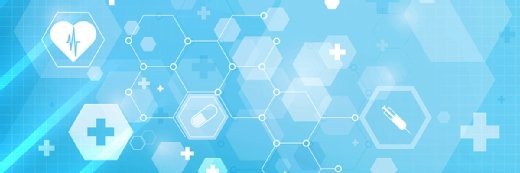
Rifqyhsn Design/istock via Getty
Why healthcare needs a patient-centered approach to AI use
Incorporating AI into clinical decision support tools could empower efforts to achieve patient-centered care if health systems know how to integrate these technologies effectively.
Clinical decision-making support tools that rely on AI can become a bridge between patients and providers, but only if they are employed with a patient-centered approach.
As the pace of AI uptake in the healthcare space rapidly increases, providers are finding many ways to employ AI-powered tools. In 2024, providers used AI tools to review medical literature (17%), help determine a patient's diagnosis (16%), review EHR data (9%), create a treatment plan (7%), identify a prognosis (6%), and more, according to a report from HIMSS and Medscape. While concerns remain around standardization, security and ethics, many physicians agree that AI has opened up exciting opportunities on both the clinical and business side of healthcare.
One of those opportunities is enhancing clinical decision support. Clinical decision support faces many challenges and has historically been a pain point for providers. However, with sufficient refinement, AI could be key to reversing that long-lasting trend.
AI tools can powerfully augment EHR-integrated decision support tools to equip providers with more information at the point of care. Many tools can retrieve patient data or external data to help providers make more informed decisions about patient care. They can identify patients' risk levels and make predictions about patient outcomes. These use cases and many more enable a more patient-centered approach to care.
In this episode, Prashila Dullabh, the vice president and senior fellow in the department of health sciences at NORC at the University of Chicago, sat down with Healthcare Strategies to discuss the opportunities and pitfalls of AI-powered clinical decision-making and what stakeholders can do to make the most of these tools.
Kelsey Waddill is a managing editor of Healthcare Payers and multimedia manager at Xtelligent Healthcare. She has covered health insurance news since 2019.
Prashila Dullabh: I think somehow the involvement of patients as part of decision support enabled by these apps is almost inevitable. It's going to happen one way or the other.
Sara Heath: Hi, and welcome back to Healthcare Strategies. I'm Sara Heath, an executive editor for Xtelligent Healthcare and the lead editor on our patient engagement website.
Today, we're going to be talking a lot about AI and how it can fit into the patient-centered care equation. AI has finally transcended this buzzword status in healthcare with industry stakeholders identifying and employing the technology with practical use cases.
One of those use cases is clinical decision support, the tools that clinicians use to help them make decisions about a patient's care. But as technology continues to proliferate, healthcare experts eye questions about the effects on patient experience and the industry's overall push for more patient-centered care. Here to talk more about AI and patient-centered care is Prashila Dullabh, the vice president and senior fellow in health sciences at NORC at the University of Chicago. Prashila is a clinician informatician and health services researcher with over 20 years of experience, including recent work on a project with [the] Agency for Healthcare Research and Quality looking into clinical decision support and patient-centered outcomes. Thanks for joining us today, Prashila.
Dullabh: Thank you. It's actually a real treat to be here and to talk about something that I am very passionate about and that has been the focus of my work for quite a long time now.
Heath: Yeah. One of these most practical applications for AI right now is clinical decision support (CDS). In what ways has the healthcare industry left patient-centered care out of the AI or CDS conversation? And what are the risks of not exploring how clinical decision support can support patient-centered care?
Dullabh: Well, Sara, as you pointed out, I would say that AI is all of the conversation at the moment, and I would say we are also at an inflection point. But I want to provide a little bit of context. So, for several years now, I would say the policy landscape has been shifting toward more patient-centered care. And this has translated in many different ways. So, for example, there have been initiatives to give patients more access so they can see the data that the doctors have about them. More recently, there have been efforts where patients can download their information and exchange it with others to support their care.
