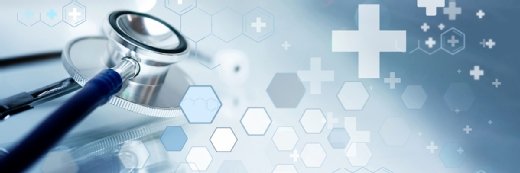
Baurzhan Ibrashev/istock via Get
Deep learning shows promise in predicting osteoporosis risk
A deep neural network significantly outperformed existing machine learning and regression models for osteoporosis risk prediction in a cohort of over 8,000 participants.
A deep learning tool developed at Tulane University outperformed five algorithms designed to predict osteoporosis risk, according to a recent study published in Frontiers in Artificial Intelligence.
Osteoporosis -- characterized by low bone mineral density (BMD) and bone tissue deterioration -- is considered a silent disease because many patients with the condition do not experience symptoms. However, osteoporosis-related bone fragility is a major cause of fracture and disability, particularly in older adults.
Mitigating these adverse outcomes depends on accurately stratifying patient risk and effectively diagnosing at-risk individuals. However, early diagnosis of osteoporosis remains a major public health challenge because the gold standard diagnostic tool for the disease, dual-energy X-ray absorptiometry, is costly and largely inaccessible to many.
To combat this, researchers have worked to build algorithms for predicting osteoporosis risk using routinely collected demographic and clinical data. Currently, many of these models have demonstrated low accuracy, leading the Tulane team to evaluate whether a deep neural network (DNN) could improve predictive performance.
The model was built using a combination of extensive demographic, clinical and BMD data from 8,134 individuals over 40 pulled from the "Louisiana Osteoporosis Study." From there, the DNN was compared to four machine learning approaches -- random forest (RF), artificial neural network, k-nearest neighbor and support vector machine -- and one regression model, known as the osteoporosis self-assessment tool, in an analysis of 16 variables.
Each tool's performance in predicting osteoporosis risk was assessed in terms of area under the curve (AUC) and various accuracy metrics.
The DNN was more effective at classifying osteoporosis compared to the other approaches, reaching an AUC of 0.848, a sensitivity of 0.740 and a specificity of 0.793. The DNN's predictive accuracy was 0.753, surpassed only by the RF model at 0.757.
Further, the analysis revealed 10 key factors for predicting osteoporosis risk: weight, age, gender, grip strength, height, beer drinking, diastolic blood pressure, alcohol drinking, smoking years and income.
These findings indicated that the DNN model yielded the best predictive performance overall, presenting opportunities to facilitate early diagnosis of osteoporosis.
"The earlier osteoporosis risk is detected, the more time a patient has for preventative measures," said lead author Chuan Qiu, MD, Ph.D, a research assistant professor at the Tulane School of Medicine Center for Biomedical Informatics and Genomics, in a press release. "We were pleased to see our DNN model outperform other models in accurately predicting the risk of osteoporosis in an aging population."
Despite the success of their model, the researchers cautioned that more work to refine and validate AI-based risk prediction tools is required before they can be deployed in clinical settings to stratify individual patient risk.
"Our final aim is to allow people to enter their information and receive highly accurate osteoporosis risk scores to empower them to seek treatment to strengthen their bones and reduce any further damage," Qiu noted.
Shania Kennedy has been covering news related to health IT and analytics since 2022.