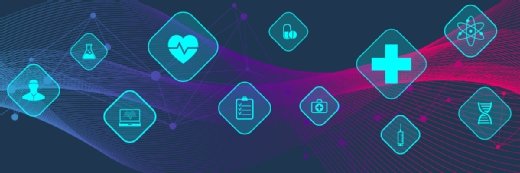
BAIVECTOR - stock.adobe.com
Machine Learning in Drug Development Requires Data Access, Standards
To leverage machine learning tools in drug development, policymakers will need to increase data access and establish uniform data standards.
Machine learning algorithms have the potential to accelerate and refine the drug development process, but the industry should expand data access and create consistent data standards to ensure drug companies can fully leverage these tools, according to a report from the Government Accountability Office (GAO).
Drug companies spend ten to 15 years bringing a drug to market, often at high costs. Only about one in every 10,000 chemical compounds initially tested for drug potential makes it through the research and development pipeline, GAO noted, and is then approved by the FDA for marketing in the US. Machine learning tools could accelerate and improve the drug development process.
“Machine learning can make drug development more efficient and effective, decreasing the time and cost required to bring potentially more effective drugs to market,” GAO said.
“Both of these improvements could save lives and reduce suffering by getting drugs to patients in need more quickly. Lower research and development costs could also allow researchers to invest more resources in disease areas that are currently not considered profitable to pursue, such as rare or orphan diseases.”
Although drug companies already use machine learning throughout the drug development process, there are several challenges that hinder its advancement in this area, including barriers to data access and sharing.
“According to one industry representative, collecting data from the early drug discovery phase can be cost prohibitive. This representative said that certain health-related data may cost tens of thousands of dollars, as compared to just cents for other consumer related data that many technology companies use,” GAO stated.
“Data sharing also presents unique legal issues. According to stakeholders, privacy laws such as HIPAA can make it difficult for drug companies, especially those that are not regulated by HIPAA, to share or access data.”
To increase data sharing and access, GAO recommended that policymakers create mechanisms or incentives to share data held by private or public sectors, while also ensuring patient information is protected.
“To promote greater availability of data, policymakers could consider forming or facilitating research consortia that allow for secure data sharing,” the organization wrote.
“Policymakers could also consider creating a data repository through encouraging an industry-driven solution, establishing a public-private partnership, or creating a repository of all data under their control.”
In creating new ways to share and access data, stakeholders should ensure they adhere to laws around information exchange.
“Improper data sharing or use could have legal consequences. Increased data sharing could therefore require a careful review of the legal ramifications, because data are often gathered through a wide variety of mechanisms and governed by multiple legal frameworks,” GAO advised.
In addition to data sharing and access, policymakers will need to address the lack of quality data in the drug development process.
“Machine learning requires a large amount of accurate and representative data. This poses a unique challenge in drug development, as much of the data were not originally collected with machine learning in mind and may not be machine-readable or model-ready,” GAO wrote.
“Furthermore, according to an industry representative, data collected across different organizations and environments come in different formats, and this lack of standardization in data quality is a barrier.”
Overcoming data quality issues will require policymakers to collaborate with appropriate stakeholders to establish data standards, GAO said.
“For example, a standard that defines synthetic data and how they can be used can help reduce bias by allowing researchers to generate data that could be used to better represent currently underrepresented communities,” the agency stated.
“Similarly, a standard data format for uploading and sharing data across platforms could reduce the need for data scientists to spend time converting data sets to machine-readable formats.”
GAO also named drug development research gaps as an obstacle to machine learning use.
“Research gaps present a significant challenge to advancing the use of machine learning in drug development. These gaps fall into two broad categories: gaps in understanding of fundamental biology and chemistry, and gaps in domain-specific machine learning research,” GAO said.
“Experts in the field have noted that addressing these issues may be transformational for future applications of machine learning in drug development.”
GAO suggested that policymakers promote basic research to generate new and better data to improve understanding of machine learning in drug development.
“Policymakers could promote the field in multiple ways, including approaches such as support for intramural research, grants, or other subsidies. Policymakers could choose to use one of these approaches or combine them,” GAO said.
“Policymakers could also support collaboration across sectors. The Machine Learning for Pharmaceutical Discovery and Synthesis Consortium (MLPDS) is a collaboration between large drug companies such as Pfizer, Merck, and Novartis with the Chemical Engineering, Chemistry, and Computer Science departments at the Massachusetts Institute of Technology, and has published a variety of papers at the intersection of machine learning and drug development.”
With these recommendations, policymakers and other stakeholders can advance the use of machine learning in drug development, refining and speeding the process to benefit patients.