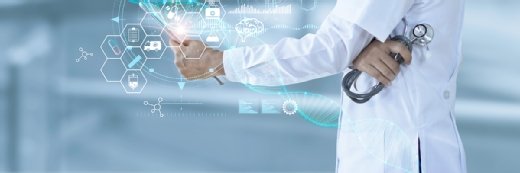
Getty Images/iStockphoto
How wearables, IoT and data paint healthcare's bright future
With the right IoT systems and architecture, IoT and AI will improve healthcare services with data from consumer wearables and the collective medical professional knowledge base.
With an unclear future of COVID-19, healthcare organizations and professionals are now, more than ever, looking for solutions to maximize the potential of their existing infrastructures and create new capabilities that will allow healthcare to be more accurate and accessible. This is where IoT comes in.
Don't build IoT; build IoT systems
It's amazing what a single IoT device can tell you, even if it's just a basic wearable, like a fitness watch. The real magic comes when you build IoT applications like a system.
Assume we have no data to detect a specific disease, but there is an urgent requirement to learn more about the spread or symptoms. If we build a system that collects and analyzes data, bring in machine learning (ML) experts who will build and train models, and have medical professionals to annotate and label the data correctly, we can improve training models. The quality and precision of the data analysis will eventually reach the level of a medical expert's predictions. Although COVID-19 is a chapter on its own, it raises an important question: whether we could use IoT to track the spread of the virus. The answer, however controversial, is that this is possible. The crucial technology to do so is patient monitoring via smart devices coupled with analytics.
What we've just painted here is quite a complex picture, so let's break it down. In the common IoT approach, there are several layers of IoT applications in healthcare:
IoT wearables and devices
Medical wearables include Neopenda's health monitoring device for newborns and the latest Apple Watch. Many medical professionals would cringe with the mention of consumer wearables. The truth is, 10 years ago, you needed to visit a hospital to learn information about your electrocardiogram using expensive, heavy machines. Today, anyone can measure pulse rate, electrocardiogram and other important information from devices the size of a coin. Manufacturers can make devices small, efficient and convenient for the user.
Data aggregation and analytics
We can get lots of interesting data from an Apple Watch and similar consumer gadgets, but this data is not medical-grade certified. Aggregating this data is a start. If healthcare professionals can combine consumer device data with medical grade data from professional devices, and apply ML and sophisticated analysis, then we can create useful insights that will make treatments more efficient and will help professionals predict issues. Thirteen types of cancer are lifestyle related, as well as cardiovascular and other diseases, all of which could be predicted through the collection of data from wearables. Individual diseases not only could be predicted on an individual level but could also be used on a wider national level if we apply data aggregation and analytics.
Now, even mentioning big data in regard to healthcare is like entering a minefield, given the amount of regulations in this space. However, with ML, learning techniques and the huge amounts of data we can collect combining medical devices and lifestyle gadgets today, we can create reports like we've never seen before.
Of course, IoT is not just about consumer data from wearables. For example, today we can make a relatively simple device intelligent, such as a CT scanner, by tying all the data the scanner produces back in the cloud and then applying analytics to create crucial insights.
Cloud services
Cloud managed services support big data by providing a huge data storage, which makes related operations simpler. However, lots of data doesn't equal quality data. To use all the aggregated data, it must be cleared and labeled properly with the help of medical experts. For example, a layman might be able to spot lung cancer even with basic training, but if we want to be able to detect a particular issue earlier when its signs are more subtle, we need to make sure we don't confuse it with another diagnostic that might show similar signs. High-quality data that is medically certified helps clarify these nuances.
Collecting and processing data at scale makes actual applications and predictions possible that can help not only medical experts but also individual users, as well as large populations, insurance companies and third parties involved in the complex healthcare system. For example, insurance companies can provide conditions tailored to their clients, track activities and build specific models to estimate potential risks.
