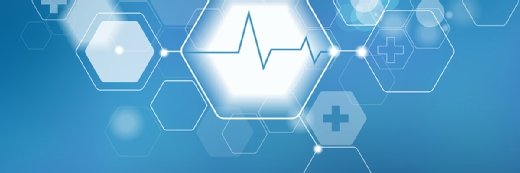
AlfaOlga/istock via Getty Images
AI model estimates heart failure from single-lead ECGs
Researchers have created an AI model that uses single-lead ECGs to predict the risk of heart failure, which could provide a new risk-stratification strategy for the disease.
A noise-adapted AI model was able to estimate heart failure (HF) risk using single-lead electrocardiograms (ECGs), indicating a new and potentially large-scale HF risk-stratification strategy, according to new research.
Published in JAMA Cardiology, the retrospective cohort study included individuals without HF at baseline who obtained outpatient ECGs in Yale New Haven Health System, as well as prospective population-based cohorts of the UK Biobank and the Brazilian Longitudinal Study of Adult Health (ELSA-Brasil).
Researchers from institutions in the U.S., U.K., Australia, Brazil and the Netherlands conducted the study, which tested whether an AI model developed to detect subclinical LV systolic dysfunction (LVSD) from single-lead ECGs can predict future HF risk. The AI model was designed to be resilient to noise to address challenges associated with noise introduction when obtaining single-lead ECGs. Researchers noted that the model, combined with the increasing availability of portable devices capable of recording single-lead ECGs, could expand HF risk stratification.
The study shows that of 192,667 Yale New Haven Health System patients, 42,775 (22.2%) screened positive on the AI model applied to the baseline single-lead ECG. A positive screening result was associated with more than a five-fold higher risk of developing HF, the researchers found. A total of 3,697 (1.9%) of patients developed HF over a median of 4.6 years.
Within the UK Biobank, 5,513 of 42,141 participants (13.1%) screened positive with the AI-ECG model. After accounting for age, sex, HF risk factors and the competing risk of death, a positive AI-ECG screening result was associated with a five-fold higher risk of HF. Forty-six patients (0.1%) in the UK Biobank developed HF over a median of 3.1 years.
Among ELSA-Brasil participants, 1,928 of 13,454 (14.3%) had a positive AI-ECG screen, indicating a nine-fold higher HF risk compared with those who screened negative. Overall, 31 ELSA-Brasil participants (0.2%) developed HF over a median of 4.2 years.
The researchers noted that using "a single portable device to record ECGs for multiple individuals could support the design of efficient community-based screening programs."
However, "future studies are required to determine if this AI-ECG–based noninvasive digital biomarker can enable improved stratification of HF risk across communities," they wrote.
The study adds to evidence showing that AI algorithms can use single-lead ECGs to detect heart issues.
In a May 2022 study, Mayo Clinic researchers described their AI algorithm for detecting a weak heart pump using single-lead Apple Watch ECG signals. The researchers adapted the algorithm from a 12-lead ECG algorithm for which the FDA awarded breakthrough device designation in 2019.
They enabled 2,454 patients to record Apple Watch ECGs using a smartphone app, collecting 125,610 ECGs in total. The average patient used the app twice a month over the six-month study period. The algorithm had an area under the curve of 0.88.
Similarly, the University of Michigan developed an AI model in 2022 that could predict hemodynamic instability more accurately than traditional vital sign measurements to indicate patient deterioration. The model, which used data from a single-lead ECG, achieved 97% sensitivity and 79% specificity, outperforming the vital sign measurement.
Anuja Vaidya has covered the healthcare industry since 2012. She currently covers the virtual healthcare landscape, including telehealth, remote patient monitoring and digital therapeutics.