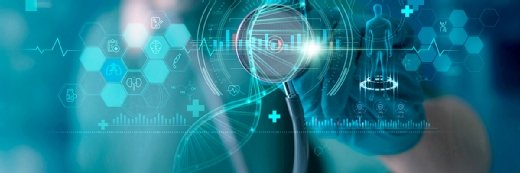
ipopba/istock via Getty Images
Machine Learning Uses Social Determinants Data to Predict Utilization
A machine learning tool was able to predict inpatient and emergency department utilization using only social determinants of health data.
A machine learning algorithm accurately predicted inpatient and emergency department (ED) utilization using only publicly available social determinants of health (SDOH) data, showing that it’s possible to determine patients’ risk of utilization without interacting with the patient or collecting information beyond age, gender, race, and address.
That’s the major finding of a study recently published in the American Journal of Managed Care.
By now, the healthcare industry is well aware of the connection between the conditions in which someone lives and works and her physical health. The researchers note that sociodemographic status, racial and ethnic disparities, and individual behaviors directly correlate with an increase in the prevalence and incidence of chronic diseases.
Healthcare organizations have made a concentrated effort to reduce health inequalities and address social needs, but this can be challenging.
“Current analyses, predictive models, and prevention initiatives focus on addressing SDOH at the population level or the zip code level. The shortcoming of this approach is a gap in addressing the individual patient’s needs, such as defining clinical action steps that are relevant to the patient as opposed to an overall population approach,” researchers said.
“Advancements in cognitive science allow for the analysis of individual contributions of SDOH at the patient level, informing appropriate interventions that can reduce the risk of negative health outcomes such as preventable readmissions and/or hospitalizations.”
The team aimed to use machine learning to predict utilization independent of a patient’s clinical condition, while establishing which determinants contribute to the greatest risk of utilization. Researchers selected 138,115 patients from a deidentified database representing three health systems in the US. The group then split patients into training and testing sets.
The machine learning tool principally measured any inpatient/ED utilization in 90 days, as well as secondary outcomes of inpatient admission, avoidable admission, and ED visit.
The results showed that the machine learning tool was able to predict utilization with a high degree of discrimination. When predicting inpatient/ED utilization, the algorithm achieved an area under the curve (AUC) of 0.84 in the training set and 0.83 in the testing set. For the secondary outcomes, the algorithm achieved an AUC ranging from 0.78 to 0.84.
The results also showed that the social determinant most associated with risk was air quality, which had a relative value more than twice that of income, which was the second determinant most associated with risk. Both air quality and income were more important to the decision-making ability of the model than age, gender, or ethnicity.
“This study demonstrates that it is possible to generate a highly accurate model to predict inpatient and ED utilization using decision tree–based machine learning with purchasable and publicly available data on the social determinants of health,” the team stated.
“All the data used in the analysis are available without collecting information directly from patients. Therefore, this study indicates that it is possible to risk-stratify patients’ risk of utilization without interacting with the patient or collecting information beyond the patient’s age, gender, race, and address.”
The machine learning approach was able to identify the relative value of multiple SDOH categories and determine their role in patient risk, which could help providers seeking to improve health at the individual level.
“Addressing the root cause of inequalities in the community runs in parallel with solving nationwide health issues such as food insecurity and limited access to care. Through the Affordable Care Act, nonprofit hospitals are required to conduct community health needs assessments and construct community interventions every three years,” researchers said.
“However, many hospitals and affiliated organizations may lack the resources and competencies to strategically address community health initiatives that commonly fall outside of basic clinical care. Other technologies including EHRs are making advances to collect patient-reported socioeconomic determinants of care.”
The team noted that health systems could target the social determinants of health using the technology and methods outlined in this study.
“A primary care physician may use this as a tool to identify areas on which to focus socioeconomic screening and topics to problem-solve regarding overcoming barriers with patients. A community care coordinator would find this technology useful for outreach programs (e.g., patient follow-up with prescribed diet),” the group stated.
The team expects that the results can inform SDOH initiatives and plans in different kinds of healthcare organizations.
“This study highlights the significant influence of SDOH on individuals’ health and healthcare use. It is an important advancement in tackling disparities in healthcare because risk can be assessed without gathering information directly from the patient and thus can be incorporated efficiently into workflows,” the team concluded.