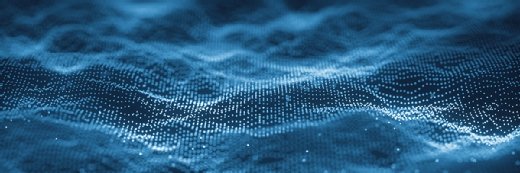
Nabugu - stock.adobe.com
LLMs show potential for hospital quality measure reporting
Large language models can process Severe Sepsis and Septic Shock Management Bundle quality measures in agreement with manual reporting in 90% of cases.
Large language models, or LLMs, can accurately process hospital quality measures efficiently and with high accuracy, according to the results of a pilot reported in the New England Journal of Medicine AI.
The researchers underscored that while hospital quality measures are key to assessing the efficacy and safety of patient care, these metrics can be inconsistent and costly to report. At the crux of this challenge is abstraction of patient charts, or how effectively information is pulled from EHRs and other sources to inform quality measure performance.
Much of this process is manual, taking human abstractors days or weeks to complete. The research team emphasized that LLMs have demonstrated promise in healthcare-related natural language processing tasks -- such as medical chatbot development and the creation of web-based symptom checkers -- but that the potential of these tools in quality measure reporting is largely unknown.
To close this gap, the researchers deployed an LLM-based system designed to ingest FHIR data reported by the University of California San Diego Health to CMS in 2022.
The tool was trained using a sample of 100 manual Severe Sepsis and Septic Shock Management Bundle (SEP-1) abstractions, a complex quality measure that traditionally requires multiple manual reviewers to conduct a 63-step evaluation process.
Streamlining this process could result in significant efficiency boosts for health systems.
"The integration of LLMs into hospital workflows holds the promise of transforming health care delivery by making the process more real-time, which can enhance personalized care and improve patient access to quality data," explained Aaron Boussina, Ph.D., lead study author and postdoctoral scholar at UC San Diego School of Medicine, in a press release. "As we advance this research, we envision a future where quality reporting is not just efficient but also improves the overall patient experience."
The study revealed that the LLM system could accurately process SEP-1 measures, achieving agreement with manual reporting in 90% of cases. Upon review of the discordant cases, the research team found that four were the result of mistakes introduced via the manual abstraction process.
Further, the findings suggest that the LLM system can bolster efficiency by correcting errors, speeding up processing time, and automating aspects of the process that could lead to reduced administrative costs at scale.
Moving forward, the researchers plan to validate the study's findings and use those results to enhance quality measure reporting methods.
"We remain diligent on our path to leverage technologies to help reduce the administrative burden of health care and, in turn, enable our quality improvement specialists to spend more time supporting the exceptional care our medical teams provide," said Chad VanDenBerg, study co-author and chief quality and patient safety officer at UC San Diego Health.
Shania Kennedy has been covering news related to health IT and analytics since 2022.