over the age of 55 and male applicants 60 years of age and older.
- Air Canada had to compensate one of its customers after a chatbot provided them with the wrong information regarding a bereavement fare.
Poorly designed, developed and governed AI systems can lead to systemic societal harms, personal safety risks and environmental issues. Additionally, they can result in corporate repercussions such as negative publicity, class action lawsuits, increased regulatory scrutiny and activist backlash.
The encouraging news is that implementing the following framework to achieve trustworthy AI can minimize these kinds of problems.
Framework to achieve trustworthy AI
The following framework details the four essential steps organizations should follow to integrate trustworthy AI into their technology initiatives. These steps are iterative; for instance, you could reach the final step and realize adjustments are needed or find that the data in Step 3 isn't suitable for certain models, necessitating a return to Step 2.
Step 1. AI strategy, design and planning
This stage of AI development focuses on exploring and selecting a humane AI use case, assessing the risk and impact of the use case, and evaluating how the use case aligns with corporate business goals and existing policies relevant to the proposed AI system's use. Design and technical requirements should be reviewed, as well as ensuring enough budget, time and skilled talent will be available without cutting corners that could unintentionally cause ethics issues down the line. Think of cheap-but-fast ill-gotten data or uncertified programmer mistakes as examples.
This article is part of
What is enterprise AI? A complete guide for businesses
Step 2. Data
Reliable data is a cornerstone of AI technology. Organizations should develop a comprehensive data strategy that details the intended data sources, methods to assess quality and bias, data management, and privacy and security policies for data access and compliance. Documenting all data sources and splits used for training and validation is essential for future AI audits; this can also be done in conjunction with Step 3.
Step 3. Algorithm
This step sets the logic and instructions for building the AI decisioning capabilities. A model needs to be selected for the use case. Data sets need to be explored, transformed and munged, and pipelines have to be properly connected and validated. The model needs to be built, trained and tested to verify trustworthiness. Accuracy and bias thresholds should be applied, and the initial model outputs and results should be benchmarked and documented, as this helps with model and data drift later.
Step 4. Deployment and governance
When the AI system or its API feed has been tested and released into production, it must be managed and monitored for model and data drift, inappropriate uses by bad actors, safety issues that come up through use, hacking activities and any cybersecurity breaches. An incident response plan should be put in place in case something goes wrong with the AI, including malfunctioning, failing to operate or causing harm in any way. Finally, employees should receive change management training to keep pace with any changes to the AI system or its feeds.
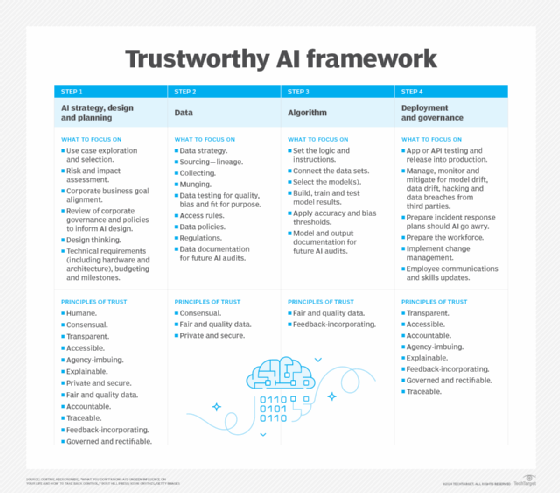
12 principles of trustworthy AI and how to start
The principles listed below come from my book, What You Don't Know: AI's Unseen Influence on Your Life and How to Take Back Control. They are the result of my experiences working with Fortune 100 AI teams to successfully scale and operationalize their AI initiatives.
Each principle listed below is accompanied by actions AI leaders need to take to help build trust in their AI systems. These principles are directly mapped to each step of the trustworthy AI framework graphic above.
- Humane. Evaluate if the use of AI serves humanity or could cause more harm than good to society, the environment and an individual's pursuit of life, freedom and happiness. Assess the likelihood that the AI could be used by bad actors in unintended and harmful ways, and put safeguards in place to deter such bad actors. Conduct a risk and impact assessment to understand if the AI use case has a high, medium or low risk of causing serious harm.
