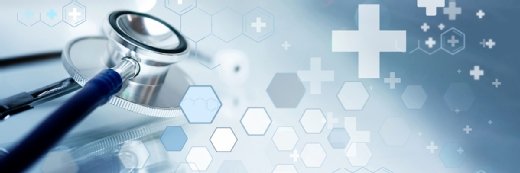
Baurzhan Ibrashev/istock via Get
AI foundation model predicts cancer diagnosis, prognosis
A ChatGPT-like AI model could inform precision medicine efforts across 19 cancer subtypes by analyzing histopathology images and predicting the molecular profile of tumors.
A pathology-based AI foundation model can accurately perform a host of diagnostic and prognostic tasks across multiple forms of cancer, per a recent Nature study.
The model -- Clinical Histopathology Imaging Evaluation Foundation (CHIEF) -- was designed to address current gaps plaguing AI tools for cancer diagnosis. These approaches are typically built to perform specific tasks, like predicting the genetic profile of a tumor or detecting cancer presence, but often only work for certain cancer types.
Harvard Medical School (HMS) researchers built CHIEF to perform a variety of these tasks across 19 different types of cancer, making it potentially more generalizable than existing AI models.
"Our ambition was to create a nimble, versatile ChatGPT-like AI platform that can perform a broad range of cancer evaluation tasks," said study senior author Kun-Hsing Yu, MD, Ph.D., assistant professor of biomedical informatics in the Blavatnik Institute at HMS, in a press release. "Our model turned out to be very useful across multiple tasks related to cancer detection, prognosis, and treatment response across multiple cancers."
The foundation model reads pathology slides of tumor tissues, and uses these to derive insights about the cancer cells and molecular profile of the sample. By pinpointing relevant features in the tumor microenvironment, the tool can shed light on how a patient is likely to respond to standard treatments and forecast patient survival.
The research team indicated that CHIEF might also be capable of surfacing new precision medicine insights, including previously unknown links between specific tumor characteristics and patient survival.
Trained on 15 million unlabeled histopathology images categorized based on image sections of interest and 60,000 whole-slide images of various tissues, CHIEF's approach allows users to interpret imaging more holistically than other methods by taking both regions of interest and the whole image into account.
The tool was tested on over 19,400 whole-slide images pulled from 32 data sets from an international pool of health systems and patient cohorts.
When compared to other AI models, CHIEF achieved high performance across a variety of tasks, data sets and cancer types. The tool performed well regardless of how the tumor cell samples were obtained or digitized.
CHIEF significantly outperformed the other AI systems by up to 36% when tasked with detecting cancer cells, identifying tumor origin, predicting patient outcomes and flagging the presence of DNA and genes linked to treatment response.
The foundation model achieved 94% accuracy in cancer detection overall across 15 data sets and 11 cancer types.
The researchers emphasized that CHIEF's performance demonstrates its potential for use in different clinical settings and precision medicine applications.
Moving forward, the team plans to further improve the model by training it on additional images of non-cancerous and rare conditions, exposing it to additional molecular data to help it identify more aggressive levels of cancer and teaching it to predict the adverse effects and benefits of standard and emerging cancer treatments.
"If validated further and deployed widely, our approach, and approaches similar to ours, could identify early on cancer patients who may benefit from experimental treatments targeting certain molecular variations, a capability that is not uniformly available across the world," Yu stated.
Shania Kennedy has been covering news related to health IT and analytics since 2022.