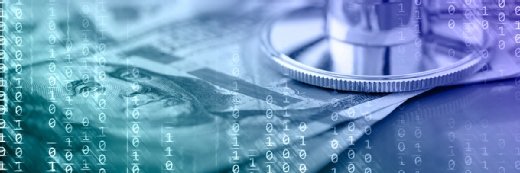
Viorika/istock via Getty Images
Machine learning algorithm improved risk adjustment models, study finds
The machine learning algorithm helped avoid significant underpayments for people with at least one rare diagnosis.
A Diagnostic Cost Group (DCG) machine learning algorithm succeeded in generating risk adjustment models and predicted healthcare spending better than the current HHS hierarchical condition category (HCC) model, a study found.
Medicare Advantage, Affordable Care Act (ACA) marketplaces, Medicare Part D benefit programs, and state Medicaid managed care programs all use diagnosis-based risk adjustment formulas for health plan capitation, performance assessment, severity adjustment, and value-based incentive payments and penalties.
CMS and HHS use HCC models for Medicare and ACA marketplace enrollees, even though there have been increases in the diagnostic specificity of claims data since 2015. Some stakeholders are concerned that HCC models do not take advantage of stronger diagnostic information, larger datasets, faster computers, and improved machine learning models. In addition, HCC models may be vulnerable to diagnostic upcoding, gaming, and fraud.
Researchers used clinical judgment to organize diagnostic items (DXIs) into DCG groups and hierarchies to be used as model building blocks. Then, they developed, implemented, and evaluated a novel machine learning algorithm to automate and empirically organize the groups and hierarchies into clusters for variable selection and prediction.
The study included 35 million commercial health insurance enrollees 64 years and younger. The DCG model had a coefficient of determination or R2 of 0.535, performing better than using Charlson Comorbidity Index variables as predictors or using diagnostic categories from the HHS HCC model (0.428)
The DCG model reduced the number of parameters needed by 80 percent and reduced model sensitivity to upcoding compared with simple additive models. The model also reliably priced rare diseases, keeping mean predicted spending within 12 percent or actual spending for the 3 percent of people who have at least one diagnosis as rare as one in one million. In contrast, the HHS HCC model underpaid this group by 33 percent.
The DCG algorithm had greater predictive power and was able to ignore diagnoses due to vagueness, inconsistent use, or gameability. In addition, the model’s hierarchy restrictions ignored lower-ranked diagnoses when in the presence of clinically related, higher-ranked diagnoses, and the algorithm’s final models avoided negative predictions.
The study findings suggest that CMS and HHS could improve risk adjustment models by automating DXI clustering within clinically specified hierarchies.