- Consensual. Seek permission from individuals, business partners and third parties to use their data for AI under development.
- Transparent. Inform any individual who might be affected by the developed AI -- in language understandable to them -- about AI algorithms that might influence decisions about their life, livelihood or happiness and the impact they could have.
- Accessible. Document the decisions the AI made regarding any individuals whose data was used, and make the information available to them online or in an app so that they can check the results. An example of this is MyFICO.com, where a person can check their credit score and understand what might be affecting their personal score.
- Agency-imbuing. Set up and communicate an appeals program for any individuals who feel the algorithm's recommendations or source data about them might be incorrect.
- Explainable. Explain the AI's decisions and sources in plain wording.
- Private and secure. Keep all information used to develop, train, deploy, manage and govern the AI system private and secure. This includes when third-party vendors and business partners are involved.
- Fair and quality data. Ensure the data used to train and develop the AI system is based on sound data standards and has been thoroughly analyzed and adjusted for biases, bad data and missing data. Use sound, appropriate proxies for data that was unobtainable or missing.
- Accountable. Declare, train and communicate the people responsible for fixing the AI system if it malfunctions. Update corporate policies and guidelines to ensure there is an incident response plan for any emergency situations that might occur.
- Traceable. Set up monitoring tools, processes and employees to communicate which part of an AI system went wrong and when it happened. So many bad AI incidents result in companies that cannot explain how and when something went wrong. This reaction does not create trust.
- Feedback-incorporating. Provide ways for users, affected people and experts to offer input into the AI system's ongoing learning. Where possible, ensure diversity of feedback to help mitigate any bias that could creep into the system upon release and into the future.
- Governed and rectifiable. Implement model drift and data drift monitoring tools and processes, as well as designated people, to detect if the AI system fails or becomes unsafe, biased or corrupt. Create an incident response plan to rectify emergency situations to ensure safety and liberties are guaranteed while the AI is down or malfunctioning.
Key benefits of trustworthy artificial intelligence
AI is a huge investment for any organization, and it can be a competitive differentiator or perhaps even a major cost-efficiency play. Either way, the technology needs to be trustworthy to reap the benefits of AI, such as the following:
- Improved brand reputation.
- Increased market competitiveness.
- Greater AI return on investment.
- Increased company and client AI adoption rates.
- Decreased risk of class action lawsuits and major harms.
- Improved regulatory readiness.
- Decreased risk of a major harm-causing incident.
Why we need trustworthy AI systems
At a macro level, AI is the bedrock of systems that can affect areas such as income-based inequality via the use of AI in financial credit scoring and hiring systems; the environment due to generative AI's outsized carbon footprint and water cooling requirements; and diplomatic tensions between nations -- for example, the suspected Chinese surveillance of U.S. citizens via TikTok.
At the micro level, AI affects individuals in everything from landing a job to planning retirement, securing home loans, job and driver safety, health diagnoses and treatment coverage, arrests and police treatment, political propaganda and fake news, conspiracy theories, and even children's mental health and online safety.
We need trustworthy AI to ensure the following:
- Physical safety. Examples include autonomous vehicles, robotic manufacturing equipment and virtually any human-machine interactions where AI is deployed in split-second decision-making.
- Health. Examples include AI-assisted robotic surgeries, AI-assisted diagnostic imaging tools, clinical decision support, generative AI-based chatbots and health insurance claims systems.
- Ability to secure necessities. Examples of AI systems affecting a person's ability to obtain food and housing, for example, are hiring systems, financial credit scoring systems and home loan systems.
- Rights and liberties. AI systems must be monitored to prevent predictive policing systems, facial matching programs and judicial recidivism prediction systems.
- Democracy. AI systems can be used to violate democratic principles including overreaching AI surveillance programs; creating divisive fake news with generative AI; and propaganda, conspiracy theories and terrorist recruitment spread via AI recommendation engines.
Editor's note: This article was updated in September 2024 to improve the reader experience and provide recent examples of untrustworthy AI.
Cortnie Abercrombie is the CEO and founder of AI ethics nonprofit AI Truth and author of What You Don't Know: AI's Unseen Influence on Your Life and How to Take Back Control. Reach her at [email protected].