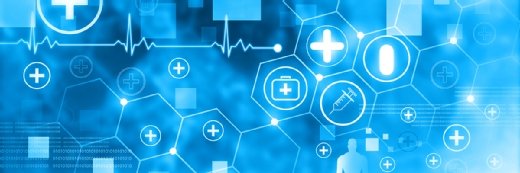
Rasi Bhadramani/istock via Getty
AI-enabled wearable helps detect medication errors
A wearable, deep learning-driven camera could help healthcare providers proactively identify potential errors prior to medication delivery with 99% accuracy.
University of Washington researchers have developed an AI-enabled wearable camera designed to automatically detect potential clinical medication errors before medication delivery, per a recent Npj Digital Medicine study.
Drug-related errors -- such as syringe and vial swap errors -- are a significant driver of preventable patient harm, especially in operating rooms, intensive care units and emergency rooms. The research team emphasized that drug administration errors are the most common critical incidents in anesthesia.
These errors often occur during intravenous injections. Before administering the medication to the patient, clinicians must transfer it from a vial to a syringe. The researchers underscored that roughly a fifth of errors are related to substitution, in which a syringe is mislabeled or the incorrect vial is selected. A similar number of errors happen when a drug is labeled correctly, but administered incorrectly.
Safeguards -- including barcode systems that read and confirm a vial's contents -- exist, but these tools add an additional step to clinicians' workflows, making it possible for a provider to forget to check the barcode system during a high-stress situation.
To address this, the researchers sought to create a tool that could integrate more seamlessly to prevent medication errors. They began by building a deep learning model capable of identifying drug labels on syringes and vials during drug preparation events. Then, the approach was paired with a GoPro camera in an effort to create a real-time warning system for potential medication errors.
The system was trained using 4K video data across 2 hospitals, 17 operating rooms and 13 anesthesiology providers over a period of 55 days. The footage captured providers managing syringes and vials of various medications, which were later labeled to help the algorithm learn to recognize them in videos and images.
Training the model presented a unique challenge, as lighting and setups varied across operating rooms. Further, the system must be able to determine the contents of a vial or syringe that might not be clearly presented to the camera.
"It was particularly challenging, because the person in the [operating room] is holding a syringe and a vial, and you don't see either of those objects completely. Some letters (on the syringe and vial) are covered by the hands. And the hands are moving fast. They are doing the job. They aren't posing for the camera," explained Shyam Gollakota, Ph.D., a co-author of the paper and professor at the University of Washington's Paul G. Allen School of Computer Science & Engineering, in a press release.
Instead of directly reading the words on the vials, the tool scans for other visual cues, such as label print size, the size and shape of each vial or syringe and vial cap color. The model is also able to differentiate between medications in the foreground and ignore those in the background.
Following training, the algorithm was then assessed on videos of 418 drug draws conducted during routine care. The model achieved 99.6% sensitivity and 98.8% specificity at detecting vial swap errors.
The research team concluded that these results demonstrate the wearable camera's potential to reduce medication errors and promote patient safety.
"The thought of being able to help patients in real time or to prevent a medication error before it happens is very powerful," said Kelly Michaelsen, M.D., Ph.D, co-lead author and assistant professor of anesthesiology and pain medicine at the University of Washington School of Medicine. "One can hope for a 100% performance but even humans cannot achieve that. In a survey of more than 100 anesthesia providers, the majority desired the system to be more than 95% accurate, which is a goal we achieved."
Shania Kennedy has been covering news related to health IT and analytics since 2022.