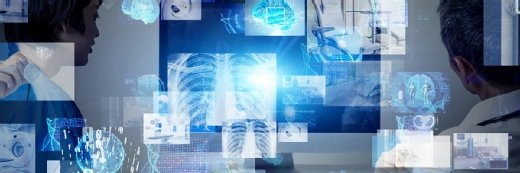
metamorworks/istock via Getty Im
Machine Learning Moves the Needle on Personalized Antidepressants
NIMH-funded research suggests a machine learning algorithm can discern the resting-state electroencephalography of participants who fared well on the antidepressant sertraline.
A machine learning algorithm can predict whether an individual is likely to benefit from sertraline treatment using a neural signature, findings published Monday in Nature Biotechnology suggest.
Researchers developed the SELSER (Sparse EEG Latent SpacE Regression) algorithm to see if specific brainwaves, as measured by resting-state electroencephalography, can be used to glean insights into antidepressant treatment response. SELSER demonstrated in the study that it can reliably predict an individual response to sertraline based on the recorded alpha waves.
The study stems from a longtime effort funded by the NIMH for the creation of biologically based approaches like brain imaging that help move the needle on personalized depression treatment.
"This study takes previous research showing that we can predict who benefits from an antidepressant and actually brings it to the point of practical utility," said senior author Amit Etkin, a professor of psychiatry and behavioral sciences at Stanford University. “I will be surprised if this isn't used by clinicians within the next five years.” Efforts are already underway.
Funded by the National Institute of Mental Health of the National Institutes of Health, the research used the data from an NIMH-funded randomized trial of sertraline (Zoloft). The drug is a selective serotonin reuptake inhibitor. It typically works in about one-third of those who take it.
The research underscored machine learning’s potential to advance a personalized approach to depression treatment. NIH said efforts already underway will look to further replicate the study funding to assess the value of SELSER as a diagnostic tool in large, independent samples.
The study monitored changes in upwards of 300 people aged 18 to 65 with depression for eight weeks, from the time they began taking sertraline or a placebo, using a depression rating scale. Participants had previously tried sertraline but were not on such drugs at the start of the study.
SELSER was able to identify a specific pattern of brain activity associated with a higher likelihood of an individual patient benefitting from sertraline by comparing the EEG recordings of participants who responded well to the drug to those from participants who did not.
Sertraline’s ability to improve depressive symptoms was questioned, with last December’s findings from a large placebo-controlled clinical trial in the UK. “It appears that people taking the drug are feeling less anxious, so they feel better overall, even if their depressive symptoms were less affected,” lead author, Gemma Lewis, said at the time. “We hope that we have cast new light on how antidepressants work, as they may be primarily affecting anxiety symptoms such as nervousness, worry and tension, and taking longer to affect depressive symptoms.”
Only 41 percent of overall participants responded well to sertraline in the SELSER study. Yet the machine-learning algorithm was able to pinpoint a subset (76 percent) in predicting that those who saw benefits from taking sertraline would do so, according to the study findings.
Senior author and a provider of psychiatry at the University of Texas-Southwestern, Madhukar Trivedi, cited one of his previous studies that showed just 30 percent of patients with depression had seen any remission of their symptoms after first treatment with an antidepressant.
Efficacy of antidepressants relative to placebo is modest partly because of the biologically heterogeneous conditions that a major depression diagnosis involves, study authors noted. Yet analyses suggest that SELSER predictions could extend to clinical outcomes beyond sertraline.
“While work remains before the findings in our study are ready for routine clinical use, the fact that EEG is a low-cost and accessible tool makes the translation from research to clinical practice more possible in the near term,” Etkin further noted. “I hope our findings are part of a tipping point in the field with respect to the impact of machine learning and objective testing.”