Even more recently, the Centers for Medicare and Medicaid Services has also been thinking a lot about how to include patient-reported outcomes and other patient information as part of their quality programs. Then, we've also seen this tremendous explosion of apps that patients get access to on their phones or through some other medical device. So, there are lots of ways in which patients are collecting information about themselves and having a desire to share that with their health systems.
All of this took place in this rather exceptional event called COVID. So, with the pandemic, in many ways, we have this natural [inaudible] for how patients can start interacting with digital technologies and clearly communicating with their health systems in different ways. And then we have the explosion of AI, which I think many people will say is a completely disruptive technology.
So, this is a long way of saying that I think, somehow, the involvement of patients as part of decision support enabled by these apps is almost inevitable. It's going to happen one way or the other. So let me give you some complete examples. Right now, we know that lots of hospital systems are starting to think about the use or actually using ambient technology. So by that, I mean patients come and see their doctors, there's some kind of listening device, the clinician gets consent, and the encounter that the patient and the doctor have is summarized and synthesized for some kind of AI tool. In some ways, it's a form of decision support. I'm sure you've heard a lot about these conversational agents, chatbots, where patients are communicating with their doctors. Before, this used to be via patient portal messages, but right now, there's this chatbot that sits in the middle. They might summarize information that the patient provides.
So I think it's already out there, so to speak. What I think as we move forward is going to become more important is much more a deliberate engagement and involvement of the patient, not just as somebody who uses the technology but as health systems decide what technologies to deploy. How will this work with the patient? What would be most effective? Actually thinking about patient advisory groups, getting information from patients as they design and develop these tools.
Heath: Yeah, absolutely. I know you all have been involved in some PCORI [Patient-Centered Outcomes Research Institute] AHRQ studies about the ways clinical decision support can drive patient-centered care. I was wondering if you could give me a little bit more information about this and the role of clinical decision support in patient-centered care.
Dullabh: This is my favorite topic, so thank you for the question. So, there are actually lots of ways in which patient-centered care actually becomes part of the clinical decision-support process, and so you are absolutely right. When you opened up the podcast, you talked about what CDS is, and that I would say is the traditional view of how decision support has been used when largely clinicians are the ones getting some support through the computer advising them what to do.
So, a lot of the work that I've been doing recently has been focused on what we are now calling patient-centered clinical decision support. So I just want to unpack what we mean by that. So, patient-centered decision support is really CDS that can support an individual patient or their caregiver, or in some cases populations of patients. Let's just say a group of patients that have diabetes or hypertension, to manage their condition. And it actually incorporates four factors. So let me just talk about these.
The first is around the actual evidence that is incorporated in the decision support tool, where the evidence is coming out of research which we call "patient-centered outcome research" that accounts for outcomes that the patient thinks are important, their goals, preferences. So, the research itself is reflective of this. So it's the incorporation of the research or the knowledge base in the decision support.
The second piece of it, in terms of how you're incorporating these patient-centered factors, is the patient's ability to give information about themselves that is not typically collected as part of the doctor-patient interaction. So, here's an example: data from a medical device, whether that's a glucometer or a blood pressure machine from home, information from your tracker or some other kind of wearable device. Patient preferences and goals are pretty important when we're thinking about using these tools, so there's actually information that a patient contributes that becomes part of the decision support tool.
The third component is around how the CDS is delivered. So we are imagining where patients via an app or the patient portal are actually receiving this information, and then being able to use that information to engage with their healthcare system.
And then, finally, we think about it in terms of its actual use, where the tool actually supports a shared decision-making process between patients and clinicians.
So, there are all these different components that drive a patient-centered aspect of decision support. Our work has shown that there are some common areas where these PCCDS tools seem to be taking hold, so, not surprisingly, in the management of chronic conditions, such as diabetes, hypertension, asthma. We're seeing use of these tools in supporting patients around well-being, whether that's diet, medication adherence, exercise. And then also an interesting use of these tools for some types of mental health apps and things like that, such as ADHD, depression. So those are the areas where we're seeing, we anticipate that over time there will be other areas where these apps will be used as well, or these tools, patient-centered clinical decision support tools.
