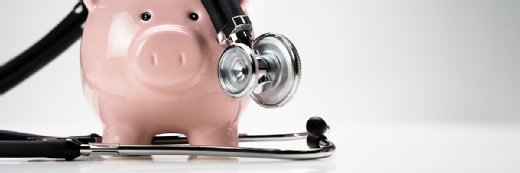
AndreyPopov/istock via Getty Ima
Medical Coding is the Next Stop for Artificial Intelligence in Healthcare
Industry leaders agree that artificial intelligence in healthcare is taking off; medical coding and billing is the latest use case for ER staffing company TECHealth.
To a layperson, medical codes look like a different language, and in a way they are. Medical coding is a highly complex process in healthcare in which clinicians and revenue cycle staff work together to translate clinical encounters into billable codes for not only reimbursement but other performance tracking efforts. The codes that ultimately end on claims tell the story of a patient’s encounter.
Understanding a clinical encounter is extremely important for patient care and for keeping the doors open. However, in a world of ever-changing payer rules and documentation requirements, medical coding is perhaps more complex than ever.
“Something that is challenging for us is getting payment in a timely fashion so that we can actually make payroll to make sure doctors and APPs [advanced practice practitioners] are paid correctly,” explains Eric Wilke, MD, Eric Wilke, an emergency medicine doctor and chief operations officer at TECHealth, an ER physician staffing company.
Medical coding can get in the way of timely reimbursement. Payers may reject or deny claims because of medical coding errors or missteps. In fact, a survey of hospital executives found that about a third cite coding as their top concern when it comes to denials and denials prevention.
With denial rates on the rise, optimizing medical coding to prevent claims denials is critical. Healthcare organizations must identify the right codes for services provided during a clinical encounter while adhering to payer requirements. Organizations must ensure they are not leaving money on the table by neglecting to code a service or applying the appropriate severity level or modifier.
Organizations are also trying to implement medical coding best practices with fewer coders and revenue cycle staff.
“We’ve experienced hiring challenges for billing and coding positions, especially in the wake of COVID-19,” Wilke says. “We had extended interviews to several people, and they never even bothered to show up.”
The “Great Resignation”—a term used to describe high levels of turnover during and in the aftermath of the COVID-19 pandemic—has hit healthcare particularly hard. Provider organizations have especially faced shortages of qualified revenue cycle talent, with entry-level roles taking an average of 84 days and over $2,000 to fill. Mid-level revenue cycle positions, which require six to ten years of experience, are taking an average of 153 days and over $3,500, survey results also show.
Many healthcare organizations choose to offshore medical coding to combat domestic staffing shortages and the high costs of filling open roles. But for organizations like TECHealth, “all of the work we were doing was domestic,” Wilke states.
Unfortunately, Google Translate does not recognize medical codes as a language. However, TECHealth found a “bit of an equalizer in that standpoint.”
Tapping into AI
Artificial intelligence (AI) has taken healthcare by storm. The predictive analytics technology is revolutionizing the way certain providers deliver care. For example, Mayo Clinic recently found that AI can help detect atrial fibrillation, while Google Health is tapping the technology to detect cancer.
AI is being leveraged by researchers, providers, and tech companies alike to diagnose some of the most common and deadly diseases out there. However, the technology can also serve a purpose on the administrative side of healthcare.
Medical coding is ripe for AI technology, according to Wilke.
“Anything image or text-centric is a great opportunity for AI,” the healthcare leader explains. “So, yes, pathology, radiology, and dermatology are all areas of opportunity, but so is analyzing charts for billing and coding.”
AI can help organizations overcome the top challenges of medical billing and coding.
“We want something that can process [payments] quickly, so get them to the clearinghouse faster. The faster that works through the system, the better,” Wilke explains.
Tapping AI technology for medical billing and coding means a large portion of TECHealth’s claims were being worked through their IT systems. The technology was able to comb through upwards of 80 percent of claims with limited or without any human interaction. Additionally, the technology was able to process tens of thousands of medical charts in a matter of days to ensure proper coding.
With a kick-out ratio of under 20 percent, Wilke describes AI technology for medical billing and coding as “a bit of an equalizer” when it comes to the workforce obstacles most healthcare organizations are currently facing, according to Wilke.
“[The technology] is not dependent on the human workforce, which is currently limited,” Wilke elaborates.
The smaller medical billing and coding team was able to work on more complex claims to recoup money from claim denials and to prevent future money-leaking missteps. Most of the low-hanging fruit was already taken care of by the organization’s IT systems.
Growing pains
While medical billing and coding are ripe for AI innovation, as evidenced by TECHealth’s success with the technology, implementation can come with some growing pains for healthcare organizations. The number one lesson learned, according to Wilke, is having quality data.
“If I could go back and redo [implementation], one of the things I would’ve redone is analyzing our coding team to make sure they were using appropriate codes because anyone using an AI engine has to use historical data so they can build the AI’s prediction model.”
“If you don’t give the [AI] the most accurate information upfront, then you don’t always get the most correct information on the backside,” Wilke stresses.
Garbage in, garbage out. That has been a major lesson for healthcare with the advent of analytics. The adage is especially true for predictive analytics models that rely on the data you feed the system to make predictions and workflows.
Healthcare organizations need enough data points or sets to ensure accurate prediction models since using a tiny fraction of your information does not provide the power analytics technologies need to be successful. That data also needs to be clean and accurate, Wilke indicates.
“I would have found somebody that coded very well, so at an appropriate level, and had those historical codes be used to train the AI,” Wilkes states.”If you’re moving to the AI side of the world, you need to analyze your data and the [evaluation and management] levels assigned. Make sure that your audit shows that coding is being done correctly, then pass that forward to the AI training engine.”
TECHealth also relied on its vendor at the time, Fathom, to get the AI prediction models just right for its medical billing and coding needs, Wilke stresses. The organization decided to go with that vendor mainly because of its “fairly robust history” of applying predictive analytics to medical coding and billing.
“They were nimble, easy to work with, and pretty aggressive and forward with making sure things got connected as fast as possible. Their technical team and our technical team worked really well together,” Wilke says.
Having a strategic partner parse through historical data and properly train models was key to TECHealth’s success with medical billing and coding at that time. And despite some pains, AI in healthcare is only growing, especially in the realm of medical billing and coding.
“AI is going to become an essential tool in this arena. It already has a pretty significant role, and it’s only going to get bigger,” Wilke states.