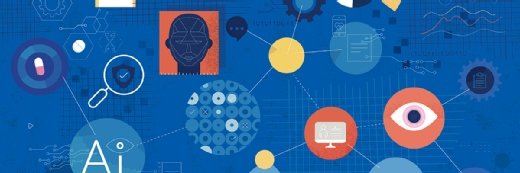
DrAfter123/DigitalVision Vectors
Bringing artificial intelligence into prior authorization processes
Artificial intelligence could play a major role in reducing providers' documentation and substantiation burdens during the prior authorization process.
Prior authorization -- the process through which a health plan grants or denies coverage approval prior to a patient receiving a healthcare service or filling a prescription -- plays a crucial role in the U.S. healthcare system, allowing payers to potentially trim unnecessary spending. But the process is rife with challenges, particularly for the providers who must document and substantiate prior authorization requests for their patients.
Historically, prior authorization has also been a significant regulatory burden for providers, outpacing existing challenges like EHR interoperability and compliance with the No Surprises Act. While the burden of prior authorizations on providers is high, with some indicating that the process is a major impediment to providing care for patients, prior authorization is unlikely to go away.
Solving the prior authorization problem requires stakeholders to balance the need for healthcare affordability and access to quality care, which is no easy feat.
However, aspects of prior authorization could be automated due to their transactional nature, and the industry is increasingly looking toward automation and other technology-driven solutions to streamline the prior authorization process.
Technologies like artificial intelligence have been touted as the solution to healthcare's myriad problems -- from automating hospital discharge to alleviating nurses' documentation burden. But what prior authorization pain points could benefit from the deployment of these tools, and how can healthcare stakeholders effectively adopt these technologies without creating additional workflow burdens?
In this episode of Healthcare Strategies, Amber Nigam, CEO and co-founder of Basys.ai, details how AI could streamline the prior authorization process for both providers and payers.
Shania Kennedy has been covering news related to health IT and analytics since 2022.
Amber Nigam: AI can also help find and fix certain blocks, which might be blocking, let's say, prior authorization or utilization management, or any such workflows, in order to make this process more streamlined and faster and more equitable, as well.
Kelsey Waddill: Prior authorizations are a key part of the U.S. healthcare system's current framework. Payers may require prior authorization before patients can obtain certain services or treatments, giving them a chance to approve or deny the procedure -- and, potentially -- cut unnecessary spending. But this process can cause a lot of burden on providers, who have to document and substantiate their prior auth requests, along with everything else they have to do. Our VP of editorial, Kyle Murphy, chatted with Amber Nigam, CEO and co-founder of Basys.ai, about how artificial intelligence could help solve this challenge.
Kyle Murphy, Ph.D.: Hello, and welcome to Healthcare Strategies. Today, we are talking prior authorizations with Amber Nigam. Amber, tell us a little about your healthcare story: How'd you get into, not only healthcare, but into technology? How do you marry those two in the work that you're currently doing today?
Nigam: Absolutely, and first of all, thanks for having me. I would say my healthcare experience actually started when my dad had diabetes. I was his caregiver, and I saw a lot of gaps in healthcare. And to your point on technology, as well, I essentially had a technology background. I had a bachelor's degree in computer science [and] worked as a data scientist for the first five years of my career.
The last seven years have been [spent] on entrepreneurship, but when I saw those gaps and I felt -- What can be done, and then, how can technology be helpful? -- I really felt this is one of the domains where technology can be helpful. At the same time, this was something that was close to my heart and my own experience as well, or vicarious experience from my dad's experience, and that's how I got started in healthcare.
So technology-wise, I had a bachelor's degree, like I said. I had previously also filed three patents on data science, instructed a course in technology and healthcare at MIT called Collaborative Data Science for Healthcare. So, that's how it started mixing together until my co-founder and I -- we met at Harvard, we were at the same grad school program -- we decided that we should work on a problem which is in healthcare, and what better problem to work on than prior authorization, which is the first communication step between the payers and the providers.
Murphy: That's an awesome segue into the bulk of the conversation today. I'm really interested in what were some of the pain points that you saw in the prior authorization process in healthcare, and where did you see technology being the right fit, or what kind of technology did you see as the right fit for this particular process?
Nigam: Absolutely. I feel [that] right now, the process is quite ambiguous, and it wouldn't be wrong to say it's also subjective, as well, because of the use of phone calls and faxes.
At a conceptual level, where data science or machine learning and technology can help is to disambiguate this process and make it more objective. But if I have to be more specific, on the payer side we have -- or health insurance company side -- we have healthcare policies which sometimes might not be very straightforward and not easy to understand.
Machine learning can disambiguate those policies and make them very straightforward, or have checkboxes, instead of people calling each other and sending faxes, which might be blurry. At the same time, it's not just prior authorization, it's also about utilization management, how different medicines and procedures like surgeries are utilized to bring the cost down or to keep the cost in check so that healthcare has more accessibility.
