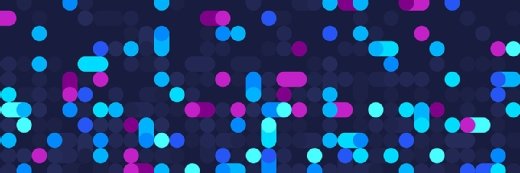
filo/DigitalVision Vectors via G
Exploring AI applications in radiology
Artificial intelligence can play many roles in radiology, including image analysis, interpretation, disease detection, and more.
Artificial intelligence (AI) has revolutionized nearly every industry worldwide, and the healthcare industry is no exception. From workflow optimization to data analysis and predictive analytics, AI has become critical in the healthcare system. One area where AI can be useful is radiology.
Radiology encompasses multiple kinds of medical imaging, including X-rays, ultrasounds, and magnetic resonance imaging (MRI). AI has been used and is continuously being researched for its ability to assist and optimize medical imaging.
Within radiology, AI technologies can be used in multiple ways, including image analysis and interpretation, computer-aided detection, workflow optimization, and more.
According to an article from Siemens Healthineers, “Artificial intelligence holds significant promise for radiology and is already starting to revolutionize healthcare in many ways. From bridging the gap between the demands of ever-increasing, extremely complex data and the number of radiologists to simplifying data interpretation through sophisticated AI algorithms and improving the diagnostic process. AI is a valuable tool that, when combined with the human expertise of radiologists and clinicians, offers vast potential to the healthcare industry.”
Image Analysis and Interpretation
Trained AI algorithms can be applied to image analysis and interpretation to analyze various medical images, including X-rays, computed tomography (CT) scans, MRIs, and ultrasounds, aiding in disease detection.
A 2018 article in Nature Reviews Cancer notes that AI tools could be used in multiple imaging areas, including the following:
- Thoracic imaging to detect pulmonary nodules
- Abdominal and pelvic imaging to identify and characterize lesions as benign or malignant
- Colonoscopies to detect and monitor polyps
- Mammography to identify microcalcifications
- Brain imaging to analyze abnormal tissue growth and make diagnostic conclusions
- Radiation oncology to evaluate treatment responses
According to an article from Radiology Affiliates Imaging, AI tools use convolutional neural networks to analyze medical images. These neural networks are, ideally, trained on vast and diverse datasets to identify patterns or abnormalities in medical images.
The organization identifies three main areas of imaging that AI can benefit radiologists.
- Image Classification: AI can place images into normal or abnormal categories.
- Image Segmentation: AI can divide the images into specific regions, allowing radiologists to observe and analyze specific areas more closely.
- Image Detection: AI can also detect abnormalities that may be missed due to human error.
Computer Aided Detection
Within image analysis and interpretation, there is computer-aided detection (CADe), which can highlight areas of concern in medical images, flagging potential abnormalities for radiologists to review. This can help reduce the chances of overlooking important findings.
CADe is approved by regulatory bodies like the FDA for use in mammography, lung cancer screening, and other applications. However, CADe systems should be used as a decision support tool rather than a replacement for radiologist interpretation.
Workflow Optimization
Radiology Affiliates Imaging also notes that AI can enhance or optimize radiologists’ workflow to address the challenges that many radiology offices face.
Siemens reports that, while the demand for radiologists increased from 3,467 whole-time equivalents from 2014 to 2023, the supply went from 3,048 to 4,118 whole-time equivalents in that time. This data suggests a 31% shortfall between supply and demand.
Radiologists at healthcare facilities often have to sift through and analyze a vast number of images, but with AI assistance, the process can be done more quickly. For example, radiology facilities can incorporate AI into their workflow for image preprocessing, using the tools to clean up medical images by removing noise or artifacts, allowing radiologists to get right to diagnosis.
In addition, AI can analyze medical images and generate reports that can help radiologists when sending information to patients or their providers. These reports may automatically highlight, characterize, or quantify anomalies for providers.
Challenges of AI in Radiology
Although AI integration in radiology has several potential benefits, multiple challenges must be considered when incorporating the technology.
Data Quality
One of the primary challenges in using AI is data quality. As mentioned above, AI algorithms and tools are trained based on existing datasets to detect and identify patterns in images. While training offers a foundation for AI conclusions, it means that the data being used must be high-quality, diverse, and unbiased.
Radiology datasets may contain biases related to patient demographics, imaging protocols, disease prevalence, and institution-specific practices. These biases can lead to suboptimal model performance, especially when deploying the AI system in new clinical settings.
However, radiologists, researchers, and AI developers can address this challenge using diverse, curated, and representative datasets that accurately reflect the population being assessed. They can also incorporate tools like active learning, domain adaption, and federated learning for bias mitigation. Finally, by conducting extensive testing and validation, radiologists can ensure the accuracy of AI models across diverse populations.
Regulation
The regulatory landscape around AI has been challenging for many hoping to incorporate the tool into medical imaging. Regulatory policies vary globally; however, the FDA has guidelines for evaluating and approving AI-based medical devices, requiring companies to show the safety, efficacy, and robustness of an AI model using clinical data and post-market surveillance.
However, as AI continues to evolve and new tools come to market, the regulatory landscape will need to adapt to address new tools and their accompanying challenges.
Oversight
Although models trained on diverse and robust datasets using quality data should be relatively accurate, radiologists must acknowledge that the technology is imperfect. There are still margins of error, and the technologies or algorithms can make mistakes.
Radiologists must maintain appropriate oversight and accountability for using AI-based systems in their practice. This includes understanding the capabilities and limitations of the AI system, validating its outputs, and taking responsibility for the clinical decisions made with the aid of AI. Radiologists should be actively involved in selecting, implementing, and monitoring AI-based tools to ensure their safe and appropriate use.
Addressing these challenges will require a collaborative effort between AI developers, radiologists, healthcare leaders, and regulators.