And maybe I can give you just one quick example. So, some years ago, we actually worked on this very interesting project where we designed and implemented this app for moms that were diagnosed with hypertension due to pregnancy. And so for some of you that might know this, postpartum moms, the moms after they've given birth often get monitored for a period of six weeks because they have hypertension during pregnancy, and actually if the condition is not carefully monitored there can be some kind of catastrophic consequences for the mom, right? Like heart attacks, strokes, things that we really don't want to happen.
So what this app did was it would send a message to a mom every day saying, "Can you please give us your blood pressure?" And just a few basic questions about some symptoms. "Do you have headache or visual symptoms, or any abdominal pain?" And then that information via the app would get relayed to the health system. The health system would then be able to see in real time, "Oh, this patient's doing fine" or "There's some worries, some symptoms here, so we need to do something about it." And we had designed this dashboard that the clinicians would get access to.
So it's a pretty simple application, but this is the example of what we mean by patient-centered clinical decision support. So the patient every day would get a message. They'd enter some information. There'd be something to tell them, "Okay, we've received your information." If there's anything worrisome that the patient enters, then they'll get a little message to say, "Please contact your doctor." And then, on the other side, the clinicians would have a similar setup, if you will. And we actually did an assessment of these tools, and in general, we found that both the patients and the clinicians really liked it, because there was much more of a real-time interaction as opposed to waiting for a patient to come with a list of their blood pressure and perhaps symptoms, and an opportunity to do something in a more timely way had been missed. So, hopefully, that explains how we think about patient-centered context with these decision-support tools.
Heath: Yeah. And you started talking about it at the end, what do we know about patient comfort or readiness to interact with this kind of technology? I know that I've heard a little bit of research about patient comfort with AI, but I wanted to know if there were any insights that you have from your research.
Dullabh: Yeah. So, I think what I was talking about is what I would call more traditional patient-centered decision support. Let's talk a little bit about AI in patient-centered CDS tools, okay? So I think that's your question.
Heath: Yeah.
Dullabh: So we actually did a very interesting report or study recently where we explored exactly that, how do patients feel about the use of these tools? And not surprisingly, I think there's a cautious optimism. They recognize it's here and they do see some value in it, so to the extent that the AI offers certain conveniences, i.e., like supporting some kinds of administrative functions, allows them to interact more effectively with their doctors, we talked about conversational agents. Even the idea that if they go to see their doctor and there's some ambient technology that's recording the conversation, it actually helps the patient-clinician interaction because now the doctor is not staring at the computer, right?
So, there are all these things that are the use of these tools that I think are interesting and hold promise. But then we heard things like, "We think it's very important that there is transparency in what our doctors are doing, so we know if some response is coming from some kind of generative AI solution [and] if the doctor's looked at it." So, just transparency in the use of these tools was something that was definitely articulated as important. There seemed to be a view that, while these technologies would be helpful, this should always be a process where there's a doctor as part of the process.
So, I know we've heard this idea of the copilot model, but we heard quite clearly that's important and should be kept front and center. We've also heard things like, while the AI may support certain basic types of functions or some kinds of use cases, when it comes to complex medical conditions we really need to think about what are the right uses of these technologies. So, just to keep that in mind in terms of the complexity of the tool and when it can be used effectively. And then I think, most importantly, we also heard that patients didn't want a situation where it's like a phone tree where they can't get to a human at the other end, that there should always be a way to come out of whatever decision support, if you will, or interaction with a tool and reach a clinician so that they can have that experience.
So I would say there's cautious optimism, there's a recognition that these tools are here and hold value, but then there are these important things that we need to keep in mind as they get brought out more broadly.
Heath: Yeah, that makes a lot of sense, and that's very reflective of what I've seen in conversations with other people and in data sources, so very interesting to hear that you're finding the same thing.
