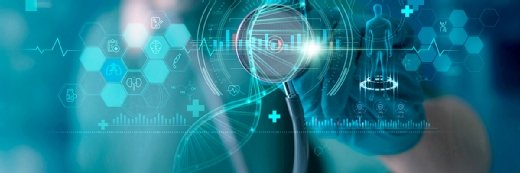
ipopba/istock via Getty Images
Predictive Analytics Model Forecasts Hernia Surgery Outcomes
A predictive analytics tool could determine the likelihood that a patient will experience complications after having hernia surgery.
Researchers at the University of Tennessee, Knoxville, have developed a predictive analytics algorithm that can identify future at-risk patients who have undergone hernia surgery, helping providers to avoid overtreatment and deliver more targeted care.
Abdominal wall hernia repair, including ventral hernia repair, is one of the most common general surgical procedures. At least 350,000 ventral hernia repairs are performed annually in the US, researchers said, and the outcomes of these surgeries are generally reported to be poor. This results in additional surgical repair rates of 12.3 percent at five years, and 23 percent at 10 years.
Overall healthcare costs associated with ventral hernia repair are estimated to be over $3 billion each year.
The research team examined data from 102 patients who had undergone ventral hernia repair surgery. Looking at historical patient data, including demographics and information from the surgical procedures, researchers evaluated patterns that led to complications following surgery.
The resulting predictive model indicates that the emotional status of the patient before surgery, such as levels of depression, anger, grief, or anxiety, impact recovery outcomes. If providers address patients’ emotional issues or fears before surgery, patients may experience less pain afterwards.
"If we begin prehabilitation, which includes a holistic assessment--not limited to physical and emotional condition--of the person prior to the intervention, then we may be able to affect outcomes," said Rebecca Koszalinski, assistant professor in the College of Nursing.
Clinicians could use the predictive model as a clinical decision support tool to more easily assess the risks associated with this kind of surgery, researchers said.
"There is a lot of potential for developing decision support tools using data science and artificial intelligence," said Anahita Khojandi, assistant professor in the Department of Industrial and Systems Engineering in the Tickle College of Engineering.
"We hear about similar models in the news every day, focused on detecting tumors in chest X-rays, among other things. This is an example of how a tool can be used for shared decision-making and change how individuals interact with the healthcare system."
Physicians, nurse practitioners, and other providers could leverage the tool to simulate different scenarios for different patients and evaluate how the risk factors change among individuals. The model could help clinicians avoid overtreatment, direct efforts on patient education, and examine the impact that lifestyle changes have on patients.
"I focus on the person and how they may be better informed and empowered to share in decision-making," Koszalinski said. "The hope is that predictive modeling coupled by empowered patients and expert clinical professionals could result in optimal patient outcomes."
Predictive analytics tools have previously uncovered links between mental wellness and physical health outcomes. In 2018, Kaiser Permanente announced the launch of a three-year, $1.3 million study to examine the relationship between opioid abuse and suicide risk.
“We’ve done preliminary work suggesting that 22 to 37 percent of opioid-related overdoses are, in fact, suicides or suicide attempts,” said Bobbi Jo Yarborough, PsyD, an investigator at the Kaiser Permanente Center for Health Research in Portland, Oregon.
“While healthcare settings are ideal places to intervene to prevent suicides, clinicians aren’t able to easily determine which of their patients are at elevated risk. Our ultimate goal is to develop the most accurate suicide risk prediction tools and put them into the hands of clinicians. If our study is successful, clinicians will have a powerful new resource in the fight against suicide.”
The research will help clinicians forecast the likelihood of a suicide attempt within 90 days of a mental health or primary care outpatient visit, strengthening suicide prevention.