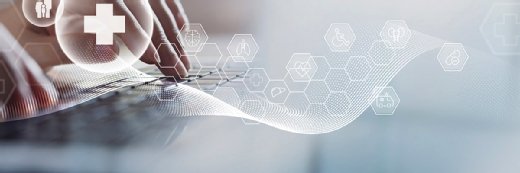
tippapatt - stock.adobe.com
AI approach pinpoints long COVID care needs among kids
Research describes a machine learning approach to identify the diverse healthcare needs of pediatric long COVID patients, enabling personalized care.
A new machine learning approach can help healthcare providers identify the varied needs of pediatric patients with long COVID, thereby enhancing clinical decision-making and enabling personalized treatment, according to recent research.
Published in Cell Patterns, the research described the development of a new ML approach to evaluating the healthcare needs of pediatric patients with long COVID, i.e., post-acute sequelae of SARS-CoV-2 infection (PASC). Long COVID is a chronic condition after a COVID-19 infection, manifesting in a wide range of symptoms lasting at least three months. The symptoms include fatigue, coughing, chest pain, headaches, brain fog and diarrhea.
In 2022, 6.4% of U.S. adults and 1.3% of children had experienced long COVID.
For the study, researchers from the Perelman School of Medicine at the University of Pennsylvania created a latent transfer learning framework (Latent-TL), an ML technique, to study the de-identified EHR data of long COVID patients from eight pediatric hospitals. The researchers analyzed data for 432,165 individuals, of which 49,430 had been infected with COVID-19.
The ML approach included conducting a multisite latent class analysis to identify the multimorbidity patterns and characterize subpopulations among long COVID patients with chronic conditions. It was followed by implementing a transfer causal learning framework to estimate the subpopulation-specific effects of COVID-19 on healthcare utilization.
The researchers pinpointed four subpopulations with distinct clinical needs and chronic conditions within the study population:
- Class 1: Mental health conditions, including anxiety disorder, psychological symptoms, attention-deficit hyperactivity disorder and autism spectrum disorder.
- Class 2: Atopic/allergic chronic conditions, which encompasses patients affected by atopic conditions, such as allergies and asthma.
- Class 3: Noncomplex chronic conditions, comprising patients with noncomplex chronic ailments, such as vision issues.
- Class 4: Complex chronic conditions, which include patients with complex conditions, such as heart or neuromuscular disorders.
The researchers further found that COVID-19 has a varied impact on inpatient and emergency department visits across patient subpopulations and hospitals. For instance, patients with mental health and complex chronic conditions had an increased probability of an ED visit after COVID-19 infection in one hospital, while in another, those with noncomplex and complex chronic conditions saw a significant rise in ED visits.
"Without identifying these distinct subpopulations, clinicians and hospitals would likely provide a one-size-fits-all approach to follow-up care and treatment," said study lead author Qiong Wu, Ph.D., formerly a post-doctoral researcher at the University of Pennsylvania and currently assistant professor of biostatistics at the University of Pittsburgh School of Public Health, in a press release. "While this unified approach might work for some patients, it may be insufficient for high-risk subgroups who require more specialized care. For example, our study found that patients with complex chronic conditions experience the most significant increases in inpatient and emergency visits."
Thus, the researchers concluded that the ML approach could offer "more nuanced, data-informed insights for patient care and management."
Research increasingly shows that ML tools and approaches can be applied to improve healthcare delivery in various ways, including supporting personalized care.
For instance, a study published in 2023 described an ML model that provided personalized treatment recommendations for hypertension and assisted clinicians with choosing the best hypertensive medication for their patients' needs.
The model uses patient-specific characteristics, such as medical history, demographics and test results from EHRs, to provide clinicians with a list of suggested medications, along with the associated probability of success of each drug for the patient.
Anuja Vaidya has covered the healthcare industry since 2012. She currently covers the virtual healthcare landscape, including telehealth, remote patient monitoring and digital therapeutics.