I wanted to move on and talk a little bit about the Clinical Decision Support Innovation Collaborative. If you wanted to talk to me a little bit about how that even came about and maybe some of the goals, that would be very helpful.
Dullabh: Sure. So the Clinical Decision Support Innovation Collaborative has been funded by the Agency for Healthcare Research and Quality. And I think there's been this recognition given to all of the topics that we are talking about, that there's an important emphasis and focus around patient-centeredness when we think about these decision support tools. So this is a very interesting project in that I think there's a whole recognition that the healthcare ecosystem is complicated, and that there are many different individuals that have different needs and interests, and we need to take that into account as we're thinking about how we design and deploy these tools, so that they are used effectively. There's lots of information out there on how decision support tools, which we've been working on for many years, are not really used and the doctors find them particularly intrusive, don't really support the care that they want to deliver in an evidence-based way.
So, what the CDSIC does, it has brought together I think over 100 stakeholders, which include patients, clinicians, representatives from health systems, from payers, software developers, electronic health record developers, people that are involved in developing apps, decision support tools. So, we brought everyone together and we've established what we call a learning community where we bring together the interests, perspectives, needs of all of these different stakeholders. And, the whole goal of the project is using input from a broad community, generating evidence, and then developing tools and resources that the industry can use to advance patient-centered decision support.
Maybe I'll unpack that a little more. When we say we are developing tools and resources, if we want these kinds of technologies to be used effectively by both patients and clinicians, so we do realize the objective of better healthcare, we need to think very carefully about how they get designed, how do we involve patients as part of that process, how do we integrate these tools in both the lives of patients that are going to use them, as well as in the workflows of clinicians? Because they aren't going to be bringing any information from patients outside of the clinical encounter.
So, there are all of these considerations about how you design, develop and implement these tools. And part of the project is to develop resources and practical guides. How do you do co-design with patients? How do you collect patient preferences? When do you do that in the workflow? So there's one dimension of developing these tools and resources. We have an emphasis on using standards, so the idea here would be the tools and resources that come out of this project can be used by others. They are not proprietary and specific to one healthcare setting. So there's an emphasis around the use of these tools in a standard space way.
And then we have a very important emphasis around measurement. Given that we are thinking about deploying new technologies, I think there's a key recognition that we need to understand what's working, what's not working, how do we measure this so that we can build the evidence base. And over time, improve the adoption and use of these tools. And then lastly, we also have an opportunity to do these real-world pilots where we take some ideas, we find a delivery system that would be willing to work with us, so we get to implement and learn from the implementation experience.
Heath: Yeah, that makes a lot of sense. And, based off of what you just told me, this sounds like a very weighted question, but I wanted to hear about some of the insights you guys have gleaned so far. Maybe some of the strategies that clinicians can adopt to support some of this patient-centered AI in CDS use.
Dullabh: Just picking up on some of the themes across several questions, some of the insights that we have gleaned as part of this process -- we really do need to be thinking very carefully about when and how we engage patients and others that are meant to be using these tools, as well as clinicians. So there's this important part of engaging the right stakeholders so we design and develop these tools, so they fit into the workflows, life flows and meet the needs of those that need to use it.
There is, I would say, a fair amount of work to be done around learning how to implement the tools in different delivery settings because, as we know, there's no one-size-fits-all in healthcare, right? We need to be mindful of local context, circumstances under which something works and under which it doesn't work, so that we can share those lessons more broadly.
Then there's work to be done in terms of really thinking about how we understand and measure whether something's working or not. So, here's a perfect example. We talk about patient engagement, but as we sit here right now the measures that we have for patient engagement are pretty basic at best. So if you want to think about these tools and patient engagement and what results in better outcomes, we actually need better measures and ways to collect the data to inform those measures. I think most importantly, we also need to be thinking from a delivery system perspective. What are the implications of these new tools and the burden that they place or the implications in terms of what they might have to do differently?
