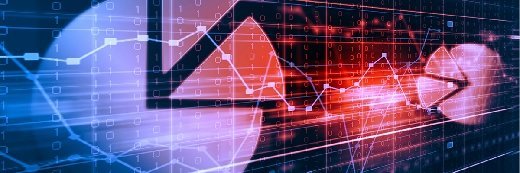
Sergey Nivens - Fotolia
IoT data analytics holds the key to putting data to use
Applying IoT data analysis to info gathered from connected devices is key to extracting the data's potential value. Knowing how to get from potential to realized, though, is the challenge.
While getting ahead in today's competitive landscape isn't easy, IoT data analytics promises to help. But if you think you can slap some sensors on machines or in products and instantly have useful data, think again. Once collected, the data must be analyzed and transformed into actionable insight, not stuck in siloes or stored away and forgotten. Yet, for many, the sheer volume of data and pace at which IoT data is generated is overwhelming.
Regu Ayyaswamy, vice president and global head of IoT at Tata Consultancy Services, knows the benefits of IoT data analysis, as well as the challenges organizations face to implement it. In this Q&A, he clarifies the current state of IoT data analytics and reviews the steps enterprises should take to get the most from their connected data.
A 2015 McKinsey report found that as little as 1% of IoT data is ever used. But IoT has come a long way since. Where do you see this number today?
Regu Ayyaswamy: Over the last three years, we've seen a significant increase in volume of data put to use -- and it's also being used in very innovative ways. The adoption and migration of data to cloud is helping facilitate this. While we do not have a firm number, we think it might have increased five times since the 2015 report.
Which enterprises best capitalize on their IoT data analytics projects?
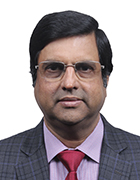
Ayyaswamy: Enterprises where the executives have been able to understand, absorb and drive these IoT-led transformations are better positioned to capitalize on the benefits of data. Industries like manufacturing, oil and gas, utilities and transportation are frontrunners in making the best of their IoT data. Benefits include being able to visualize real-time data in changing customer demands and expectations, personalized feedback on product usage and improved productivity, supply chain and fleet management, and asset monitoring and diagnostics.
For example, PostNord Sverige AB, the Swedish postal services, now receives real-time fleet data and feedback it never had before. Managers can use the IoT data to understand and track the fleet and its movements, perform dynamic routing and trace the movement of the goods to the last mile. This allows the organization to improve customer experience by ensuring on-time delivery through IoT.
In another example, we're working with a Japanese utilities company to gather data from its coal-fired boiler and use AI-based algorithms to tune the performance, resulting in reduced emissions and greater efficiency.
With so much data being collected and only about 5% being put to use, what happens to the other 95%? Is IoT data waste a cause for concern?
Ayyaswamy: The business use case defines the nature of data that will be required, and the analytical technique used decides if the data is useful. The right architecture, with the right blend of edge and cloud-based data processing, helps reduce IoT data waste.
Note that sensor data is historically used for controls in process industries and can't be termed as waste. IoT uses the data for better predictive models, which have not been put to use before.
What's so different about IoT data that makes it more difficult to use than data from even five or 10 years ago?
Ayyaswamy: Data was always there. The challenge was around the availability, accessibility, analytics and the ability to derive meaningful insights from it. The cloud, ready-built platforms to process data, high-performance computing power on tap and AI has since transformed data usage. The game-changer has been real-time data insights, which was not the case five to 10 years back.
What are the different types of IoT data analytics? How do you decide which to use to best capitalize on your IoT data?
Ayyaswamy: IoT data can be put through various analytics techniques, depending upon the type of data, pattern, periodicity, availability and volume. A combination of analytical techniques, physics and data sciences through statistical techniques and AI models and algorithms will successfully lead enterprises through data and information toward intelligence. There are currently multiple levels of analytics maturity -- namely descriptive, diagnostic, predictive, prescriptive -- which enterprises are going through in stages as they embark on their IoT journey.
Which technologies are key to getting actionable intelligence from IoT data analysis?
Ayyaswamy: Big data, machine learning, artificial intelligence and cognitive computing are some of the key technologies. These technologies, coupled with data sciences and physics and processed with key algorithms, are helping enterprises use actionable intelligence.
In terms of IoT analytics tools, there are several available off the shelf from various providers. However, mere availability and the use of tools does not assure insights-based outcomes. Models are built on tools, but the organization's ability to combine its domain, physics and data science to truly understand the system is key for success.
If an enterprise is starting its IoT journey, what steps would you offer to help them successfully analyze their IoT data?
Ayyaswamy: The IoT journey is one of gradual adoption. As enterprises are moving from simple IoT data analytics to more advanced analytics to solve complex business problems, they need to plan this transformation in a phased manner with a realistic time frame.
The benefits from IoT data are best realized with its attribution to business value. And as mentioned, the IoT data sources determination, frequency of data collection and combination of data science and physics-based AI are key elements in IoT success.