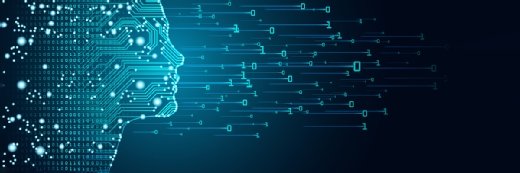
Getty Images
ML helps tailor AHA-PREVENT risk stratification tool
Using open source machine learning tools, researchers recalibrated the AHA-PREVENT calculator to improve cardiovascular risk assessment in local patient populations.
A team from Brigham and Women's Hospital used machine learning to improve the accuracy of the American Heart Association's Predicting Risk of Cardiovascular Disease Events calculator when applied to local populations, per a recent study in JAMA Cardiology.
The AHA-PREVENT model, designed to predict the likelihood of an individual aged 30-79 experiencing a heart attack, heart failure or stroke over a 10-year and 30-year period, has performed well when tasked with assessing risk in national cohorts since its release in 2023.
However, risk calculators that perform well at the national level can deteriorate when adapted for use in local populations, sacrificing accuracy and utility.
"Risk calculators are incredibly important as they are an integral part of the conversation between providers and patients on risk prevention," explained first author Aniket Zinzuwadia, MD, a resident physician in Internal Medicine at Brigham and Women's Hospital, in a press release. "But sometimes, when applying these global calculators to local populations, there is variability inherent to the nature of an area -- whether that is different demographic characteristics, different physician practice patterns, or different risk factors -- so we wanted to find a way to tailor the foundational cardiovascular disease risk model to local populations in a safe way that builds upon what is already being done."
To determine whether the PREVENT model could be effectively adapted for local populations, the researchers turned to an interpretable, machine learning-based method to recalibrate the tool.
The research team pulled EHR data for 95,326 Mass General Brigham patients aged 55 or older in 2007 with at least one recorded lipid or blood pressure measurement in the previous decade and one encounter with the hospital from 2007 to 2016.
Using the open source ML tool known as XGBoost, the researchers recalibrated the PREVENT equations for use in a local population with minimal predictor variables -- AHA-PREVENT, age and sex. This allowed the team to tailor the risk calculator for local use while preserving known associations between risk factors and cardiovascular events.
An analysis of the recalibrated PREVENT calculator showed higher accuracy overall in the Mass General Brigham cohort than the original AHA-PREVENT, reclassifying one in 10 patients into a different risk category.
"This could theoretically represent a group of patients that might not have been prescribed statin therapies in the original application of the model, for example, but who might have benefited from them," Zinzuwadia stated.
The researchers emphasized that their AI-driven risk stratification approach requires further validation before it could be deployed to inform patient care, but noted that in the future, they would like to see the technique applied to other health systems' populations.
"A major challenge of applying AI to medical research is ensuring that machine learning models are not just flexible, but also transparent, reliable, and grounded in domain knowledge," said co-senior author Olga Demler, Ph.D., an associate biostatistician at Brigham and Women's Hospital's division of preventive medicine. "Our approach shows that it is possible to avoid the 'black box' nature of AI applications and may offer a path forward where sophisticated algorithms can retain their flexibility while producing guarantees of their performance."
Shania Kennedy has been covering news related to health IT and analytics since 2022.