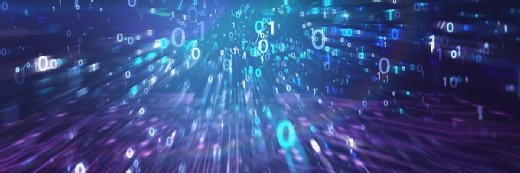
Getty Images
Data quality fuels analytics, AI
Data quality is tied to organizations' ability to gain actionable data from analytics and AI processes, and orgs that implement data quality tools can make faster decisions.
Data quality has profound significance in shaping the landscape of analytics, AI and generative AI.
Data has become the new currency, propelling industries toward transformative innovations in analytics, AI and generative AI. However, amidst the discordance of data, the critical importance of data quality stands as an unwavering beacon.
In its May 2023 survey of IT decision-makers called "Data Platforms: The Path to Achieving Data-driven Empowerment," TechTarget's Enterprise Strategy Group asked, "What is your organization's most important expected outcome from implementing data quality tools or services?" What we found, as seen in the chart, is that organizations are heavily focused on implementing data quality tools and practices to drive faster and more informed decision-making for internal stakeholders at lines of business as well as for end users. Data quality directly correlates to actionable, trusted data from analytics and AI processes.
The foundation of analytics and AI. At the heart of every analytical endeavor and AI-driven application lies data. The quality of this data profoundly impacts the outcomes of the processes it fuels. Data quality encompasses a range of factors, including accuracy, completeness, consistency, reliability and timeliness. When data quality is compromised, the accuracy of insights and predictions derived from it is at risk, leading to erroneous conclusions and misguided decisions.
Precision in analytics. The essence of analytics lies in extracting valuable insights from data. High-quality data ensures that the insights derived are reliable and accurate, enabling organizations to make well-informed strategic decisions. Inaccurate or incomplete data, on the other hand, can lead to misguided conclusions, causing a ripple effect across the decision-making process.
Efficacy in AI. AI thrives on data, relying on historical and real-time information to build predictive models and make intelligent decisions. Quality data serves as the lifeblood of AI, enhancing the performance of algorithms and enabling them to produce dependable forecasts, recommendations and classifications. Clean data empowers AI to learn from genuine patterns, amplifying its predictive prowess.
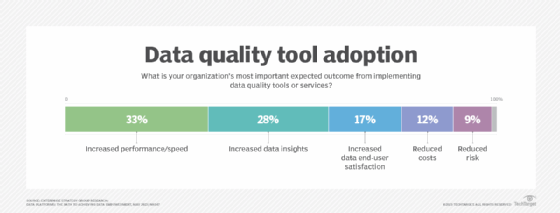
Generative AI and creative output. In the realm of generative AI, where machines produce creative content such as art, music and text, data quality assumes a novel significance. High-quality input data ensures that the generated output is coherent, meaningful and aligned with the desired creative direction. Without data quality, generative AI risks producing nonsensical or disjointed results that lack artistic merit or practical value.
Ethical considerations. Data quality is integral to ethical AI development. Biased or skewed data can perpetuate biases within AI systems, leading to unjust outcomes and reinforcing societal inequalities. Ensuring data quality is paramount for fostering fair and unbiased AI applications that respect ethical considerations and uphold social values.
Data quality and the AI lifecycle. The AI lifecycle encompasses data collection, preprocessing, model training, validation and deployment. At each juncture, data quality serves as the lodestar, guiding AI endeavors toward success:
- Data collection and preprocessing. The foundation of AI lies in data collection and preprocessing. Quality data harvested at this stage enhances the reliability of subsequent analyses and model training. Ensuring that data is clean, consistent and representative minimizes the risk of skewed or misleading results.
- Model training and validation. During model training, data quality impacts the learning process. High-quality data ensures that models capture accurate patterns and relationships, leading to more accurate predictions and generalizations. Validation using quality data assures the model's robustness and effectiveness.
- Deployment and real-world impact. In the real-world deployment of AI solutions, data quality guarantees that the insights and recommendations provided are actionable and reliable. Stakeholders and decision-makers can confidently rely on AI-driven insights to shape business strategies and operational decisions.
Elevating generative AI with data quality: Generative AI introduces a novel paradigm by enabling machines to create original content. Data quality becomes a critical factor in curating input data that stimulates meaningful and coherent creative output. The artistic integrity and real-world applicability of generative AI creations hinge on the caliber of data that fuels the generative process.
Desired outcomes
Enterprise Strategy Group research showed four key desired outcomes from organizations implementing data quality tools, services and practices to enhance analytics, AI and generative AI. They included the following:
- Accurate insights and informed decisions. Data quality ensures that the insights drawn from analytics and AI are accurate and reliable. Flawed or inconsistent data can mislead organizations, leading them to base decisions on faulty information. Quality data supports a higher level of confidence in analysis and predictions, enabling businesses to make informed decisions that drive growth, efficiency and innovation.
- Enhanced predictive modeling. AI algorithms and machine learning models rely heavily on historical and real-time data to make predictions. High-quality data enhances the efficacy of these models, resulting in more accurate forecasts and recommendations. Clean, consistent data ensures that models learn from reliable patterns, making them adept at handling complex scenarios and improving their predictive accuracy.
- Effective problem-solving. Data quality directly impacts an organization's ability to identify and solve problems. Analytics and AI can uncover hidden insights, patterns and root causes of issues, enabling proactive decision-making. However, if the underlying data is inaccurate or incomplete, the problem-solving process can become convoluted, delaying resolutions and hampering progress.
- Personalized customer experiences. For businesses striving to offer personalized experiences to customers, data quality is paramount. AI-driven personalization relies on accurate customer data to understand preferences, behaviors and buying patterns. Clean data ensures that recommendations and interactions align with customer expectations, leading to higher satisfaction and loyalty.
Data quality isn't a mere checkbox; it's the linchpin that holds the realms of analytics, AI and generative AI together. Its influence permeates every facet of these domains, orchestrating the symphony of insights, predictions and creations that drive innovation and transformation. In an era defined by the relentless pursuit of data-driven excellence, organizations that prioritize data quality pave the way for accurate analytics, intelligent AI and inspired generative AI -- ultimately shaping a future that's as visionary as it is data-driven.
Enterprise Strategy Group is a division of TechTarget. Its analysts have business relationships with technology vendors.