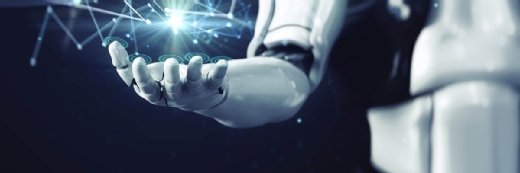
Getty Images
Medical testing inequities contribute to racial bias in AI
Inequitable lab testing to diagnose severe disease can create racial biases in the data used to train healthcare AI, potentially perpetuating care disparities.
Racial disparities in diagnostic tests for severe diseases could create a mechanism for bias in AI-enabled clinical decision-making, according to recent research published in PLOS Global Public Health.
The study, alongside work presented at the 2024 International Conference on Machine Learning, demonstrates that Black patients are significantly less likely to receive medical testing for conditions like sepsis than their white counterparts.
These testing inequities create racial biases in the clinical data often used to train healthcare AI, making these tools more likely to underestimate illness in Black populations. The use of these models in clinical decision support systems can exacerbate existing health disparities, making tackling AI bias a major priority for health systems.
The PLOS study revealed that -- in two cohorts from Michigan Medicine and Beth Israel Deaconess Medical Center in Boston -- when patients were matched for sex, age, medical complaints and emergency department triage scores, white patients received medical testing at a 4.5% higher rate than Black patients.
The research team indicated that this bias is likely partially the result of hospital admission rates, as Black patients were less likely to be assessed as ill and admitted to the hospital than white patients. Accounting for these biases when training AI tools enables them to make more equitable, accurate predictions.
"If there are subgroups of patients who are systematically undertested, then you are baking this bias into your model," said corresponding author Jenna Wiens, Ph.D., associate professor of computer science and engineering at the University of Michigan, in a press release.
"Adjusting for such confounding factors is a standard statistical technique, but it's typically not done prior to training AI models. When training AI, it's really important to acknowledge flaws in the available data and think about their downstream implications," Wiens continued.
To correct for biases in AI, developers could omit patient records to create a less biased data set. In practice, this could look like only including records from patients who received diagnostic medical tests, but the researchers noted that this could make a model trained on that data inaccurate for less ill patients.
Instead, the research team set out to address the bias without omitting patient records. To do so, they developed a computer algorithm designed to identify whether untested patients were likely ill based on factors such as vital signs and race.
While the use of race in clinical algorithms is being reexamined, it was kept as a variable in this research because patients identified as Black in the data set were more likely to be affected by the medical testing bias.
The algorithm was then tested with a simulated data set, in which a known bias was introduced by reclassifying patients originally labeled as ill as "untested and healthy" instead. This data set was used to train a machine learning (ML) model.
When the known bias was corrected using the computer algorithm, ML could effectively differentiate between patients with sepsis and those without in roughly 60% of cases. Without using the algorithm, ML performance dipped significantly.
The accuracy achieved using the computer algorithm to correct for biased data was similar to that of ML trained on simulated, unbiased data.
"Approaches that account for systematic bias in data are an important step towards correcting some inequities in healthcare delivery, especially as more clinics turn toward AI-based solutions," said first author Trenton Chang, a University of Michigan doctoral student in computer science and engineering.
Shania Kennedy has been covering news related to health IT and analytics since 2022.