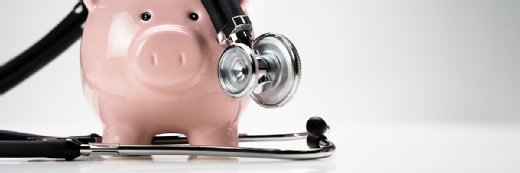
AndreyPopov/istock via Getty Ima
Study reveals cost-efficiency strategy for LLM deployment
Efficient deployment of large language models at scale in healthcare settings could streamline clinical workflows and reduce costs up to 17-fold without sacrificing reliability.
Researchers from the Icahn School of Medicine at Mount Sinai have identified avenues for cost-effective large language model deployment at health system scale, according to a recent Npj Digital Medicine study.
The research team emphasized that LLMs have shown promise for optimizing clinical workflows, but that the computational and economic costs of AI adoption present challenges for healthcare organizations looking to utilize these tools at scale.
To explore how stakeholders might mitigate these hurdles, the researchers assessed 10 LLMs of varying capacity and size using real-world patient data. To evaluate model performance, each tool was presented with chained queries and multiple clinical notes of increasing complexity.
Outputs were measured in terms of accuracy, formatting quality and adherence to clinical instructions. From there, an economic analysis was conducted.
"Our study was motivated by the need to find practical ways to reduce costs while maintaining performance so health systems can confidently use LLMs at scale. We set out to 'stress test' these models, assessing how well they handle multiple tasks simultaneously, and to pinpoint strategies that keep both performance high and costs manageable," said first author Eyal Klang, M.D., director of the Generative AI Research Program in the division of data-driven and digital medicine (D3M) at Icahn Mount Sinai, in a press release.
The research team ran over 300,000 experiments to test the LLMs, revealing that performance deteriorated as the number of clinical notes and queries increased. High-capacity models had the most success, with Meta's Llama-3-70B model achieving high accuracy and low failure rates.
GPT-4 Turbo 128k showed similar results, but saw performance dips after 50 tasks with large prompt sizes.
"Recognizing the point at which these models begin to struggle under heavy cognitive loads is essential for maintaining reliability and operational stability. Our findings highlight a practical path for integrating generative AI in hospitals and open the door for further investigation of LLMs' capabilities within real-world limitations," explained co-senior author Girish N. Nadkarni, M.D., the Irene and Dr. Arthur M. Fishberg Professor of Medicine at Icahn Mount Sinai, director of The Charles Bronfman Institute of Personalized Medicine and chief of the D3M.
The findings further revealed that LLMs can generally handle groups of up to 50 clinical tasks -- including identifying patients eligible for preventive health screenings, reviewing medication safety, extracting data for epidemiological studies, matching patients for clinical trials and structuring research cohorts -- simultaneously without significant drops in accuracy.
The economic analysis showed that by grouping tasks in this way, health systems could streamline workflows and reduce application programming interface (API) costs up to 17-fold, potentially saving larger organizations millions of dollars annually.
The researchers noted that their findings could inform strategies to help health systems efficiently integrate advanced AI technologies to automate tasks.
"This research has significant implications for how AI can be integrated into health care systems. Grouping tasks for LLMs not only reduces costs but also conserves resources that can be better directed toward patient care," stated co-author David L. Reich, M.D., chief clinical officer of the Mount Sinai Health System. "And by recognizing the cognitive limits of these models, health care providers can maximize AI utility while mitigating risks, ensuring that these tools remain a reliable support in critical health care settings."
Moving forward, the research team plans to study how these LLMs perform in clinical environments and test emerging models to determine whether their cognitive thresholds shift as AI technology advances.
Shania Kennedy has been covering news related to health IT and analytics since 2022.