3 reasons to use IoT in healthcare
We've now looked at the technical layers of building IoT-based systems. But for some, the question remains: Why should we use IoT in healthcare?
First, IoT enables what we call an "AI-augmented doctor." It's not about replacing medical professionals, but about helping them augment their day, their diagnostics and knowledge.
In some real-world trials of deep learning such as CT scans for cancer, AI typically performs at a similar level to a doctor. But it's not about comparing professionals to technology; AI should be seen as a tool that can enforce medical expert capabilities by picking up subtle signs. For example, if there are 100 cases of cancer, a doctor might detect 70 cases. But ML can examine the data and spot relationships that were never expected or predicted previously, providing information to medical experts. The diagnostic process is a complex one, and a doctor might write off a particular diagnosis as a fluke, but it's ML that can provide the data needed to avoid missing crucial correlations. Furthermore, AI and ML explainability tools can help understand and interpret predictions, providing doctors with suggestions on what diagnosis they are detecting. Medical professionals can use or disregard this insight. AI would serve as a high-tech sounding board.
Second, IoT enables us to move from individual to institutional knowledge. If 100 professionals look at a CT scan, they might come to different conclusions. This comes down to their level of expertise and experience. When using AI, we can leverage individual knowledge and make it institutional knowledge. Everyone gets at least the same baseline because the AI model will always perform at the same level.
Lastly, IoT data gives power. We've already covered aggregation of data. The whole IoT system wouldn't be possible if it didn't start with an IoT device in the beginning. But IoT wouldn't be used to its maximum potential without collecting and analyzing the data available. Start collecting data, today, even if it's just to build repository data for the future.
How to build the right IoT system
Healthcare organizations are, in a way, businesses like any other; they need answers to questions, but efficiency is equally important. The process of creating an IoT service should be treated similarly to technical projects in other sectors, following a path from problem statement to the discovery stage to proof of concept, then ideally to a pilot based on carefully selected and refined architecture. Building a minimum viable product with an agile approach allows development teams to gather crucial feedback from early users and then, finally, approach the production-ready stage.
When it comes to efficiency, this is where architecture matters. Healthcare organizations need to consider existing technology. Often organizations have legacy infrastructure that should be, from a technical efficiency point of view, thrown away, but that's not always possible if there was a huge investment into the hospital 10 years ago. It's not always the case though. Using data principles, such as loosely coupled data layers, can allow new solutions to be useful to the legacy environment.
In general, building a functional infrastructure from scratch tends to pay off in the long run, and allows healthcare organizations to be future-proof. It's worth embracing proofs of concept. By working with small projects to prove the value and efficiency, healthcare organizations can make projects more efficient from a cost perspective and increase their chances of further investment from key stakeholders or external partners.
Fast forward to the future
Professional machines will get smarter exploiting data and AI; there is no doubt about that. What we also expect to see in the years to come is a harmonization of healthcare standards that will bring together two parties: professional medical care and the results from consumer wearables. We are already seeing steps to unify these domains, and development on how we can prove the usability of data from devices. The power of using IoT and big data in healthcare is still unfolding and faces many hurdles -- such as regulations -- but there is a huge potential to make healthcare more accurate, efficient and most importantly, available to all.
About the author
Henry Brown is the data and analytics director of Ciklum. Brown has experience in leading data transformation, machine learning adoption and cloud-native projects across organizations and sectors such as the public sector, retail, commodities, financial services and manufacturing. Brown has a wealth of knowledge and experience in data and delivering value through data, including working on data predictions for both the U.K.'s 2016 Brexit vote and the U.S. election. At Ciklum, Brown works with Fortune 500 companies and startups, helping them grow and leverage business opportunities through strategic consultancy and custom-built data, analytics and AI solutions. He also has a Ph.D. in particle physics from the University of Liverpool, where he worked on the Large Hadron Collider beauty experiment at CERN, the Conseil Européen pour la Recherche Nucléaire, which translates to English as European Council for Nuclear Research.