So here's an example, if health systems are going to be bringing in data from patients, what policies, what processes do they set up for bringing this data to make sure that somebody sees that this information that has come in, that something needs to be acted upon? So I feel like that's a whole new area of work that we need to be thinking about. And then, I think, most importantly, we do need to be thinking about trust and transparency in all of the work that we're doing. It's pretty fundamental if we want to think about adoption and use over time.
Heath: Yeah. And just on the flip side, if you wanted to talk about maybe some of the pitfalls that clinicians should avoid to ensure that their AI use isn't stymieing any of their efforts for delivering patient-centered care.
Dullabh: Yeah. I think of those more from a healthcare system perspective. My general sense is that healthcare and how it adopts and uses technology tends to be pretty careful and deliberate. So I feel like there's a few things that healthcare systems are going to need to be thinking about.
Heath: Yeah.
Dullabh: Most importantly, this idea of: If they are using tools, what's that model in terms of how patients are being notified about the use of these tools? So, that trusted transparency dimension I think is going to be important.
Other areas that I think healthcare systems are also going to have to be thinking about is, as they think about which tools that they plan to deploy: Are those tools vetted? What's the seal of approval that they get that something's safe and secure? So, something else I think from a healthcare systems perspective that's going to be important.
I do think that there's value in having some more practical guidance for healthcare systems in the use and deployment of these tools. I think that's going to be quite an important area because, in the AI landscape, I think there's lots of conversation around principles, guiding principles -- transparency, accountability, fairness, explainability. But what that actually means in implementation and how to translate it, I think, is where the rubber meets the road.
Heath: Yeah, that makes a lot of sense. And then moving out of the hospital health system area, I know that your data is influential in a lot of policymaking. So just based on some of your own research, what does healthcare, as a policy-driven industry, need to do through that policy lens to ensure patient-centered clinical decision support?
Dullabh: I'm going to approach this with the policy view pretty broadly, and I want to be careful in terms of the approach that I take because my work is very much in the clinical space, so that's a lens that I will apply when I think about this.
But again, building upon some of the things that I've talked about, I think it's going to be very important to help us build the evidence base. If we want anyone to use these tools, they need to understand and appreciate that evidence that undergirds their use. The evidence base becomes very important. Lots more projects that clearly focus at scale on the use of these technologies, when they work, how they work, when don't they work, and what are some of the issues that we need to deal with collectively. I do think there's going to be an increasing need … given with the large language models and generative AI, where we don't always even understand how this tool is used … [for] some assurance that health systems, patients, when they're using certain tools and apps, have some kind of sense, they've been tested, approved, independently vetted, and are safe to use.
So thinking about what processes allow us to do that I think is going to be quite important. At some point, and maybe that point is now, there will have to be some thinking around the reimbursement models around the use of these tools. Large language models and AI are complex in that they have a role to play in multiple parts of a care process, so I think it opens up a lot of considerations of how we think about reimbursement models.
And then finally I do think there will be some implications to regulatory oversight. And again, we are dealing with these tools that can learn on their own, so unlike some kind of predictive algorithm where the inputs always result in the same outputs, with the use of generative AI as these models are learning, we cannot assure that the inputs at the beginning that generated certain outputs will be the same.
So how do we think about oversight on safety, quality, in a landscape where the performance of the models shifts over time? I think is going to be an important consideration. So I think those are, from a policy perspective, some of the things that surface on my radar.
Heath: Yeah, absolutely. That makes a lot of sense. Like I said before, it just ties into what I've been hearing across the industry, so it just goes to show how some of this research goes a long way in getting everybody on the same page.
Great. Well, thank you so much for joining us today, Prashila. I really appreciate it, and I think that this is going to be super fruitful for our listeners. And thank you to our audience for joining us today as well.
Dullabh: Thank you so much for the opportunity.
Kelsey Waddill: And thank you, listener, for tuning in. If you liked what you heard, head over to Spotify or Apple and drop us a review. We will be choosing some of our reviews to be read on the show in appreciation, so keep listening through to the end because you might get name-dropped. See you next time. Music by Kyle Murphy and production by me, Kelsey Waddill. This is an Informa TechTarget production.