And finally, on the payer side, sometimes it's also about explaining your decision-making process. So, these are some of the components on the payer side. On the provider side, we have a lot of workflows. There's almost too many workflows for providers to manage, which creates a lot of chaos sometimes.
So, AI can essentially help in providing some sort of interoperability through which those workflows could be streamlined. At the same time, personalization is also key when you talk about healthcare, because it's not a one-size-fits-all approach that works in healthcare. If I, as an individual, am more prone to having diabetes, medications for me might work differently as compared to someone else. So, I would say there's a lot of nuances that AI helps in uncovering when we talk about different stakeholders in healthcare, or healthcare in general, as well.
Murphy: Now you mentioned all the different ways that prior authorization takes place. Those of us who benefit from the consumer space where everything is highly digitized, healthcare is very much ... there's still some physical paper involved, there's still some faxing involved, there's still some manual processes involved, which really create a lot of lag. What are some of the challenges with bringing AI to bear on these processes, many of which aren't really optimized or haven't been digitized yet?
Nigam: Yeah, I would say threefold. One is inertia. Even if there is machine learning, AI [or] data science at the ground level, you need to really adopt it, and moving away from phone calls and faxes -- especially when people have been used to it for the past couple of decades, not just couple of years -- it's difficult. So, you need to understand, as a technology company, or as a technology vendor, or even as a payer or a provider, you need to have that perspective that healthcare sometimes is different from a technology domain.
At the same time, even if it is adopted, sometimes in recent technologies, as we have seen, there is a problem of hallucination. Generative AI is supposed to hallucinate sometimes and generate something which is not true. So people, if they see such instances, they're of course driven away from technology because it's like a black box -- it might say something right, it might say something wrong.
And even if it's a 0.1% scenario and humans are, let's say, making mistakes at 1% scenario point, 1% is still improvement over a human committing mistakes. But I think technology has a different yardstick of measurement in that perspective.
The final thing is about privacy. So when you're using, let's say, technology in a very move fast, break things way, you tend to skip things. You tend to ignore certain regulations which might be extremely harmful because you might be in danger of exposing some of the patient or member-related information. So, I would say these three are some of the things that we should be careful about when we are adopting technology.
Murphy: Now, there's a lot that the average patient or health plan member doesn't really understand about prior authorizations when it comes to compliance with healthcare regulations, all these different standards. How can AI improve compliance? And then, if I could add to that, how can it maybe create greater opportunities for communication as to why something didn't turn out a particular way, or could we identify trends to avoid certain mishaps or breakdowns in communication?
Nigam: Absolutely. I feel one of the most important parts or roles that AI can play is on personalization. That is, if, let's say, as a member, if I have certain preconditions that I should be aware of, and let's say it would be easier for health insurance companies, payers or providers to know those things, AI can really bubble those important details up. So that's one way.
The second way is when there are compliances, usually they're not just at state level or federal level, there are quality standards as well. So, you need to be mindful of that. AI can really help in superimposing different standards and resolve prior authorization queries, keeping in check that all the standardizations and regulations are met, which for a human being might be a bit difficult. Sometimes it can be a bit subjective as well when human beings are the only entities who are keeping a check on those.
And finally, I would say AI can also help in identifying and fixing certain blocks which might be blocking, let's say, prior authorization or utilization management, or any search workflows, in order to make this process more streamlined and faster and more equitable, as well.
Murphy: What considerations do you have to make as an AI company when it comes to -- you mentioned data privacy before, but data security, we obviously understand the importance of HIPAA [and] HITECH in the space. There's no shortage of regulation dictating what you're supposed to be doing with that data. And you also mentioned black box and things like that. How do you ensure transparency, but also ensure the security and the protection of sensitive health information?
Nigam: That's a very interesting question, and something that we are also wrapping our heads around all the time because the policies keep changing. But what we have seen so far in our journey is like a three-folded approach.
First of all, as an organization, you need to establish guardrails, not just at your level. Sometimes when you're communicating with peers and providers, you need to be mindful of their guardrails, as well, and establish your structure based on that.
The second way through which you can also establish certain trust is by having or going through audits. So, enforcing those audits and making sure that you are compliant with whatever guardrails that you were hoping to achieve is really important as well.
And finally, there's one very understated component of compliance, which is ignored sometimes: interoperability. So, when you go for interoperability, you are exchanging a lot of information. So, how you marry that interoperability with the guardrails that I was talking about and remove silos and consider the true breadth of your data pipeline so that there is no leakage is really important, as well.
Murphy: What kinds of AI technologies are currently being used in the prior authorization space, what are the most mature, and then what's on the horizon? Where do you see future opportunities? Obviously, how we define AI is really a snapshot of where we are. Technology, in terms of development, it's always changing.
Nigam: I think, to your first question, right now, it's more about making sure we have the right workflows even before implementing, let's say, AI or machine learning. Making sure that we are not missing anything because, like I mentioned, privacy is really important in healthcare, and you also don't want to be automating certain cases which might be harmful for members or patients.
