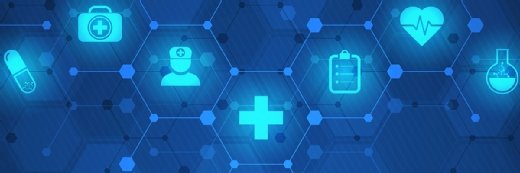
bestbrk/istock via Getty Images
AI algorithm determines probability of heart condition
New research shows that calibrating the scores of an AI algorithm can help clinicians identify and triage high-risk hypertrophic cardiomyopathy patients.
Calibrating scores of an AI algorithm can help clinicians identify patients with a high risk of developing hypertrophic cardiomyopathy, or HCM ,a type of heart disease, and quantify the risk they face, according to new research.
HCM is typically caused by abnormal genes in the heart muscle that make the heart chamber walls thicker than usual. This reduces the amount of blood pumped in every heartbeat, resulting in chest pain and shortness of breath, and in some cases, heart failure. The study published in NEJM AI assessed an AI algorithm called Viz HCM, which previously received FDA approval for the detection of HCM on an electrocardiogram (ECG) and assigned numeric probabilities to its findings. Viz HCM is developed by health AI company Viz.ai, which sponsored the study.
The researchers used the Viz HCM algorithm to identify HCM among 71,000 patients who had an ECG between March 7, 2023, and Jan. 18, 2024. The algorithm flagged 1,522 patients.
The research team confirmed the diagnoses using records and imaging data. They then applied model calibration to the algorithm to determine whether the probability of having HCM correlated with the actual likelihood of patients having the disease. They found that the calibrated algorithm accurately estimated a patient's likelihood of having HCM.
For example, without calibration, the algorithm would have only flagged HCM as "suspected" or "high risk." With calibration, the algorithm can provide a more specific risk calculation, such as "You have about a 60 percent chance of having HCM," said study corresponding author Joshua Lampert, MD, director of machine learning at Mount Sinai Fuster Heart Hospital, in a press release.
Researchers concluded that calibrating the scores of the AI algorithm offers critical information for clinical decision-making and can help prioritize patients for follow-up.
"This study reflects pragmatic implementation science at its best, demonstrating how we can responsibly and thoughtfully integrate advanced AI tools into real-world clinical workflows," said study co-senior author Girish N. Nadkarni, MD, chair of the Windreich Department of Artificial Intelligence and Human Health and director of the Hasso Plattner Institute for Digital Health at Mount Sinai, in a press release.
"It's not just about building a high-performing algorithm — it's about making sure it supports clinical decision-making in a way that improves patient outcomes and aligns with how care is actually delivered. This work shows how a calibrated model can help clinicians prioritize the right patients at the right time, and in doing so, help realize the full potential of AI in medicine," said Nadkarni, who is also the Irene and Dr. Arthur M. Fishberg professor of medicine at the Icahn School of Medicine.
The study highlights the efficacy of AI models in cardiac care, adding to growing evidence in the area.
For example, research released earlier this month shows that a noise-adapted AI model can estimate heart failure risk using single-lead electrocardiograms. Researchers tested the model in various cohorts, one of which included 192,667 Yale New Haven Health System patients.
Of these, 22.2% screened positive on the AI model applied to the baseline single-lead ECG. A positive screening result was associated with more than a five-fold higher risk of developing heart failure, the researchers found. A total of 3,697 (1.9%) of patients developed heart failure over a median of 4.6 years.
Researchers concluded that a single-lead ECG could be used to create community-based screening programs for heart failure.
Anuja Vaidya has covered the healthcare industry since 2012. She currently covers the virtual healthcare landscape, including telehealth, remote patient monitoring and digital therapeutics.