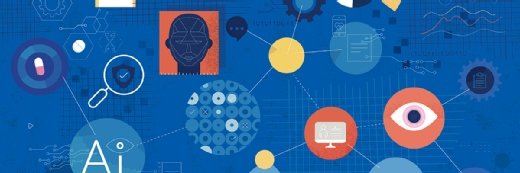
DrAfter123/DigitalVision Vectors
Deep Learning Can Identify Newborns at High Risk of Eye Disease
The FDA is currently reviewing the deep learning tool, which could help spot newborns at risk for a severe eye disease.
A deep learning device could help identify newborns at risk for aggressive posterior retinopathy of prematurity (AP-ROP), a condition that is difficult to diagnose and can lead to vision loss if left untreated.
Babies born prematurely are at risk for retinopathy, meaning they have fragile vessels in their eyes that can leak blood and grow abnormally. If untreated, vessel growth can get worse and cause scarring, leading to detachment of the retina and vision loss. The incidence of ROP each year in the US is about 0.17 percent, and most cases are mild and resolve without treatment.
When babies are born prematurely, providers screen and watch their eyes for signs of retinopathy. However, ROP-related changes occur along a spectrum of severity, and AP-ROP can elude diagnosis because its symptoms can be more subtle than those of typical ROP. While AP-ROP was recognized as a diagnostic entity in 2005, there is still significant variation among clinicians about whether eyes show signs of AP-ROP.
“Even the most highly experienced evaluators have been known to disagree about whether fundus images indicate AP-ROP,” said the study’s lead investigator, J. Peter Campbell, MD, MPH, Casey Eye Institute, Oregon Health and Science University in Portland.
Previous studies have shown that deep learning outperformed clinicians in detecting subtle patterns in fundus images and classifying ROP. Researchers at nine neonatal care centers used deep learning to determine how well these algorithms could detect AP-ROP.
The team followed 947 newborns for the study and had a deep learning model as well as a team of experts analyze fundus images from a total of 5,945 eye examinations. The results showed that among all newborns followed, three percent developed AP-ROP. Among the experts, there was a significant level of disagreement, indicating a need for objective metrics of disease severity.
Researchers were able to develop a clearer, quantifiable AP-ROP patient profile, which could help identify at-risk infants earlier. Newborns who developed AP-ROP tended to be more premature and born lighter than infants who needed treatment but never developed AP-ROP. In this population, no infants born after 26 weeks developed AP-ROP.
The team also found that AP-ROP tended to onset rapidly and quickly get worse. Providers have always implied rapid progression of disease when diagnosing AP-ROP, but to date there hasn’t been a way to measure this clinical feature. Researchers noted that monitoring the rate of vascular severity score changes could improve detection of AP-ROP risk.
“Artificial intelligence has the potential to help us recognize babies with AP-ROP earlier. But it also provides the foundation for quantitative metrics to help us better understand AP-ROP pathophysiology, which is key for improving how we manage it,” said Campbell.
The results also showed that infants with AP-ROP were more likely to have comorbidities such as chronic lung disease than infants without AP-ROP.
The deep learning system used in the study, called the i-ROP DL system, is currently under review by the FDA. The model was recently granted breakthrough status by the federal agency, which will help accelerate the development and potentially approval of the device.
The researchers noted that the technology could help improve diagnosis and management of AP-ROP, reducing the number of newborns who go blind from the condition each year.
“It’s important to acknowledge that there is currently no gold standard for diagnosing AP-ROP. But having objective, AI-based metrics for detecting AP-ROP is a step in the right direction for this highly vulnerable population of infants,” said Grace L. Shen, PhD, who manages the retinal diseases program for the Division of Extramural Science Programs at the NEI.
Artificial intelligence tools have played an increasingly big role in eye disease diagnosis and treatment. A research team recently developed an artificial intelligence tool that could help non-ophthalmologists accurately detect diabetic retinopathy in 60 seconds, enabling real-time screening for primary care providers and diabetes centers. The algorithm was able to accurately identify eye diseases in patients 95.5 percent of the time.
“Diabetic patients already outnumber practicing ophthalmologists in the United States, and unfortunately, that imbalance is only expected to grow,” said Srinivas Sadda, MD of the Doheny Eye Institute/UCLA and researcher on the diabetic retinopathy study.
“Accurate, real-time diagnosis holds great promise for the millions of patients living with diabetes. In addition to increased accessibility, a prompt diagnosis made possible with AI means identifying those at risk of blindness and getting them in front of an ophthalmologist for treatment before it is too late.”