To my point, I'm talking about automating denials, for example. It's so bad, you cannot afford to do that. At the same time, if you consider AI and where it is leading us right now, I feel that generative AI is quite transformative. At the same time, we have to be mindful of the shortcomings of generative AI, as well. For example, we at Basys are ... We just filed a patent for sieving the fluff out of generative AI output, which uniquely differentiates us as well.
So, being mindful of what can go wrong -- being very structured and systematic about what can go wrong and then addressing those points -- is really important. And some of the other things where technology might help, or AI might help, like I previously mentioned, was interoperability, because a lot of standardization that AI can do can be utilized in interoperability measures.
And the third, and one of the most important things, is also about making sure healthcare, given its traditional background, you need to be implemented ... on the ground in order to have any sort of impact. So, how do you use AI to reduce the friction between payers and the providers? How you use AI to make sure -- even within a payer organization, or a health insurance company -- organizations [are] able to use your product in a very streamlined fashion is quite critical in adoption of AI.
Murphy: What's it like being an AI company and working in the healthcare space? This is an industry that has been rather slow to adopt new technologies, [with a] high level of skepticism. Have you encountered challenges with just getting people to accept the idea, or have they moved past that kind of stage of acceptance to now they're seeing opportunities where they can apply AI more strategically?
Nigam: I think it depends on who you're talking to. For some people, it's still a barrier. There's still some sort of inertia, and they need to see you as a neutral party, neutral vendor, for example. And you're not just preaching, 'Just use the AI and every problem will be solved,' like not, essentially, drinking the Kool-Aid, if you will.
But at the same time, what I have also seen and observed is people are getting more and more aware of what's out there in the market of what's actually happening with AI, especially with the advent of generative AI. They're seeing instances -- in different industries, not just in healthcare -- where generative AI can be helpful, where machine learning can be helpful. And there is a scope of a lot of automation. One thing that I have seen in the past is, as much as payers want or providers want to have that neutral system, which right now is generally not available, vendors are typically either payer-facing or provider-facing.
We, at Basys, try really hard to be that transparent and neutral platform. For example, we have payer partners, we have providers who are some of our investors, like Mayo Clinic is one of our investors. And then we have pharma, like Eli Lilly and Company, they are our investors, as well.
So, that's one thing which we feel is really important. The second thing that we also feel is important is everyone realizes healthcare is quite expensive, and we need to reduce that operational burden. And I think this is one common pain point that payers and providers have. And I think we -- given that our focus is on making sure the operational burden is reduced and, essentially ... the prior authorization staff is actually working, or repurposed to work on something that they were really hired to work on -- I feel this is something that everyone buys as well.
And the final thing that I would also mention is on policy. Given that, right now, there's a lot of flux in the policy space, I do see in three to five years' time, it'll start normalizing, it'll start maturing. So, in five years' time, we can almost have automated prior authorization acceptance at least for maybe 70% or 80% of the cases. And for certain denials, we might also have better propositions. So, not just deny, but also propose something which is relevant for the member. So, these are some of the things that I feel would happen in the coming years.
Murphy: Now, we started this conversation off with you talking about, you're helping your father with his diabetes, his chronic disease. What's your level of optimism now of the potential for technology to improve the healthcare system? Are you more optimistic? Clearly, you're still working, so I have to imagine you're excited to get up and go to work every day. What's your passion level nowadays? Have you made progress?
Nigam: I think it's 10 times right now because when I had started, I had zero context, and I was almost confused, and I was also worried. I had so much anxiety because of my dad, and sometimes his sugar level used to go down -- crash -- and we did not used to know what will happen. And when we used to schedule doctor's appointments, it used to be so far down the line, sometimes doctors would say that he needs this medication, but it requires him to go through certain more conservative therapies. And we did not know what is that.
Now, knowing what all these jargons are, and also understanding at a policy level, at a macro level or at a population level as well, what typically happens, I think I'm much more grounded in reality and having the technology background ... So just grounding yourself in the reality has been really motivating for me. And I think I have become more appreciative of the fact that healthcare is in the process of accepting me than the other way around. It feels really good. I'm absolutely passionate about the work that I'm working on, my team is working on, and I'm honored and grateful to be a part of this ecosystem, actually.
Murphy: Well, Amber, thank you so much for sharing your experience with us today, and we really appreciate your time.
Nigam: Thank you so much, Kyle, for having me.
Waddill: And thank you, listener, for tuning in. If you liked what you heard, head on over to Apple or Spotify and drop us a review. We'll actually be choosing some of our reviews to be read on the show in appreciation. So, keep listening through to the end because you might get name-dropped.
Thank you to Mayra W. for reviewing our show. Mayra wrote, "Great information! Recently discovered this podcast, and I'm hooked. A binge-worthy show talking about everyday solutions to providers' common problems, with top expert guests that share their experiences with these challenges that impact healthcare today."
We're so glad to get to bring you experts like Amber and so many others, Mayra. Thank you for sharing your thoughts. That's it for today, but tune in next week to hear some timely health IT security strategies from our very own Jill McKeon, associate editor on Healthtech Security. See you then.