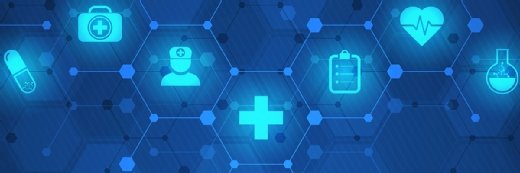
bestbrk/istock via Getty Images
Machine Learning Tool Predicts Hospitalizations for Asthma Patients
A machine learning algorithm was able to forecast hospital encounters among patients with asthma better than current predictive models.
Using machine learning, researchers developed a more accurate model to predict hospital encounters for asthma patients, which could improve asthma outcomes and reduce care costs, according to a study published in JMIR Medical Informatics.
Asthma affects 8.4 percent of the US population, researchers said, and leads to 2.1 million emergency department (ED) visits and 479,300 hospitalizations annually. The condition costs the healthcare system $50.3 billion each year.
Current risk prediction models that identify high-risk asthmatic patients have significant gaps, leading to poorer outcomes, the group stated.
“When used for care management, these models miss over half of the patients who will incur future hospital encounters and incorrectly classify many other patients as patients who will incur future hospital encounters,” researchers said.
“This makes it difficult to align care management enrollment with the patients who will actually incur future hospital encounters, increasing healthcare costs and impairing patient outcomes.”
The team set out to develop a more accurate model to predict hospital encounters among patients with asthma. Researchers built a machine learning model using data from asthmatic patients who visited Intermountain Healthcare facilities between 2005 and 2018.
The new model achieved an area under the curve (AUC) of 0.859. When the cutoff threshold was set at the top ten percent of asthmatic patients with the highest predicted risk, the model reached an accuracy of 90.31 percent, a sensitivity of 53.7 percent, and a specificity of 91.93 percent.
“Our final model achieved a higher AUC than what has been reported in the literature for this task,” researchers said.
“After further refinement to improve its accuracy and to automatically explain its prediction results, our final model could be integrated into an electronic medical record system to guide care management allocation for asthmatic patients. This could better allocate a scarce and expensive resource and help improve asthma outcomes.”
While the model did achieve a higher level of accuracy than previous algorithms, the researchers noted that the new model still reached a relatively low positive predictive value (PPV) of 22.65 percent. However, the team stressed that this doesn’t prevent the model from being clinically useful.
“A PPV of 22.65 percent is reasonably good for identifying high-risk asthmatic patients as candidates for receiving relatively inexpensive preventive interventions,” the team said.
“Four examples of such interventions are teaching the patient how to correctly use an asthma inhaler, teaching the patient how to correctly use a peak flow meter and giving it to the patient to use at home for self-monitoring, training the patient to keep an environmental trigger diary, and arranging for a nurse to make additional follow-up phone calls with the patient.”
The team also pointed out that the study had several limitations, including the fact that they used data from a single healthcare system, which could mean the model won’t produce the same results for other organizations. The team plans to use data from another major health system to evaluate the model’s predictive performance.
“After further refinement, our final model could be integrated into an electronic medical record system to guide allocation of scarce care management resources for asthmatic patients. This could help improve the value equation for asthma care by improving asthma outcomes while also decreasing resource use and cost,” researchers concluded.