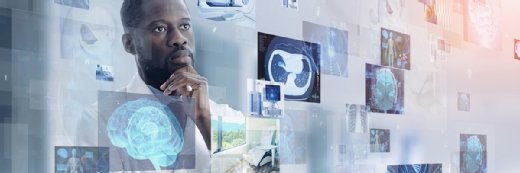
Getty Images/iStockphoto
Anatomy-aware GenAI shows promise in medical imaging
An anatomy-aware generative AI tool could improve the synthesis of high-quality computed tomography images for research and clinical applications.
A Boston University-led research team has developed an anatomy-aware generative AI tool capable of synthesizing high-quality, 3D computed tomography images using textual information.
The researchers emphasized that AI-powered image generators are transforming various industries, including healthcare. In the context of medical imaging, GenAI tools have the potential to help clinicians interpret complex X-rays, diagnose conditions and track disease .
However, many image generators are trained on foundation models -- a type of machine learning model trained on broad data sets to be fine-tuned and adapted for various applications. Using the foundation model framework, image generators can then analyze patterns in their training data to create new images.
However, GenAI tools are prone to hallucinations, which cause them to generate misleading or false information. In medical imaging, such hallucinations could lead to delayed treatment and incorrect diagnoses, which means overcoming such hurdles is crucial for the use of generative AI in healthcare.
The research team further emphasized that foundation model-driven image generators often provide low-resolution outputs that do not effectively utilize the wealth of data found in radiology reports.
To tackle these challenges, the researchers built MedSyn, a publicly available, anatomy-aware GenAI tool designed to produce high-fidelity chest CT scans using text prompts. MedSyn was trained on more than 9,000 volumetric chest CTs and 200,000 deanonymized radiology reports, making it an organ-specific, multimodal approach, unlike traditional foundation models.
The approach allows for the incorporation of radiology report data, enabling the tool to aid users with a variety of tasks, such as interpreting complex abnormalities in medical images.
"While the trend for foundational models has taken a one-size-fits-all approach, we don't believe that is the best practice for medical imaging," said corresponding author Kayhan Batmanghelich, Ph.D., assistant professor of engineering and junior faculty fellow of Hariri Institute for Computing at Boston University, in a press release. "Pathological changes to anatomy can occur years before clinical evidence of disease. For interventions to have the most impact, we need sophisticated AI models that can detect abnormalities that are often elusive in the earliest stages of disease progression."
The information pulled from radiology reports -- such as pathology and anatomical location -- can help users generate more refined images for data augmentation in clinical research. This capability is important for studies that require large samples of hard-to-acquire images, such as lung scans.
"Lung CT scans exhibit more challenging details compared to other organs," explained Batmanghelich. "By specifically fine-tuning the AI language model to the language used in radiology reports and incorporating constraints that enforce standard anatomical positions consistent with the human body, our model can synthesize images with a level of granularity, even when presented with small, hard-to-see anatomical details."
The researchers also underscored that MedSyn is a type of explainable AI, which could help stakeholders navigate concerns about black box healthcare AI.
"Since the data set for MedSyn is derived from CAT scans and radiology reports, the output is fully transparent and as interpretable as a radiology report," Batmanghelich stated. "The tool not only empowers clinicians but researchers can use this method as a building block in their research.
The research -- a collaboration among researchers from Boston University, Carnegie Mellon University, the University of Pittsburgh and Stanford University -- is just one of many promising applications of generative AI in healthcare.
"Our research takes a significant step toward demonstrating the capability and potential impact of building anatomically-specific foundational models, models that know about anatomy and know about the macros and micros structure that is changing the anatomy," Batmanghelich noted. "These next-generation models deliver the accuracy, reliability and interpretability that clinicians and researchers require, and that the healthcare sector requires, for AI to realize its transformative potential."
Shania Kennedy has been covering news related to health IT and analytics since 2022.