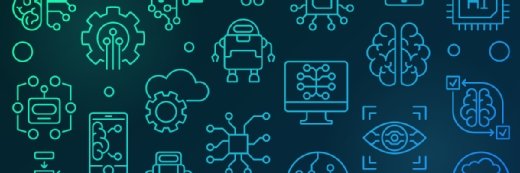
How AI is revolutionizing clinical trials, drug development
AI is transforming clinical trials by enhancing patient recruitment, site selection and data analysis. These advancements accelerate drug development while reducing costs.
Clinical trials are critical to drug development. However, they’re often slowed by inefficiencies, lengthy timelines, high costs and challenges in patient recruitment and data analysis. Artificial intelligence is changing that. By streamlining trial design, optimizing patient matching and accelerating data analysis, AI is helping companies bring treatments to market faster and more cost-effectively.
Optimizing clinical trials
According to a study by the Tufts Center for the Study of Drug Development, the average cost of bringing a new drug to market is $2.6 billion, with clinical trials accounting for a significant portion of that expense.
"The time it takes to recruit the right patient for the right treatment, the laborious data collection and the subsequent manual data analysis are major bottlenecks," Mohan Uttawar, co-founder and CEO of OneCell, said in an interview.
AI is addressing these challenges by streamlining site selection, patient recruitment and data analysis. By leveraging historical data, AI can more efficiently identify appropriate sites and patients, reducing the time and cost associated with these critical steps.
"Using AI has several major advantages -- from site selection to the delivery of results," Uttawar continued. "The process is more streamlined now, with past data helping to identify appropriate sites and patients while leveraging the vast amount of available data to accurately select and eliminate sites and patients for clinical trials."
Uttawar shared one notable example of AI’s impact is Exscientia, a company that used AI to design a cancer immunotherapy molecule in under 12 months, a process that traditionally takes four to five years. This accelerated timeline demonstrates how AI can rapidly advance promising therapies from concept to patient testing.
Enhancing drug development
AI is not only optimizing clinical trials but also revolutionizing the broader drug development process. One of the most significant contributions of AI in this space is its ability to refine trial protocols and optimize site selection.
"A major paradigm shift has erupted with AI as these tools efficiently optimize trial design and execution by leveraging access to large data and streamlining site selection and patient recruitment," Uttawar noted.
Machine learning is also playing a crucial role in biomarker discovery and patient stratification, which are essential for developing targeted therapies. By analyzing large datasets, AI can identify patterns and insights that would be impossible for humans to detect manually.
"Availability and accessibility to large data sets from machine learning are instrumental in developing robust algorithms that garner key insights into patient stratification and corresponding targeted therapies," Uttawar explained.
The cost savings associated with AI-driven drug development are substantial. Traditional in silico models, which use computational methods to analyze biological data, can take five to six years to complete. In contrast, AI-powered optimizations can reduce this timeline to as little as five to six months, significantly lowering costs.
Regulatory and ethical considerations
While the benefits of AI in clinical trials are clear, there are also regulatory and ethical challenges that must be addressed. One of the primary concerns is the robustness of the data used to develop AI algorithms.
"The regulatory challenges for AI-driven clinical trials primarily center around questions about the robustness of data used to develop algorithms, as well as their validation and comparison with existing software," Uttawar highlighted.
To address these challenges, regulatory agencies like the FDA are developing frameworks for validating AI-driven data and algorithms.
"Potentially, the FDA would create an AI-based validation framework for the data, with guidelines for future algorithm development and insights," Uttawar suggested.
Data privacy and security are also critical considerations, especially given the vast datasets required to train AI models. Compliance with regulations is essential to ensure data privacy and security.
"Defined regulations including HIPAA, ISO 13485, GDPR, and 21CFR Part 820 are key in determining security, compliance and data privacy, making it mandatory for AI models to be compliant," Uttawar mentioned.
AI also has the potential to promote greater diversity in clinical trials and reduce biases in patient selection. By analyzing data objectively, AI can recruit patients from diverse populations more efficiently than traditional methods.
"AI helps analyze data objectively, recruiting patients from diverse populations in a time-sensitive manner," Uttawar added. “AI reviews all selection criteria and, based on vast data, efficiently provides data-driven insights and decisions about patient composition for clinical trials."
Trends and predictions
The adoption of AI in clinical trials and drug development is expected to grow exponentially in the coming years.
"In the next five years, almost 80-90% of all clinical trials are expected to use AI," Uttawar predicted. "AI will be involved in trial design, data analysis and even regulatory submissions."
Emerging AI applications, such as OneCell’s AI-based toolkit for predicting genomic signatures from H&E Whole Slide Images, are particularly promising. This technology enables hospitals and other facilities to analyze high-resolution images and identify potential cancer patients who may benefit from specific treatments.
"This toolkit captures high-resolution H&E slide images at 40X resolution and then analyzes them using AI-driven algorithms to detect morphological changes," Uttawar detailed. "This approach is easily accessible to hospitals and other facilities, enabling image analysis and risk stratification to inform physicians' targeted treatment decisions."
For AI to reach its full potential in drug development, greater collaboration between small AI-focused companies and the pharmaceutical industry is needed. Additionally, regulatory frameworks must evolve to support the validation and standardization of AI-driven insights.
"Culturally, greater collaboration is needed between small companies developing AI-based products and tests and the pharmaceutical industry," Uttawar insisted. "From a regulatory perspective, the FDA needs to create the framework to validate data obtained through AI, as well as provide clear guidelines for future AI-driven algorithms and insights to ensure consistency with existing standards."
As Uttawar mentioned, AI is transforming drug development research and clinical trials, offering unprecedented efficiencies in site selection, patient recruitment and data analysis. By accelerating timelines and reducing costs, AI is not only making drug development more sustainable but also expanding access to life-saving treatments.
However, realizing AI’s full potential will require continued collaboration between technology innovators, pharmaceutical companies and regulatory bodies. As frameworks evolve to ensure data integrity, security and compliance, AI-driven advancements are poised to further shape the future of precision medicine, ultimately improving patient outcomes and reshaping healthcare.
Alivia Kaylor is a scientist and the senior site editor of Pharma Life Sciences.