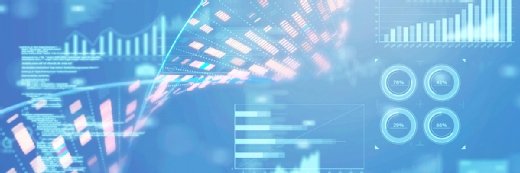
Yuichiro Chino/Moment via Getty
Predictive Analytics Algorithm Displays Bias, Drives Inequity
The predictive analytics algorithm perpetuated some implicit racial bias and health inequity, the UC Berkeley researchers found.
A predictive analytics platform referring high-risk patients to care management programs is infected with the same implicit racial bias that many human decision-makers have, according to new research from the University of California Berkeley, the University of Chicago Booth School of Business, and Partners HealthCare.
Specifically, predictive analytics algorithms are referring healthier white patients to care management programs at higher rates than they are referring less healthy black patients to those same care management programs.
Healthcare organizations, like many other business sectors, are becoming increasingly reliant on predictive analytics algorithms to make key decisions. In the case of this platform, algorithms assess patients based on their risk to refer them to certain care management protocol.
But the basis for the algorithm is flawed, the researchers said, perpetuating the same health disparities that may have contributed to the algorithm’s limitations.
“We found that a category of algorithms that influences health care decisions for over a hundred million Americans shows significant racial bias,” Sendhil Mullainathan, the Roman Family University professor of Computation and Behavioral Science at Chicago Booth and senior author of the study, said in a statement.
The algorithm detects patient risk by assessing the amount of healthcare dollars spent on the patient. But currently health disparities and inequities are skewing those risk assessments to favor white patients who are in many cases healthier, the researchers said.
Cases of implicit bias in other areas of the healthcare industry sometimes lead providers to deliver more care to these white patients, meaning more healthcare dollars are spent on those white patients.
And because these risk algorithms look at healthcare dollars spent, it will ultimately favor those healthier white patients.
“The algorithms encode racial bias by using health care costs to determine patient ‘risk,’ or who was mostly likely to benefit from care management programs,” said Ziad Obermeyer, acting associate professor of health policy and management at UC Berkeley and lead author of the paper.
“Because of the structural inequalities in our health care system, blacks at a given level of health end up generating lower costs than whites,” Obermeyer added. “As a result, black patients were much sicker at a given level of the algorithm’s predicted risk.”
The researchers detected these inequities by comparing the algorithm’s current risk analysis with an analysis of other markers of health risk, such as number of chronic illnesses treated in a year, avoidable cost, or other biomarkers.
The algorithm currently refers patients in the 97th risk percentile to care management programs, the research team reported. When adjusting the algorithm for those new markers of health risk, the number of black patients referred to care management programs increased from 18 percent to 47 percent of all patients.
“Algorithms can do terrible things, or algorithms can do wonderful things. Which one of those things they do is basically up to us,” Obermeyer said. “We make so many choices when we train an algorithm that feel technical and small. But these choices make the difference between an algorithm that’s good or bad, biased or unbiased. So, it’s often very understandable when we end up with algorithms that don’t do what we want them to do, because those choices are hard.”
The good news is, it is possible to fix these algorithm flaws, the researchers said. For one thing, Obermeyer reported that one of the software manufacturers that uses this algorithm in its predictive analytics platform was extremely responsive when alerted to the bias.
“For algorithms, just as for medicine,” he said, “we’d prefer to prevent problems, instead of curing them.”
Other manufacturers using the same algorithm should likewise look into its use and identify solutions that would bring about more Health equity.
“Algorithms by themselves are neither good nor bad,” Mullainathan said. “It is merely a question of taking care in how they are built. In this case, the problem is eminently fixable — and at least one manufacturer appears to be working on a fix. We would encourage others to do so.”