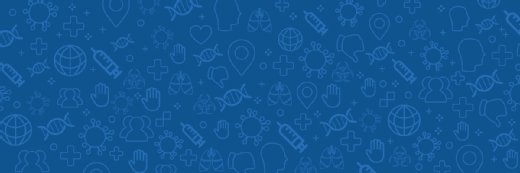
Leveraging Predictive Analytics for Effective Disease Management
Predictive analytics transforms disease management by enabling early detection, personalized treatment, and optimized resource allocation in healthcare.
Predictive analytics, a powerful tool fueled by advanced technologies and data-driven insights, is transforming how healthcare providers approach disease management. By analyzing vast amounts of patient data, predictive analytics enables early detection, accurate diagnosis, personalized treatment plans, and optimized healthcare resource allocation.
Predictive Analytics
Predictive analytics uses statistical algorithms and machine learning (ML) techniques to analyze historical data and predict future outcomes. In the context of disease management, predictive analytics leverages patient data, including medical records, genetic information, lifestyle factors, and environmental data, to generate actionable insights and predictions. By identifying patterns and correlations within the data, predictive analytics empowers healthcare professionals to anticipate disease progression, identify high-risk patients, and make informed decisions for optimized treatment plans.
Early Detection and Prevention
One of the key advantages of predictive analytics in disease management is its ability to facilitate early detection and prevention. By analyzing patient data, predictive models can identify individuals at risk of developing certain diseases, even before symptoms manifest. This early detection allows healthcare providers to intervene promptly, providing personalized preventive measures and reducing the likelihood of disease progression.
For example, predictive analytics can identify individuals at high risk of developing diabetes by analyzing various factors such as age, family history, lifestyle choices, and biomarkers. By leveraging statistical models and ML algorithms, predictive analytics can assess the probability of future diabetes onset, enabling healthcare providers to implement targeted preventive measures and interventions for at-risk individuals.
Personalized Treatment Plans
Predictive analytics plays a vital role in tailoring treatment plans to individual patients. By analyzing patient data and comparing it to vast medical knowledge databases, predictive models can recommend the most effective treatment options for specific conditions. This personalized approach considers factors such as genetic predispositions, medication responses, and lifestyle choices, ensuring that patients receive treatments that are most likely to yield positive outcomes. For instance, predictive analytics can be used in cancer care to determine the optimal chemotherapy regimen based on a patient's genetic profile, minimizing adverse effects, and maximizing treatment efficacy.
Resource Allocation and Operational Efficiency
Predictive analytics plays a crucial role in healthcare resource allocation and operational efficiency. Predictive models enable healthcare organizations to optimize resource allocation, staffing levels, and patient flow by analyzing historical data on patient admissions, bed occupancy rates, and resource utilization.
For instance, predictive analytics can accurately forecast future admission rates by examining patterns in historical admission data. This empowers healthcare providers to allocate resources, such as staff, beds, and equipment, based on anticipated demand. As a result, waste is minimized, and resources are readily available when needed.
Moreover, predictive analytics is valuable in predicting disease outbreaks by analyzing disease prevalence, weather patterns, and population movement data. This proactive approach to resource allocation allows healthcare providers to effectively prepare for epidemics and take preventive measures to curb their spread.
Another area where predictive analytics excels is in anticipating equipment failures. Predictive models can identify equipment that is likely to fail by analyzing equipment usage and maintenance history data. This enables healthcare providers to schedule maintenance or replacement, preventing disruptions in care and potentially saving lives.
Challenges and Considerations
Predictive analytics in healthcare faces several challenges and considerations. These include issues related to data quality and availability, data privacy and security, model interpretability, ethical concerns, integration and implementation, and validation and evaluation.
Data Quality and Availability
Predictive analytics relies on historical data to train models and make accurate predictions. However, healthcare data may suffer from issues such as missing data, data sparsity, and data irregularity. These data quality issues can affect the performance and accuracy of predictive models.
Data Privacy and Security
Healthcare data is highly sensitive and subject to privacy regulations like the Health Insurance Portability and Accountability Act (HIPAA). Implementing predictive analytics in healthcare requires careful consideration of data privacy and security to ensure patient confidentiality and compliance with legal requirements.
Model Interpretability
Predictive models in healthcare often utilize complex algorithms such as machine learning. While these models can provide accurate predictions, they may lack interpretability, making it challenging for healthcare professionals to understand and trust the model's recommendations. The interpretability of predictive models is crucial in healthcare, as it helps clinicians make informed decisions and understand the reasoning behind the predictions.
Ethical Considerations
Predictive analytics in healthcare raises ethical considerations, particularly regarding the potential for bias and discrimination. Biased training data or biased algorithms can lead to unfair predictions or unequal treatment of patients. It is important to ensure that predictive models are fair, unbiased, and do not perpetuate existing healthcare disparities.
Integration and Implementation
Integrating predictive analytics into healthcare systems and workflows can be complex. Healthcare organizations must consider compatibility and interoperability issues between different data sources and systems. Additionally, there may be challenges in effectively implementing predictive models into clinical practice and ensuring their adoption by healthcare professionals.
Validation and Evaluation
Validating and evaluating predictive models in healthcare is crucial to assessing their performance and reliability. Healthcare organizations must establish robust validation processes and evaluate the predictive models' accuracy, sensitivity, specificity, and other relevant metrics. Continuous monitoring and refinement of the models are necessary to ensure their effectiveness over time.
Addressing these challenges and considerations is essential to harness the full potential of predictive analytics in healthcare. By overcoming these obstacles, healthcare organizations can leverage predictive analytics to improve patient outcomes, optimize resource allocation, and enhance decision-making processes in healthcare delivery.
As the healthcare industry continues to embrace digital transformation, predictive analytics will undoubtedly play a pivotal role in shaping the future of disease management.