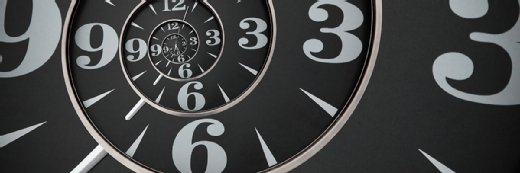
Getty Images
Medical AI Device Evaluation Process Could Mask Vulnerabilities
A majority of data test sets from FDA-approved medical AI devices were collected and evaluated before device deployment rather than concurrently, which can mask vulnerabilities.
A recent Nature study found that evaluating the performance of FDA-approved medical artificial intelligence (AI) devices in multiple clinical trial sites is crucial to ensuring that the devices perform well across representative populations
In the overview, researchers found that data test sets from 126 out of 130 FDA-approved medical AI devices were collected and evaluated before device deployment (retrospective), rather than concurrently with device deployment (prospective).
And 93 of the 130 devices did not have a publicly-reported, multi-site assessment. Notably, none of the 54 high-risk devices studied were evaluated by prospective studies.
The path to safe and robust clinical AI requires that important regulatory questions be addressed. But currently, there are no established best practices for evaluating these devices.
Therefore, FDA called for improvement of test data quality to improve trust and transparency, monitor algorithmic performance, and bring clinicians into the loop.
To understand the extent to which these concerns are addressed in practice, researchers looked at medical AI devices approved by FDA between January 2015 and December 2020 and analyzed how these devices were evaluated before approval.
Specifically, they looked at patients enrolled in evaluation studies, the number of sites used in the evaluation, if the test data was collected prospectively or retrospectively, and whether stratified performance by disease subtypes was reported.
“Evaluating the performance of AI devices in multiple clinical sites is important for ensuring that the algorithms perform well across representative populations. Encouraging prospective studies with comparison to standard of care reduces the risk of harmful overfitting and more accurately captures true clinical outcomes,” researchers said in the study.
“Post-market surveillance of AI devices is also needed for understanding and measurement of unintended outcomes and biases that are not detected in prospective, multi-center trial,” they continued.
Separate from the comprehensive overview, researchers conducted a case study of collapsed lung, or pneumothorax, triage devices. Currently, there are four FDA-cleared medical devices for the triage of X-ray images of pneumothorax, as well as multiple available chest X-ray datasets that include pneumothorax as a condition.
Researchers used deep-learning models to classify chest conditions from patients at various hospital sites across the US, including the National Institutes of Health Clinical Center (NIH), Stanford Health Care (SHC), and Beth Israel Deaconess Medical Center (BIDMC).
In the case study, the models achieved “good performance” on NIH patients, but performed much worse on BIDMC test patients and SHC test patients.
Findings from both the overview and case study suggest that a “substantial proportion” of FDA-approved devices may have been evaluated only at a small number of sites, which limits geographic diversity.
“Across the board, we found substantial drop-offs in model performance when the models were evaluated on a different site. Evaluating deep-learning models at a single-site along, can mask weaknesses in the models and lead to worse performance across sites,” researchers said.
Over the past five years, the number of approvals for AI devices has increased rapidly, with over 75 percent of approvals coming in the past year. But the proportion of approvals with multi-site evaluation and reported sample size has been stagnant during the same time period.
Multi-site evaluations are important for multiple reasons, researchers noted.
First, multi-site evaluations can help experts understand algorithmic bias and reliability.
Additionally, these evaluations can help in accounting for variations in the equipment used, technician standards, image-storage formats, demographic makeup, and disease prevalence.