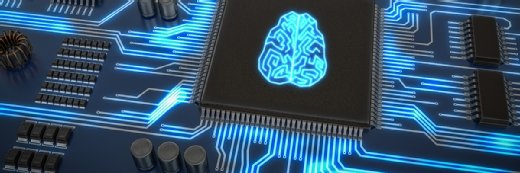
vchalup - stock.adobe.com
AI in IoT elevates data analysis to the next level
As IoT devices voraciously gather data, many companies are unprepared to take it all in. Deploying AI in IoT systems can make a profound difference when sorting through data.
While IoT is expanding by billions of devices annually, the enterprise clouds that process their output are only growing at a rate of thousands per year. That staggering mismatch produces several serious problems -- the biggest of which is that, for most organizations, there's no practical way to cope with all that data in a timely, efficient manner. Add in the challenges that the cloud presents to IoT data processing -- including latency, bandwidth, security and more -- and it's clear the benefits of IoT may never be realized.
Fortunately, AI in IoT is here. Advancements in both AI capabilities and IoT devices are bringing analytics where they need to be, solving IoT's top data challenges while creating dynamic networks and distributed intelligence.
The IoT data challenge: Round trips and real-time responses
In a typical enterprise network, IoT exists beyond the boundaries of the cloud, passing data back through a firewall, where it then takes residence in storage and is made available to some process or application. But with so many different devices reporting -- a number that will steadily increase -- traffic problems are inevitable. Managing the flow of so much data from so many endpoints is beyond the resources of most companies.
But wait; it gets worse. Many IoT applications are two-way streets, where data gathered by sensors has consequences in the locations they're reporting on; for example, adjusting the power consumption of a building based on changes in occupancy and weather. In many such cases, there's no time for data to make a round trip to a cloud.
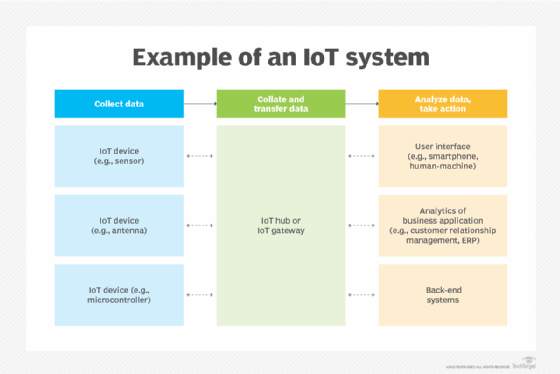
In fact, it's often the case that IoT data needs to be turned into responsive action in real time, especially in scenarios such as traffic management, security intrusions or equipment failure.
Enter AI
The fix for these problems is edge computing -- extending the processing power of an enterprise network by adding gateways and IoT devices that offer local processing power. This solves several big problems, including routing and accommodation of real-time response requirements, and creates a point at which data gathered can be parsed, with some information processed on the spot and information bound for cloud storage passed on separately.
For this to work as described, one final piece must be added: AI. And that AI must live not in the cloud, but on the edge. The work of IoT, in responding to changing conditions, managing disruption and fine-tuning resources, is all about pattern detection and machine learning -- activities that can now occur, via edge computing, where that work is happening. AI needs to move out of the cloud and onto the edge.
Where AI in IoT fits
When IoT data stays local and feeds into edge-based machine learning, patterns emerge. Those patterns can become predictive of machine faults or system failures, making it possible to apply preventative maintenance and avoid disruption or downtime in manufacturing environments, for example, or to anticipate interruptions in supply chains and adjust to avoid them.
More than that, applying machine learning to production environments, traffic management and other complex environments or processes can surface unexpected patterns that lead to their optimization. AI in IoT can create intricate, detail-rich snapshots of those environments and processes that, when studied, reveal potential efficiencies that could easily go undetected by human beings reading gauges.
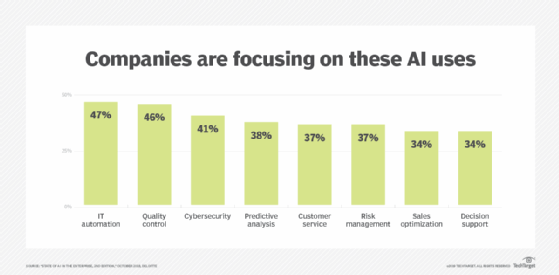
For example, a client of Caterpillar Marine, the marine division of machinery giant Caterpillar, used AI on IoT data collected from its fleet of ships to discover the affect hull cleaning had on ships' efficiency. The company justified the cost -- and importance -- of cleaning its ships' hulls twice a year versus once every two years, concluding that cleaning the buildup of barnacles and ocean life and remediating corrosion issues twice a year saved up to $400,000 per ship per year.
Finally, there's AI's application to the edge network itself. AI in IoT edge networks enables applications to monitor their own performance, self-optimizing over time as the applications and processes started to improve.
Call it distributed intelligence, the seeding of IoT deployments with local AI subnetworks that learn continuously, improve performance persistently and bring management not only to IoT data traffic, but to the information that needs to go back to the home cloud. It's a win-win with emergent benefits, not only solving big problems, but enabling big, often unexpected gains in the process.