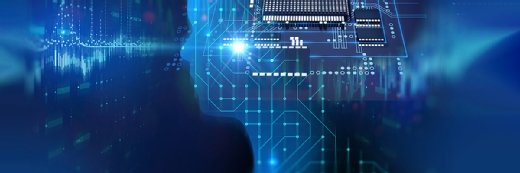
Getty Images
New Method Determines Accuracy of Predictive Risk Models
The technique can help providers assess whether a predictive risk model’s results can be trusted for a given patient.
A team from the Massachusetts Institute of Technology (MIT) has developed a method that determines the accuracy of predictive risk models, helping clinicians to choose better treatments for their patients.
When patients have a heart attack or stroke, providers often use risk models to determine the best treatments. These models can calculate a patient’s risk of dying based on elements such as the patient’s age, symptoms, and other factors. Although these models are useful, they often fail to make accurate predictions for many patients. This can result in clinicians choosing ineffective or risky treatments for some patients.
Together with researchers at the MIT-IBM AI Lab and the University of Massachusetts Medical School, MIT researchers built a method that can determine whether a particular model’s results can be trusted for a given patient.
"Every risk model is evaluated on some dataset of patients, and even if it has high accuracy, it is never 100 percent accurate in practice," said Collin Stultz, a professor of electrical engineering and computer science at MIT and a cardiologist at Massachusetts General Hospital. "There are going to be some patients for which the model will get the wrong answer, and that can be disastrous."
Predictive risk models are often developed using machine learning algorithms, which can sometimes lead to inaccuracies.
"Very little thought has gone into identifying when a model is likely to fail. We are trying to create a shift in the way that people think about these machine-learning models. Thinking about when to apply a model is really important because the consequence of being wrong can be fatal," said Stultz.
High-risk patients who are misclassified could fail to receive aggressive treatment, while low-risk patients who are determined to be at high risk could receive unnecessary and potentially harmful therapies.
Researchers focused on the Global Registry of Acute Coronary Events (GRACE) risk score, a widely used risk score that can be applied to nearly any type of risk model. GRACE is a large dataset that was used to develop a risk model that evaluates a patient’s risk of death within six months of experiencing an acute coronary syndrome. The resulting risk assessment is based on age, blood pressure, heart rate, and other clinical features.
The researchers’ technique generates an “unreliability score” ranging between zero and one. For any given risk prediction model, the higher the score, the more unreliable the prediction.
The unreliability score is based on a comparison of the risk prediction generated by a particular model, like the GRACE risk score, with the prediction generated by a different model that was trained on the same dataset. If the models produce different results, it is likely that the risk model prediction for that patient is unreliable.
"What we show in this paper is, if you look at patients who have the highest unreliability scores -- in the top 1 percent -- the risk prediction for that patient yields the same information as flipping a coin," Stultz said. "For those patients, the GRACE score cannot discriminate between those who die and those who don't. It's completely useless for those patients."
The team also found that patients for whom the models don’t work well tended to be older and had a higher incidence of cardiac risk factors. Researchers were able to develop a formula that determines how much two predictions would disagree without having to build an entirely new model based on the original dataset.
"You don't need access to the training dataset itself in order to compute this unreliability measurement, and that's important because there are privacy issues that prevent these clinical datasets from being widely accessible to different people," Stultz said.
The team is now working on a user interface that providers could use to determine whether a patient’s given GRACE risk score is accurate. Going forward, researchers will also aim to improve the reliability of risk models that make it easier to retrain models on data that include more patients who are similar to the patient getting diagnosed.
"If the model is simple enough, then retraining a model can be fast. You could imagine a whole suite of software integrated into the electronic health record that would automatically tell you whether a particular risk score is appropriate for a given patient, and then try to do things on the fly, like retrain new models that might be more appropriate," Stultz said.