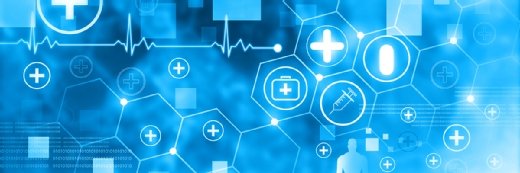
Rasi Bhadramani/istock via Getty
Artificial Intelligence Model Tracks Spread of Lyme Disease
The artificial intelligence tool could help states and counties develop more specific Lyme disease prevention strategies and improve public health.
Using 17 years of publicly available data, researchers built an artificial intelligence model that can help identify regions of the US where Lyme disease cases may go unreported, a study published in JAMA Network Open revealed.
Developed by researchers at Columbia University and RTI International, a nonprofit research organization, the model could allow states and counties to develop more comprehensive Lyme disease prevention and control plans.
“We believe our analysis can help predict the trajectory of where Lyme disease will spread,” said Maria Pilar Fernandez, a post-doctoral researcher at Columbia and lead author of the study.
“Identifying high-risk areas can lead to surveillance in counties and areas where infections are likely to emerge. It also allows authorities to alert physicians and the public, which can lead to early treatment, when it is most effective.”
If caught early, Lyme disease can be effectively treated with antibiotics, researchers said. However, longer-term infections often lead to more serious symptoms, including joint stiffness, brain inflammation, and nerve pain.
The team also noted that although about 300,000 cases of Lyme disease occur annually, just 30,000 cases are reported each year. Underreporting may limit the ability to identify areas of Lyme disease emergence, hindering early detection and effective treatment.
Lyme disease has been diagnosed in almost every state, with most reported cases occurring in the Northeast and upper Midwest. Developers have built models in the past to identify high-risk areas in certain states or regions of the US, but none have expanded the geographic scope to all areas of the country.
“Because no standardized nationwide tick surveillance system currently exists in the United States, there is a need to maximize information extraction from data reported by the CDC in an attempt to overcome potential biases and produce actionable public health information,” researchers said.
The team developed an artificial intelligence model that could expand the geographic scope of Lyme disease patterns. The model included all main endemic areas of the US as well as surrounding states and could help identify areas of the country where Lyme disease may go unreported.
Researchers trained the model on publicly available data that tracked the geographic spread of Lyme disease over nearly two decades. The group studied nearly 500,000 cases of the illness reported to the CDC from different counties between 2000 and 2017.
The model was able to predict the first reported Lyme disease case an average of 5.5 years earlier than it was reported to the CDC. Using the algorithm, researchers were also able to identify counties that could potentially have unreported cases of the disease.
“We were able to show that about 162 US counties may already have Lyme disease, but they have not yet been reported to the CDC,” said Maria Diuk-Wasser, associate professor in the Department of Ecology, Evolution and Environmental Biology at Columbia and a co-author on the study.
Among the 162 counties without reported cases, 47 had an 80 percent probability of reporting Lyme disease by 2018, the team noted. This finding offers an opportunity for public health officials to anticipate and explore areas where Lyme disease may be occurring or could emerge in the future.
As more cases are reported to the CDC, officials can continue to refine the model and direct active surveillance efforts to explore underreporting issues.
“Our predictive model, particularly if it is updated annually and expanded geographically, can enable states and counties to develop more specific Lyme disease prevention and control strategies, including optimizing active surveillance of human disease and ticks to increase early detection,” researchers concluded.
“Furthermore, this model will facilitate more targeted communication to and sensitization of the general population and the medical community to the risk of tick exposure and Lyme disease. Our findings can help public health authorities focus surveillance efforts in counties identified as being at high risk of Lyme disease emergence but where cases have yet to be reported.”
With this new algorithm, leaders can boost public health efforts and better plan for cases of Lyme disease.
“In the future, the model can be expanded,” Fernandez said. “We hope to continue to keep track of the spread and inform authorities about areas where Lyme disease is likely to emerge.”