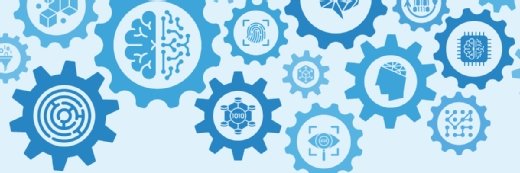
Getty Images
How Health Information Exchange Models Impact Data Analytics
How are health information exchange models structured? How can providers use them for data analytics and population health management?
Health information exchange is both a verb and a noun, and it’s hard to have one without the other. While HIE can be as simple as sending a secure Direct message to a specialist or connecting several local providers through their EHRs, large-scale health information exchange requires a bit more technical development.
What are the three primary infrastructure models for health information exchanges, and how do they affect participation in population health management and data analytics for providers?
The centralized model
The centralized HIE model connects a number of payer, provider, and public health data sources through a single common data pool. Individual organizations feed into the aggregated clinical data repository, which can then respond to queries from those providers. The centralized model is simple, but it is also highly effective for aggregating information from multiple sources, since all data is being held in a consolidated data warehouse that is managed by a strong central authority.
This model can be effective for community-level data analytics and population health management since all information from the participating region is being held in the same place. However, there are certain limitations to this architecture. Health information exchange is not always easy to accomplish, since the centralized model is better at gathering data than pushing it back into the provider ecosystem. The HIE authority itself may perform data analytics on the information it holds, but providers are more likely to receive static reports from the HIE rather than be able to perform their own analytics on the fly.
Additionally, without stringent data governance guidelines, the centralized model is prone to suffering from duplicated records, especially if all participating organizations do not share a single system of identifying and tagging patient data. Centralized models may work best in areas where healthcare providers are largely users of a single HIE vendor or are especially savvy about adopting technologies that rely on industry-wide data standards.
Health information exchange organizations based on the centralized model have seen success on the regional and state level. Of the seven Beacon Community Program participants, three use HIE models based on a centralized infrastructure, including HealthInfoNet in Maine, INHS Health Information Network serving parts of Washington State and Idaho, and MyHealth Access Network in Oklahoma. All of these communities include population health management and data analytics as part of their core missions, and have successfully used centralized HIE to provide reporting that can help providers improve quality of care and patient outcomes.
The federated or decentralized model
The decentralized or federated model for health information exchange eschews the single data warehouse strategy all together. Data stays at its source, giving healthcare providers control over their own patient information. Participation requires an agreement on the part of the provider to allow other organizations access to their data, which has both its benefits and drawbacks.
On the plus side, this prevents some of the potential problems with privacy and security that may arise from keeping data in one large pool. It also removes the question of data ownership that has been a significant stumbling block for some health information exchanges looking to connect fiercely protective participants. As healthcare data becomes an increasingly valuable business asset to providers competing for market share and patient loyalty, the question of ownership can derail negotiations quickly.
A decentralized model also ensures that the entire structure does not collapse if one healthcare provider experiences a technical difficulty. Only a small number of records would be unavailable until the problem can be resolved.
Federated HIEs must employ a record locator service (RLS) in order to connect their participating providers. The RLS manages requests between organizations, acting as a fetch-and-carry messenger for clinical data when a query is received. While this ensures that data is accessed only when necessary, it also presents difficulties in the setup and maintenance of a far-flung, interconnected web of providers with dozens of points of connection.
The hybrid HIE model
The most common structure for a health information exchange combines both the centralized and federated model into a hybrid structure. A centralized data repository is present, as is a record locator service. Both entities work together to ensure that the HIE can track a patient’s movements between participating providers while ensuring that healthcare organizations can receive a minimum clinical data set for each beneficiary. The hybrid model is the choice of the majority of Beacon Community Program participants, and has produced long-term success for many.
Hybrid models, as well as centralized infrastructures, can provide several critical care coordination and population health management tools that are much more difficult to deploy in a fully decentralized system. Admission, discharge, and transfer (ADT) alerts, for example, require a master patient index (MPI) or other matching engine that can coordinate the proper delivery of the reminders without the need for action on the part of an individual provider.
The hybrid structure also facilitates clinical analytics, risk stratification, and medical research by allowing the development of patient cohorts through the data warehouse. Participants can extract data from the centralized repository, add the information to their own EHR data, or track patient outcomes. The centralized model and the hybrid structure are significantly more suited to this type of advanced population health management than a fully decentralized set-up.
Health information exchanges that utilize a hybrid model are among the most flexible, and may be able to enhance participants’ EHR and data analytics capabilities at a more responsive rate than other technologies while supporting the nationwide goals of health IT interoperability, patient engagement, and population health management. While each type of HIE has its pros and cons, the hybrid approach balances the need for provider autonomy with the high impact of the data warehouse for significant results.
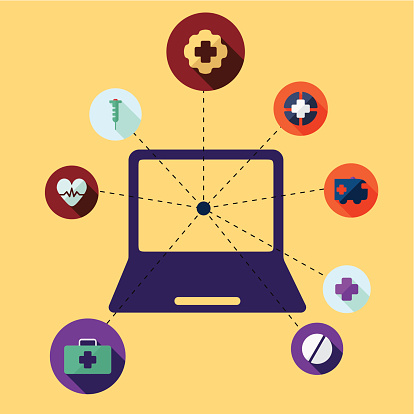